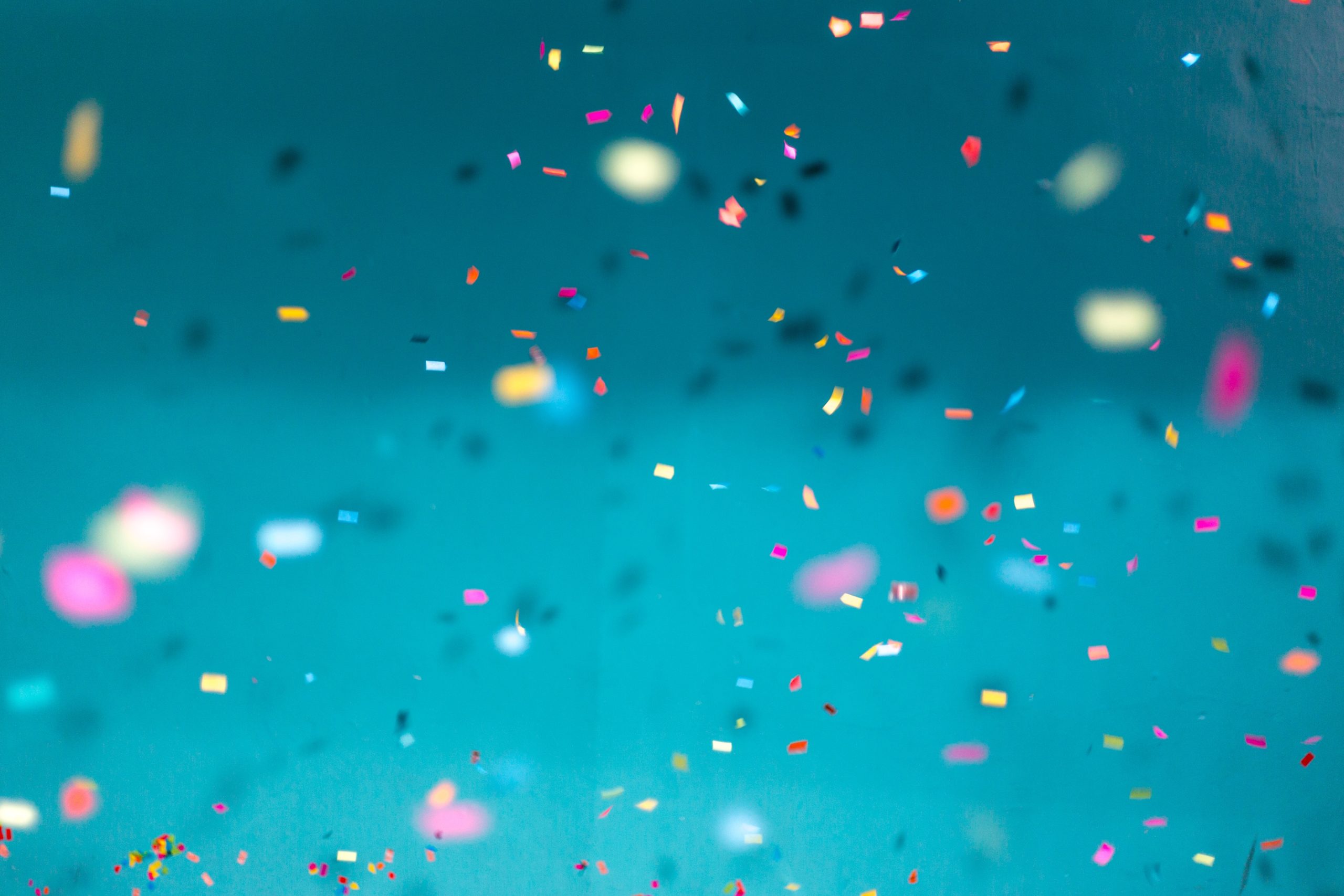
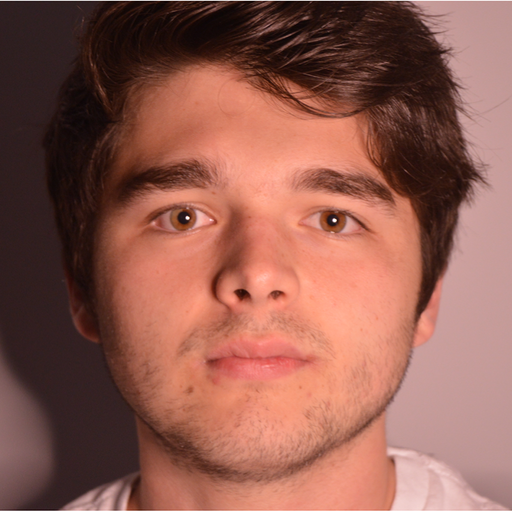
š¬ Research summary by Connor Wright, our Partnerships Manager.
[Original paper by Stephen Cave and Kanta Dihal]
Overview: Are white and non-white AI presented as equals? The authors argue not. While more diversity helps dissipate this effect, the problem cannot be solved by more diversity alone.
Introduction
Are white AIs and non-white AIs portrayed the same? As a commentary response to āMore than Skin Deepā by Shelley M. Park and a development of their 2020 paper āThe Whiteness of AIā, Cave and Dihal explore just that. To show this, Iāll get into the generalisability of white AI and touch upon Ex Machina as a key use case. Iāll then note the dilemma the authors point out, the solutions to this problem and how they can make a difference. Iāll then conclude with my view that acknowledging AI for what it is – the labour and extraction of materials alike – is where we will first start to see the signs of a difference being made.
Key Insights
Whiteness and Generality
White AI conforms to certain tendencies such as power and professionalism within the AI space, with the main attribute being its generalisability. This stems from the ideal that White people can be whomever they want: they are not vilified when they take on the role of a thug/thief, nor are they stereotyped in such positions. However, this luxury is not enjoyed by non-white people and, subsequently, non-white AI.
When assuming such roles, non-white people are stereotyped and confined to the role they find themselves in. Non-white people, when playing the role of a thief, are viewed as thieves instead of ānon-white people playing the role of a thiefā. This is a consequence of their non-generalisability; they cannot be whoever they want. Hence, given the non-universal nature of non-white people, they start to represent their whole race in their roles. To illustrate, the authors refer to the movie Ex Machina.
Ex MachinaĀ
In the movie, the main machine character, Ava, is played by a white character. āAva is portrayed as intelligent, eloquent, creative and powerfulāattributes the White racial frame associates with Whitenessā (p. 1777). This is opposed to another robot in engineer Nathanās house, Kyoko, an East Asian representation presented as submissive and less intelligent through her inability to speak. Furthermore, Jasmine, a black android, is also introduced, who does not have a head. Out of the three androids present, only the white edition is presented as fully human and capable of being whatever they choose. This is the whiteness frame which the authors mentioned previously in action, which offers its own dilemma.
The Diversity Dilemma
On the one hand, the dilemma involves how AI is presented in a white framework that reproduces harmful stereotypes of non-white AI (such as in Ex Machina). Yet, on the other hand, trying to solve this problem by clearly demarcating white and non-white AI could then play into the hands of racist ideas of servitude. AIās typical role is to obey the commands of a master human, which, if we establish white and non-white AI, would hark back to these ideas of a non-white entity serving a white person, even if this includes cases of a white AI doing the same. Placing white AI in the servant role may help white humans feel less guilty, but this doesnāt abstain them from their guilt as non-white AI are also still being exploited.
To tackle this problem, the authors observe three solutions.
The three solutions to the dilemma
- Sparrow (2020) proposes abandoning racialisation and anthropomorphism altogether. However, a study conducted by Liao and He (2020) showed how racialisation could be beneficial. It helps establish strong relationships between a human and an avatar of a similar skin tone. In terms of anthropomorphism, it proves extremely useful in establishing relationships of trust between humans and AI.
- A second solution could involve putting non-white AI in roles that break the stereotypical mould, such as Maeve in Westworld, disregarding the role assigned to her by white designers and leading an android revolution.
- A third comes in presenting non-white AI within powerful and intelligent positions to counteract any stereotypes further.
Nevertheless, the authors note how any long-lasting solution to the problem of whiteness requires a change in our perception of AI. We need to present AI in terms of the labour costs it involves, not just the genius ideas of Hollywood directors and Silicon Valley Billionaires.
Between the lines
I agree wholeheartedly with his change in perception. A brilliant resource for this is the Anatomy of an AI System undergone by Crawford and Joler. The whole lifecycle of an Amazon Alexa is presented in clear view, noting all the points of extraction and exploitation involved. In this way, seeing an AI for what it involves and ālooking under the hoodā (as advocated by Dr Maya Indira Ganesh in this podcast) will help address the issues that whiteness brings. Without doing so, we reproduce the same exploitation, just offshore.
Further resources
Liao, Y., He, J., 2020. The racial mirroring effects on human-agent in psychotherapeutic conversation. In Proceedings of the 25th International Conference on Intelligent User Interfaces, IUIā20. ACM, New York, pp. 430ā442. https://doi.org/10.1145/1234567890 Sparrow, R. (2020). Robotics has a race problem. Science, Technology, & Human Values, 45, 538ā560. https://doi.org/10.1177/0162243919862862