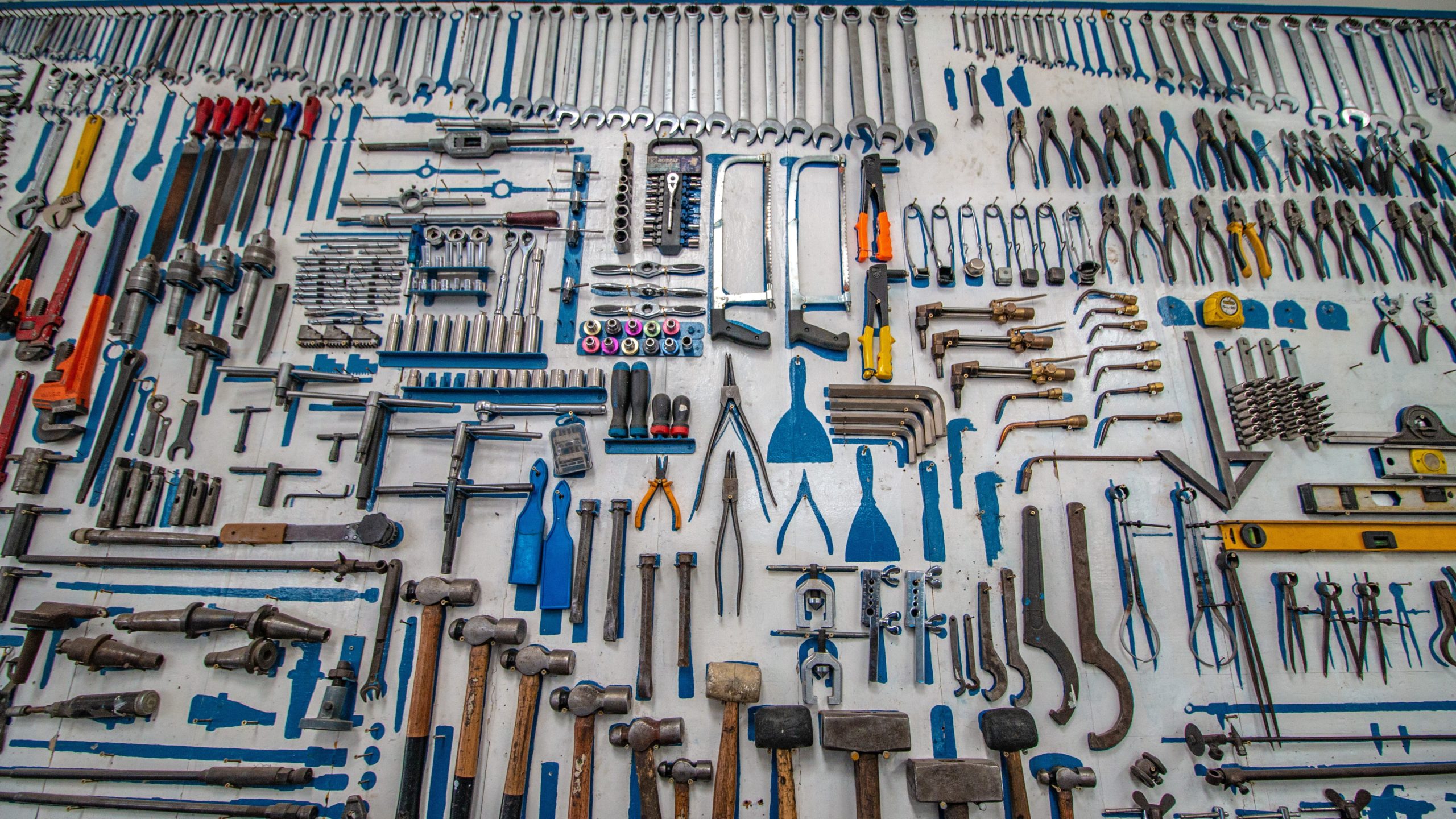
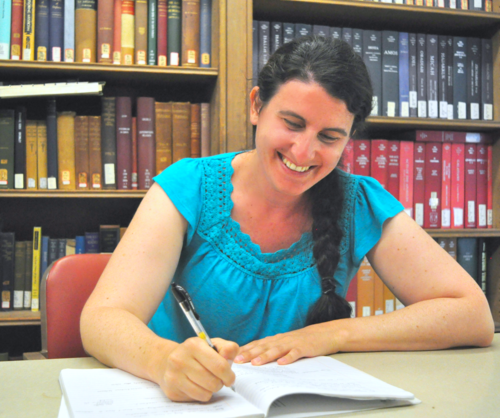
🔬 Research summary by Ravit Dotan, who mainly works in epistemology, philosophy of science, and philosophy of machine learning. Her secondary areas of interest are feminist philosophy and philosophy of religion.
[Original paper by Jacqui Ayling and Adriane Chapman]
Overview: This paper maps the landscape of AI ethics tools: It develops a typology to classify AI ethics tools and analyzes the existing ones. In addition, the paper identifies two gaps. First, key stakeholders, including members of marginalized communities, under-participate in using AI ethics tools and their outputs. Second, there is a lack of tools for external auditing in AI ethics, which is a barrier to the accountability and trustworthiness of organizations that develop AI systems.
Introduction
As more and more AI ethics tools are developed, it becomes difficult to get a handle on the terrain. This paper addresses the challenge by mapping and analyzing the existing AI ethics tools (as of the end of 2020).
The authors conducted a thorough search and identified 169 AI ethics documents. Of those, 39 were found to include concrete AI ethics tools. Each of the 39 tools was classified using the following questions: (i) What sector are the document’s authors from? And what sector are the users of the tools from? (ii) Which stakeholders would either use the tool or engage with the results? (iii) What type of tool is it? Which strategy does it employ? (iv) Were these tools for use internally, or did they have external elements? (v) In which stage in the AI production and use chain was the tool used? (vi) Was the tool appropriate for addressing the model, data, or both?
The paper presents statistics characterizing the tools using these questions. Among its findings, the paper uncovers that a wide stakeholder base, involving customers, the broader public, and the environment, is typically not a part of AI ethics evaluation processes. Moreover, the paper finds that almost all AI ethics tools are used internally, without external oversight. The authors emphasize that these characteristics stand in the way of accountability and trustworthiness of organizations that develop AI systems.
Key Insights
The map of AI ethics tool landscape
The paper divides AI ethics tools into three categories:
- Impact assessment tools
Impact assessment is a fact-finding and evaluation process that precedes or accompanies the production of artifacts, systems, or research. Ex-ante assessments are used in the use case development and testing stages. Ex post assessments are used post-deployment, in the monitoring stage, to capture the impacts of the system. The most predominant tools for impact assessments in AI ethics are checklists and questionnaires.
- Technical and design tools
These tools are typically developed by the ML community. Some of them are computational, e.g., computationally identifying and mitigating bias. Others are design processes, e.g., workshop-style events for raising awareness in design teams or participatory design processes. These tools are used along the whole process and can facilitate impact assessment and auditing.
- Auditing tools
An audit is an examination of evidence of a process or activity against some standards or metrics. To ensure transparency and to be able to place liabilities, the auditing process needs to be independent of the assessment process and from the day-to-day management of the auditee. AI ethics auditing tools are used in the late stages of the production process, when testing and monitoring the AI system. The focus of these tools is on appropriate documentation for verification and assurance. Checklists are also used for auditing, but less so.
Some statistics: The paper finds that tools for AI ethics are developed mostly by the private and academic sectors. However, the private and the public sectors are the ones that mostly use the tools. The paper also finds that more tools are developed for the early stages of the production process, namely the use case and design stages. Overall, AI ethics tools focus more on addressing models, as opposed to addressing data.
A gap in stakeholder participation
Typically, AI ethics tools are directly used by those who develop the AI system (e.g., development, delivery, quality assurance). The outputs of the AI ethics tools are typically used by decision-makers, such as elected officials and board members. There is typically little participation in the assessment and audit processes by traditionally marginalized groups, the users of the developed services, and vested interest stakeholders such as citizens, shareholders, and investors.
The paper emphasizes the relation between participation in AI ethics processes and power dynamics. The two are linked because participation has to do with who has the power to make decisions, who is invited to the table, and whose views and goals are prioritized. The paper recommends integrating a wider stakeholder base in AI ethics assessments and audits. It also recommends focusing the conversation on power relations rather than strictly on participation. Focusing on participation alone runs the risk of giving rise to “participation washing.”
A gap in auditing
Nearly all the AI ethics tools are for internal self-assessment only. There are generally no requirements or processes for publishing the outputs externally. The authors emphasize that external oversight is required for the trustworthiness of organizations developing the systems. Without robust oversight, there is a risk that organizations that develop AI systems would fall into a “checklist mentality” and would settle for performative gestures that constitute “ethics washing.”
Between the lines
This paper gives us language to talk about the different AI ethics tools that are out there. In doing so, it helps in understanding the complex landscape of AI ethics. The identification of the participation and auditing gaps invites the reader to seek solutions.
One topic for further exploration is which strategies are appropriate for external oversight in the case of AI ethics. It might be tempting to think of auditing processes familiar from the financial and other sectors. However, in the case of AI ethics, the participation from a wider stakeholder base in external oversight seems especially important given that ethical evaluations involve the values and the perspectives available to the evaluator. Can sufficient participation be introduced into familiar auditing processes, and if so, how? Alternatively, would it be better to design different oversight procedures for AI ethics? If so, what should they look like?