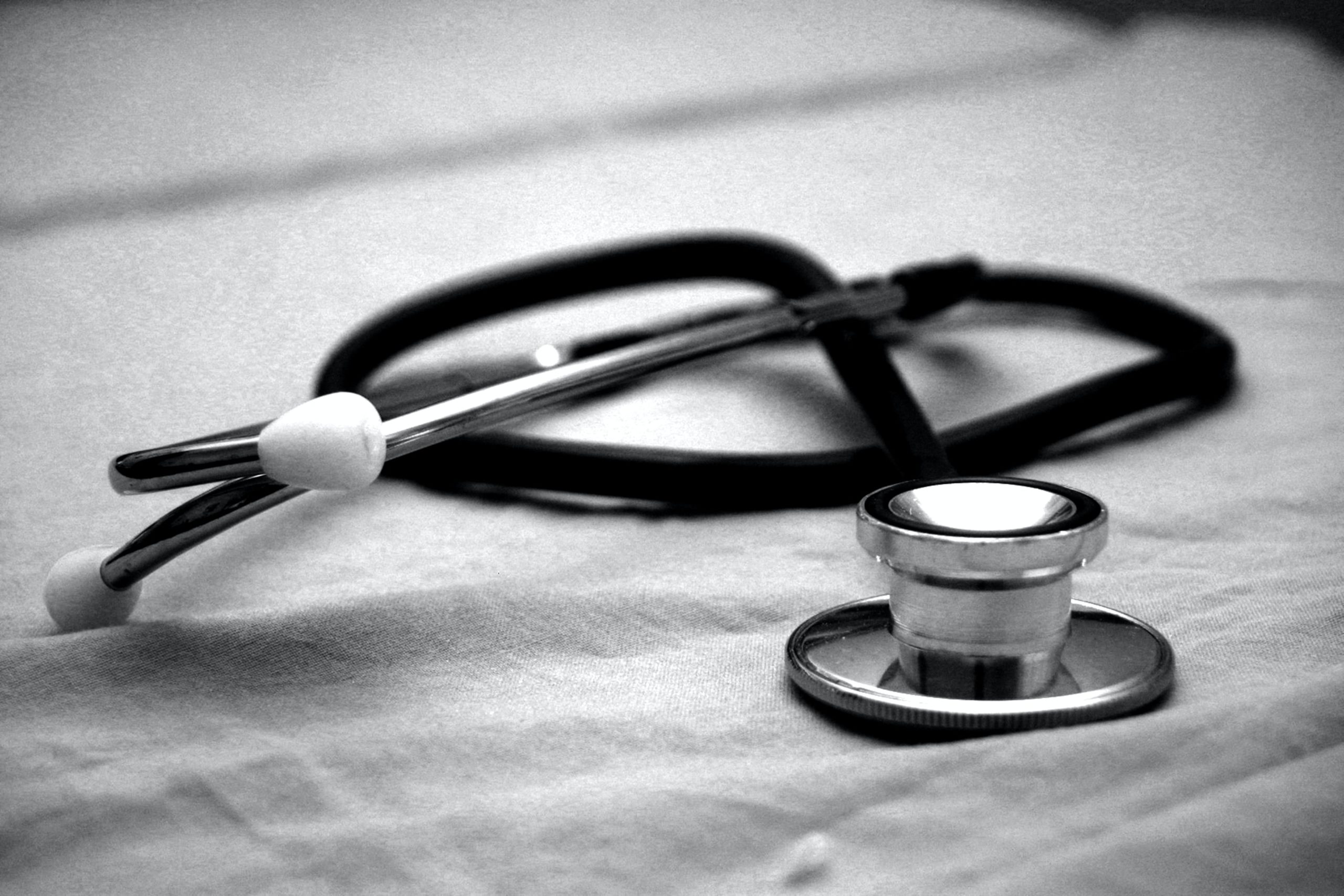
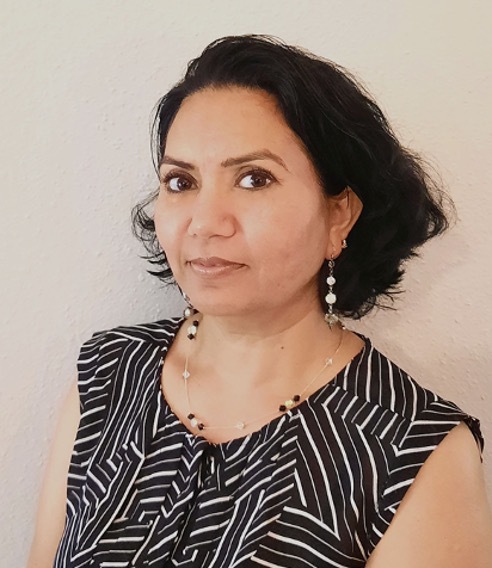
š¬ Research Summary by Shalini Saini, a doctoral researcher exploring privacy and security of AI in Medicine, Voice Biometrics, and Mobile Apps. She is working with Dr. Nitesh Saxena, Professor Of Computer Science at Texas A&M in the Department of Computer Science and Engineering and the SPIES ā Security and Privacy In Emerging computing and networking Systems research group.
[Original paper by Shalini Saini and Nitesh Saxena]
Overview: The security, integrity, and credibility of Medical AI (MedAI) tools are paramount issues because of dependent patient care decisions. MedAI solutions are often heavily dependent on scientific medical research literature as a primary data source that draws the attackerās attention as a potential target. We present a first study of identifying the existing predatory publication presence in the MedAI inputs and demonstrating how predatory science can jeopardize the credibility of MedAI solutions, making their real-life deployment questionable.Ā
Introduction
Technological advancements help medical research for groundbreaking achievements like the first human-independent AI system to detect Diabetic Retinopathy. The NIH invests more than $30 billion a year towards medical research, and NIH-NLM maintains a PubMed research repository comprising more than 32 million citations for biomedical literature. Many MedAI systems rely upon scientific research publications as the primary data source for knowledge extraction. Especially in the case of rare diseases, a single publication can be of great interest, but what if it comes from a questionable source? If research gets manipulated through bogus, plagiarized, biased, or fraudulent conclusions, it becomes predatory research, potentially harming patients directly or indirectly.
MedAI solutions utilize research literature as primary data input and are prone to data pollution. Passive data pollution attacks and active adversarial attacks can impact the integrity and security of MedAI solutions. Untargeted predatory publications induce passive data pollution, while an active adversarial attack is a targeted approach that deliberately poisons the publication databases through specific predatory journals.
Our research demonstrates that the existing and presumably untargeted data pollution can potentially influence the output of MedAI. Our work casts serious doubt on whether research literature-based MedAI solutions are reliable enough to be part of practical healthcare services.
Key Insights
Health Care Revolution through MedAI
Around one in 10 Americans is affected by some rare disease, and 80% of around 7000 known rare diseases are genetics-based. Non-availability of the diagnosis and wrong diagnosis can delay much-needed medical assistance. There is an ongoing effort to have a more extensive set of inputs on genomics, drugs, diseases, and documenting patientsā history. Medical AI (MedAI) is expected to utilize this plethora of existing knowledge to answer many unanswered questions to provide accessible, affordable, and improved healthcare services. A 2021 data shows that 230 startups are using AI in drug discovery. There is compelling evidence that MedAI can play a vital role in enhancing and complementing future cliniciansā medical intelligence.
Security and Integrity of MedAI
MedAI aims to combine all relevant data and filter out irrelevant information without skipping more challenging instances. Modern healthcare solutions using AI look promising to save time, money, and effort significantly, but the cost of ātrusted but manipulatedā information from such MedAI solutions is too high to ignore. An unreliable and potentially flawed MedAI output can misalign the overall cycle of future research and healthcare solutions in a harmful direction. It is a known issue that adversarial attacks on neural networks can cause erroneous results damaging confidence in MedAI. The attacker can potentially perturb it in a direction that aligns well with the weights of the MedAI algorithm and thus amplifies its effect on the output. It is crucial for MedAI, especially in rare diseases, to include that one paper with the latest finding that may alter the life of some patient(s). It is even more critical to validate whether that paper is predatory.
A real challenge is maintaining the integrity and security of research data inputs of MedAI in Precision Medicine and not letting it be Predatory Medicine, especially in finely targeted scenarios.
Predatory Research: Threat to Data Integrity and Credibility of MedAI Solutions
Medical research has been revolutionary in the past few years, but innovation is not the only reason. āPublish or Perishā culture puts enormous pressure on researchers to publish their work. Publications and citations are a standard metric for progressing towards the doctorate, employment, promotions, and grants/ funding by state and federal agencies. Opportunists may exploit these trends for their benefit to lure easy targets looking for some publication credits. Research misconduct is an even more significant threat as misleading conclusions may go undetected for an extended period and affect clinical practices. With the possibility of having plagiarized, incorrect, unverified, fake data and manipulated results, predatory journals are, in fact, increasingly interfering with genuine research.
From a few identified by Beall in 2011, by 2015, there were estimated as many as 10,000 predatory journals worldwide. The ultimate risk is the altered results of synthesized research because of the rapidly increasing numbers of such predatory publications. Efforts have exposed such practices of accepting fake papers and recruiting fake editors. However, numbers are still rising each year and making their presence in trusted research repositories like PubMed. A genuine concern is that Cabellās list has more suspected predatory journals than legitimate ones. Accommodating information from heterogeneous sources is vital in decision-making. However, it also enables predatory research to get mixed with authentic inputs.
Predatory Research in Research Literature-Dependent Medical AI Systems
Evaluating the extent of existing passive data pollution induced by potential predatory publications is vital. We have two broad objectives; the first is to measure the presence of potential predatory publications in the input data sources of medical AI solutions, and the second is to demonstrate the impact of the polluted dataset on the outcome of the MedAI solutions. We studied two real-life medical AI solutions covering diverse input formats, AI methods, and target populations for a more generalized conclusion.
Conclusion and Implications
Our results show a growing presence of predatory research in PubMed and, eventually, how it is navigating through trusted intermediate datasets to the output of the MedAI. We observed that potential predatory research publications have a significant presence in PubMed, PubMed-derived trusted NIH research databases like SemMedDB and NIH Translational Knowledge Graphs. We could demonstrate the presence of predatory research in the output of studied real-life MedAI solutions. We verified that research literature-dependent medical AI solutions are prone to predatory data pollution and thus diminishing the credibility of such MedAI solutions.
Our study shows clear evidence of how predatory research may influence patient care decisions if used in practice without resolving the existing threat of predatory research intrusion. Implementing such medical AI solutions can be critical in clinical settings affecting patient care decisions directly, and thus cannot be taken lightly.
Between the lines
Precision Medicine and MedAI solutions are making their way into mainstream patient care. MedAI solutions may not meet the high expectations of delivering trustworthy solutions. It is paramount to address existing vulnerabilities and possible future adversarial attack threats beforehand. It is vital to identify issues earlier in the process to avoid failure later and re-engineer the approach. These concerns resonate with the known ethical and regulatory challenges with MedAI solutions involving privacy, data integrity, accessibility, accountability, transparency, and liability.
Without a standard definition for predatory journals and rigorous scrutiny, it is challenging to build a workable defense mechanism to filter out predatory research. The derived databases also need regular updates to keep the information current and valid, including removing or flagging the retracted research references. A viable adversarial attack scenario to produce targeted predatory publications in bulk through new-age NLP text-generator tools like GPT-3 may further damage the trust in research literature-based MedAI solutions.
The new age MedAI solutions need to be as comprehensive as possible, but with the open-access research publications and social media, the future trustworthy information extraction will have more significant challenges and higher stakes.