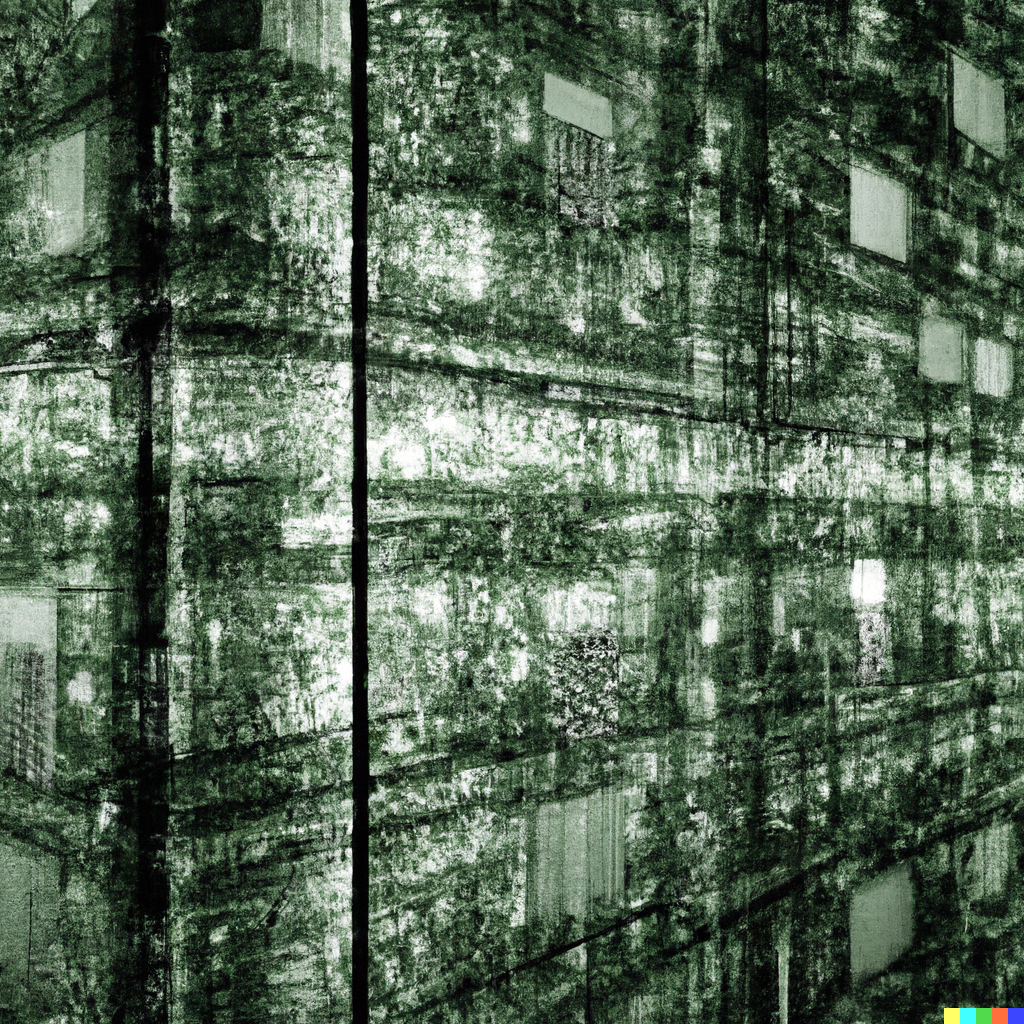
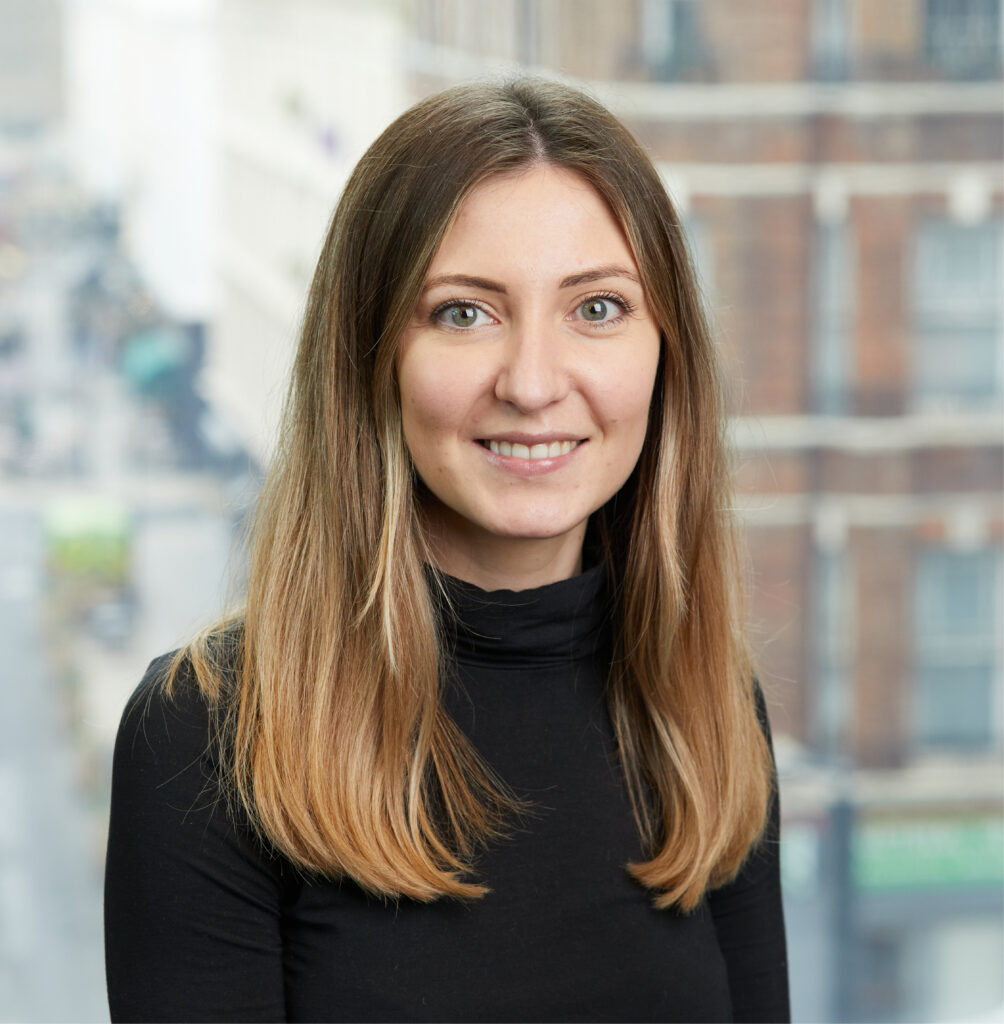
Research Summary by Lauren Arthur, Marketing Director at Hazy, a leading synthetic data company.
[Original paper by Georgi Ganev, Jason Costello, Jonathan Hardy, Will O’Brien, James Rea, Gareth Rees, and Lauren Arthur]
Overview: Generative AI technologies, such as synthetic data, are gaining significant popularity, especially in large enterprises. This paper focuses on the challenges of deploying synthetic data in enterprise settings and the need for a structured approach to address these challenges effectively and establish trust in implementing synthetic data solutions.
Introduction
Generative AI has surged to the forefront of mainstream media in recent years, largely thanks to OpenAI’s open-source tools, which democratize access to powerful technology. While individuals are harnessing these tools for increased efficiency and speed in everyday tasks, businesses have the potential to use them to drastically scale up operations, thereby enhancing business growth.
Within the generative AI realm, synthetic data is a sub-category that has existed for some time. It empowers businesses to use data quickly and easily, unburdened by the constraints of outdated infrastructure and privacy regulations. However, implementing this still relatively nascent technology within large, complex organizations—enterprises—presents challenges.
In this paper, the authors delve into 40 distinct challenges—spanning technical and business domains—that enterprises face when they deploy and use synthetic data. The authors advocate for integrating synthetic data into an organization’s strategic objectives and propose a methodical approach, divided into three phases, to assist professionals in successfully deploying this privacy-enhancing technology (PET) for success/to drive impact.
Key Insights
Synthetic data: an advanced PET within Gen AI
Generative AI refers to a class of artificial intelligence techniques and models that have the ability to generate new data samples that are similar to existing data. These models can generate various types of content, including text, images, audio, and more. They’ve gained popularity for their creative and generative capabilities.
Synthetic data is a subcategory of generative AI – data artificially created by generative models. It can be used to mimic real-world data, allowing organizations to create large datasets without exposing sensitive or private information. It’s particularly useful when working with data with privacy concerns, like medical records or financial transactions.
Whereas newer large language model variations of generative AI (ChatGPT, for example) have only gained mass media and consumption in the last year, synthetic data has been used in organizations, specifically enterprises, for the last five years. Common use cases for PETs include software and database testing, AI model training, internal and external sharing, and monetization of data and insights. It is a powerful tool for enterprises to access and use their data and drastically speed up operations. That said, to deploy it within an enterprise is no small feat. For it to have a wide impact, there are numerous stages to work through – both from a technical and business standpoint – which the paper delves into.
An overview of the challenge areas
The paper looks at 40 specific challenges, grouped into five sections – data generation, infrastructure & architecture, governance, compliance & regulation, and adoption. Whilst not exhaustive, this list was drawn from first-hand experience deploying this technology within enterprises.
It’s important to emphasize that these five areas cannot be assessed or addressed in isolation; they hold equal importance in ensuring the successful deployment and sustained effectiveness of synthetic data within an enterprise.
Privacy is important in the paper and in general when discussing synthetic data, primarily because an enterprise’s reputation is paramount for success. Mishandling or breaching personal customer data can severely damage its standing. From a technical standpoint, privacy in the context of synthetic data adds an extra layer of complexity. The paper looks at the application of differential privacy to synthetic data, offering a robust mathematical safeguard. However, there are specific parameters to decide about to ensure that the intended level of protection is effectively achieved.
A practical approach
Whilst the paper focuses on the challenges of deploying synthetic data, it remains certain that synthetic data is a viable and extremely beneficial technology for enterprises to deploy. It speeds up operations across various functions, including IT, analytics, marketing, and operations, all whilst protecting customer privacy and complying with regulations.
In addition to addressing individual-level challenges, the authors propose a three-stage approach to deploying the technology while effectively mitigating these challenges. The initial phase of this approach, common to many transformation projects that include nascent technology, emphasizes the “starting small” approach, which includes educating stakeholders about synthetic data and demonstrating its practical application quickly to secure buy-in and support for future scaling.
In the second phase, known as “scaling,” the primary focus revolves around broadening the scope of use cases and increasing adoption across the organization. This phase encompasses technical aspects, such as architectural adjustments and cultural and governance considerations.
The third and final phase, termed the “future” phase, envisions the integration of synthetic data as a fundamental component of an enterprise’s data strategy. This integration can be achieved through models like a data marketplace or the use of on-demand synthetic data, effectively reducing reliance on real data for greater speed of operations and protection of customer privacy.
Conclusion
Synthetic data has proven to be a reliable solution in commercial environments, offering tangible benefits such as efficiency improvements, innovation acceleration, and reduced compliance risk.
This paper examines the myriad challenges of deploying synthetic data in large-scale enterprise settings. The categorization of challenges and proposed systematic approach should act as a starting point for practitioners and professionals interested in adopting synthetic data solutions. Navigating these challenges effectively will unlock the full potential of synthetic data and contribute to building trust in its implementation within enterprises.
Between the lines
In today’s data-centric world, the importance of data and privacy spans individual and corporate agendas and is only growing. As a result, privacy-enhancing sub-sections of generative AI, such as synthetic data, are maturing and increasingly being used by more organizations. Yet, as with any maturing technology, these advancements are not without challenges.
While there is a vast body of research on the theory of generative AI, its practical use in large, complex businesses is limited. To make a substantial and lasting impact, businesses must trust this technology before wide-scale adoption, but building this trust is not straightforward; it is nuanced and demands resources, budget, time, and regulation, as well as buy-in, a level of expertise, and enthusiasm.
This paper explores the specific challenges faced when deploying synthetic data and offers a starting framework for addressing them. There could be a much deeper exploration of all the challenges – particularly the technical implications of bias, data hallucinations, and privacy. As the domain evolves, additional research will be essential to refine and expand this groundwork.