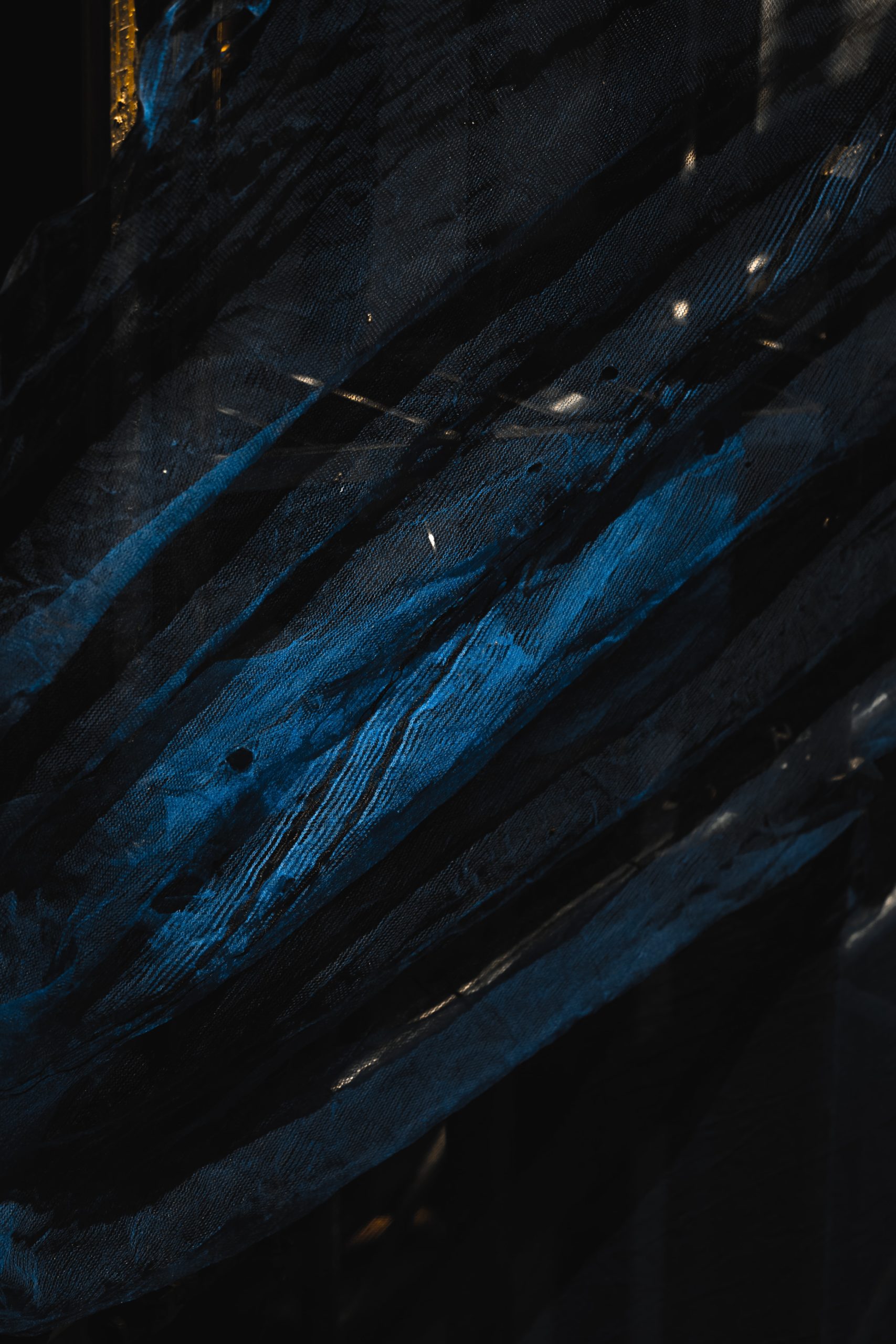
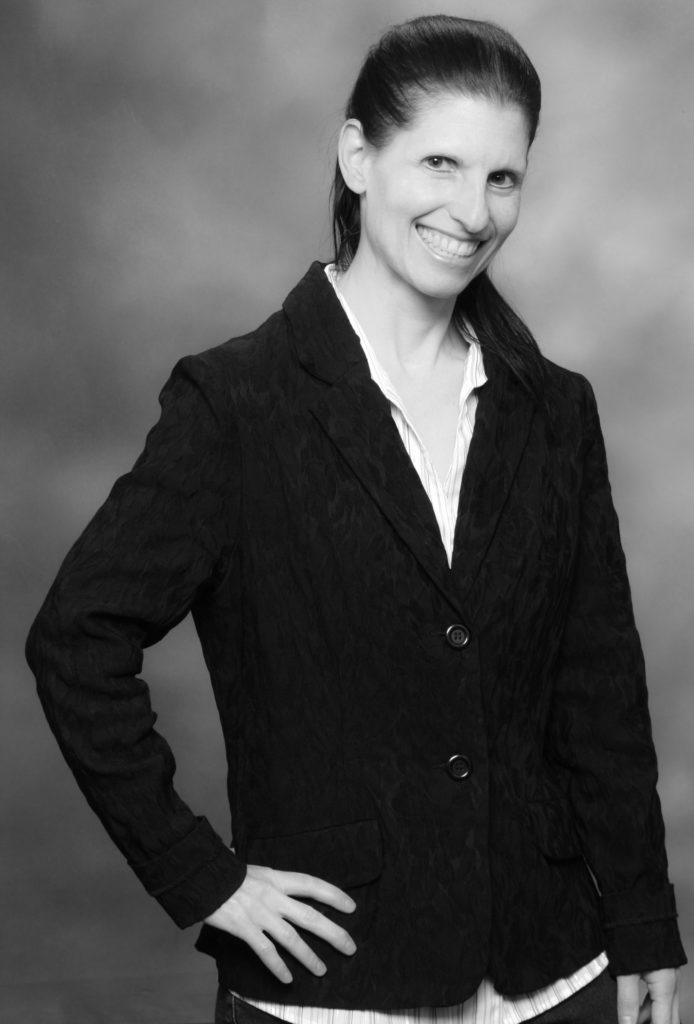
🔬 Research Summary by Christina Catenacci, BA, LLB, LLM, PhD, works at the intersection of electronic surveillance technologies, privacy, cybersecurity, and data ethics.
[Original paper by National Institute of Standards and Technology (NIST) contributors: Reva Schwartz, Apostol Vassilev, Kristen Greene, Lori Perine, Andrew Burt, Patrick Hall]
Overview: While there may be several benefits associated with Artificial Intelligence (AI), there are some biases associated with AI that can lead to harmful impacts, regardless of intent. Why does this matter? Harmful outcomes create challenges for cultivating trust in AI. Current attempts to address harmful effects of AI bias remain focused on computational factors, but the currently overlooked systemic and human, as well as societal factors are also significant sources of AI bias. We need to take all forms of bias into account when creating trust in AI.
Introduction
But how can we take all forms of AI bias into account? We can expand our perspective and take a socio-technical approach when examining and addressing AI bias. By socio-technical, I mean an approach used to describe how humans interact with technology within the broader societal context. In fact, the authors point out that, while there are many practices that aim to responsibly produce AI, guidance from a broader socio-technical perspective is needed in order to manage the risks of AI bias, operationalize values, and create new norms around how AI is built and deployed.
In this report, the authors aim to provide a first step for developing detailed socio-technical guidance to identify and manage AI bias. In this special publication, the goal is to describe the challenges of bias in AI, identify and describe the three categories of bias in AI (computational, systemic, and human), and discuss the three broad challenges for mitigating bias. Ultimately, the authors provide preliminary guidance, and indicate that NIST intends on continuing this work and creating further guidance and standards in the future.
Key Insights
Characterizing AI bias
The authors identify the following categories of AI bias:
- Statistical and computational. These biases stem from errors that result when the sample is not representative of the population. They arise from systematic error, and can occur in the absence of prejudice, partiality, or discriminatory intent. In AI systems, these biases are present in the datasets and algorithmic processes used in the development of AI applications, and often arise when algorithms are trained on one type of data and cannot extrapolate beyond that data
- Systemic. These biases result from procedures and practices of particular institutions that operate in ways which result in certain social groups being advantaged or favoured and others being disadvantaged or devalued. This may not be the result of any conscious prejudice or discrimination, but rather of the majority following existing rules or norms. It occurs when infrastructures for daily living are not developed using universal design principles, thereby limiting or hindering accessibility for persons belonging to certain groups
- Human. These biases reflect systematic errors in human thought based on a limited number of heuristic principles (mental shortcuts) to simplify decision-making. These biases are often implicit and tend to relate to how an individual or group perceives information when they make decisions or complete missing or unknown information. These biases exist across the AI lifecycle. There is a wide variety of cognitive and perceptual biases that appear in all domains.
It is also worth noting that page 8 of the report provides a useful diagram of the three main types if biases in AI, with plenty of examples.
How might the above biases be harmful? Applications that use AI are often deployed across sectors and contexts for decision-support and decision-making—without involving humans. And it is not clear whether they are capable of learning and operating in accordance with our societal values. Moreover, according to the authors:
These biases can negatively impact individuals and society by amplifying and reinforcing discrimination at a speed and scale far beyond the traditional discriminatory practices that can result from implicit human
or institutional biases such as racism, sexism, ageism or ableism.
This is why it is so important to begin utilizing a socio-technical systems approach when examining AI. In this way, we may evaluate dynamic systems of bias and understand how they impact each other. We can better understand the conditions under which these biases are attenuated or amplified. It involves a reframing of AI-related factors, and taking into account the needs of individuals, groups, and society.
Challenges and guidance
The authors set out several challenges and propose guidance relating to these challenges:
- Dataset factors such as availability, representativeness, and baked-in societal biases
- Issues of measurement and metrics to support testing and evaluation, validation, and verification (TEVV)
- Human factors, including societal and historic biases within individuals and organizations, as well as challenges related to implementing human-in-the-loop
Following this, the authors provide guidance concerning governance—why is this important? Governance processes impact nearly every aspect of managing AI bias. Looking at things holistically, the authors delve into various elements such as organizational measures and culture:
- Monitoring. It is important to determining whether performance is different than expected once deployed
- Recourse channels. It is necessary to have feedback channels to allow system end users to flag incorrect or potentially harmful results, and seek recourse for errors or harms
- Policies and procedures. It is important ensure that written policies and procedures address key roles, responsibilities, and processes at all stages of the AI model lifecycle to manage and detect potential overall issues of AI system performance
- Documentation. Clear documentation practices can help to systematically implement policies and procedures, standardizing how an organization’s bias management processes are implemented and recorded at each stage. It also helps to ensure accountability
- Accountability. It is critical that individuals or teams bear responsibility for risks and associated harms to ensure that there is a clear assessment of the role of the AI system itself, and provide a direct incentive for the mitigation of risks and harms
- Culture and practice. AI governance must to be embedded throughout the culture of an organization in order to be effective
- Risk mitigation, risk tiering, and incentive structures. There needs to be an acknowledgement that risk mitigation, not risk avoidance, is often the most effective factor in managing such risks
Information sharing. Sharing cyber threat information helps organizations improve their cybersecurity postures, and those of other organizations
Between the lines
This report takes a broad overview of the complex challenge of addressing and managing risks associated with AI bias. In order to promote the trustworthiness of AI, the authors approach AI as a socio-technical system, acknowledging that AI systems and the associated biases extend beyond the computational level. In my view, the document rightly acknowledges that there are some factors that are contextual in nature, and they must be examined from different angles using a fresh approach.
I think that this report constitutes a useful first step in the process. In terms of next steps, it would be highly beneficial for NIST to develop further socio-technical guidance in collaboration with interdisciplinary researchers. The authors create a helpful Glossary that explains the main terms used throughout the report—a valuable tool for future discussions.