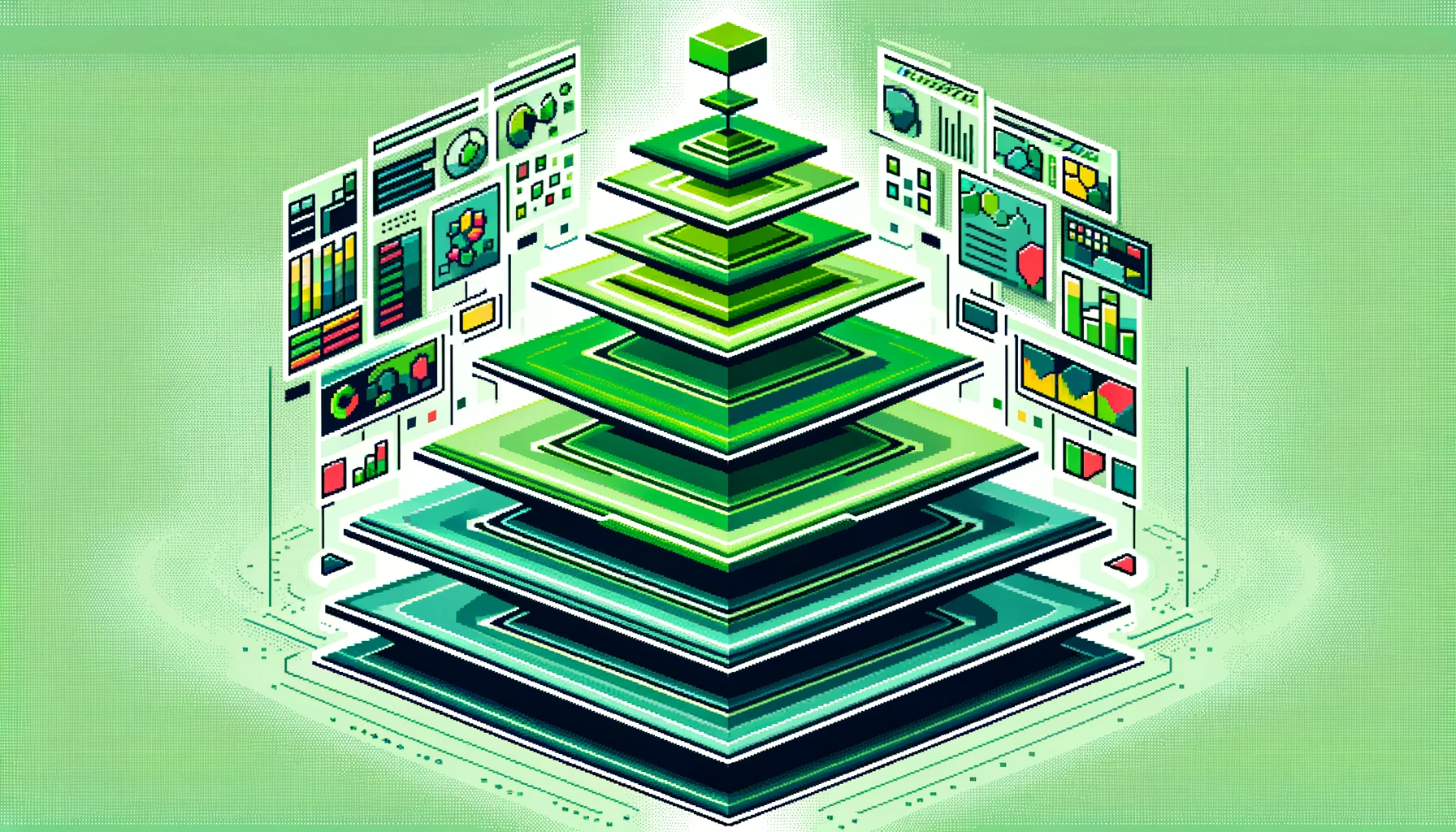
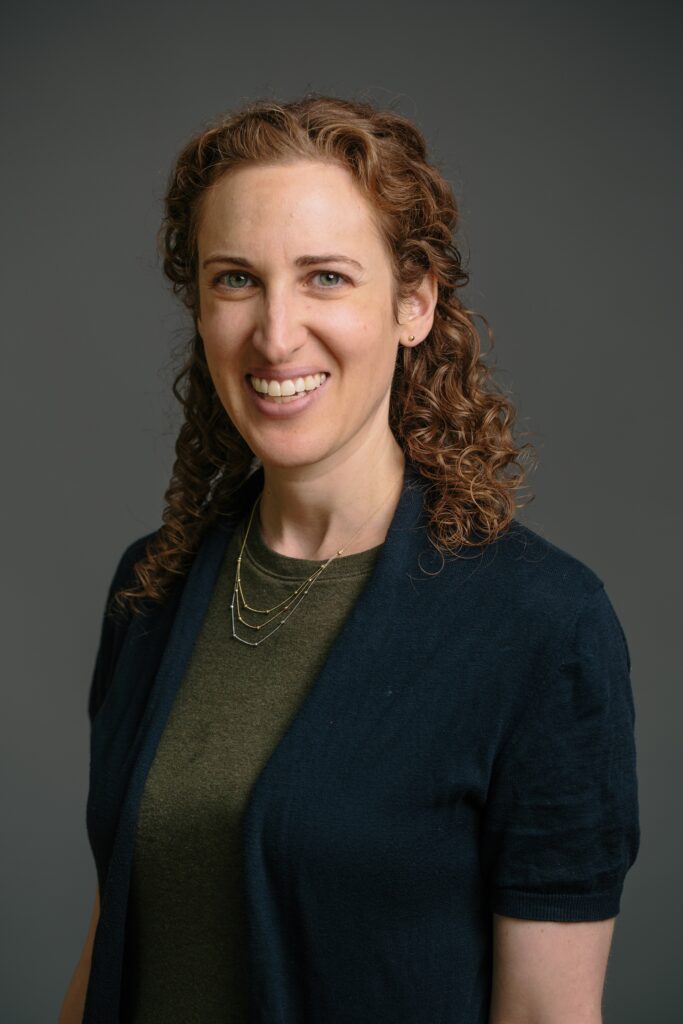
🔬 Research Summary by Meredith Ringel Morris, Director of Human-AI Interaction Research at Google DeepMind; she is also an Affiliate Professor at the University of Washington, and is an ACM Fellow and member of the ACM SIGCHI Academy.
[Original paper by Meredith Ringel Morris, Jascha Sohl-dickstein, Noah Fiedel, Tris Warkentin, Allan Dafoe, Aleksandra Faust, Clement Farabet, and Shane Legg (all affiliated with Google DeepMind)]
Overview: This paper proposes a theoretical framework for classifying the capabilities and behavior of AGI (Artificial General Intelligence) systems. The proposed ontology introduces a level-based classification of AGI performance, generality, and autonomy. The paper includes discussions of how this framing relates to prior conceptualizations of AGI, how more nuanced terminology around AGI systems can support discussions of risk and policy options, and the need for future work in developing ecologically valid, living benchmarks to assess systems against this framework.
Introduction
Artificial General Intelligence (AGI) is an important and sometimes controversial concept in computing research, used to describe an AI system that is at least as capable as a human at most tasks. Given the rapid advancement of Machine Learning (ML) models, the concept of AGI has passed from being the subject of philosophical debate to one with near-term practical relevance. Some experts believe that “sparks” of AGI (Bubeck et al., 2023) are already present in the latest generation of large language models (LLMs); some predict AI will broadly outperform humans within about a decade (Bengio et al., 2023); some even assert that current LLMs are AGIs (Agüera y Arcas and Norvig, 2023). However, if you were to ask 100 AI experts to define what they mean by “AGI,” you would likely get 100 related but different definitions. We argue that it is critical for the AI research community to explicitly reflect on what we mean by “AGI” and aspire to quantify attributes like the performance, generality, and autonomy of AI systems. Shared operationalizable definitions for these concepts will support comparisons between models, risk assessments, and mitigation strategies; clear criteria from policymakers and regulators; identifying goals, predictions, and risks for research and development; and the ability to understand and communicate where we are along the path to AGI.
Key Insights
The paper presents nine case studies of prior prominent formulations of the concept of AGI and analyzes these to develop six principles for a clear, operationalizable definition of AGI. These six principles are:
- Focus on Capabilities, not Processes
- Focus on Generality and Performance
- Focus on Cognitive and Metacognitive Tasks
- Focus on Potential, not Deployment
- Focus on Ecological Validity
- Focus on the Path to AGI, not a Single Endpoint
These six principles are used to formulate a matrixed ontology, the “Levels of AGI,” considering a combination of performance and generality. The six levels of Performance are:
0. No AI
1. Emerging (equal to or somewhat better than an unskilled human)
2. Competent (at least 50th percentile of skilled adults)
3. Expert (at least 90th percentile of skilled adults)
4. Virtuoso (at least 99th percentile of skilled adults)
5. Superhuman (outperforms 100% of humans)
Table 1 in the paper attempts to classify classic and SOTA AI systems according to their level of performance and generality (narrow vs. general) and discusses the need to create an ecologically valid, living benchmark to determine the performance of novel systems more precisely. The paper theorizes that today’s generative language models would be considered “Emerging AGI.” However, they display Competent or even Expert level performance for some narrow tasks, highlighting the unevenness of capability development that is likely to be characteristic of AGI systems.
Table 2 in the paper introduces an autonomy dimension to the ontology, observing that the paradigm of human-AI interaction jointly with system capabilities determines risk. While higher Levels of AGI unlock novel human-AI interaction paradigms (up to and including fully autonomous operation), they do not determine them – the choice of appropriate interaction paradigm depends on many contextual considerations, including AI safety. The levels of Autonomy are:
0. No AI (human does everything)
1. AI as a Tool (human fully controls tasks and uses AI to automate mundane sub-tasks)
2. AI as a Consultant (AI takes on a substantive role, but only when invoked by a human)
3. AI as a Collaborator (co-equal human-AI collaboration; interactive coordination of goals & tasks)
4. AI as an Expert (AI drives interaction; human provides guidance & feedback or performs subtasks)
5. AI as an Agent (fully autonomous AI)
Between the lines
This paper introduces a nuanced framework for classifying AI systems’ performance, generality, and autonomy. Shared terminology and frameworks can help researchers, policymakers, and other stakeholders communicate more clearly about progress toward (and risks from) powerful AI systems. This paper also emphasizes the need for future research on developing an AGI benchmark (and discusses the challenges of doing so) and emphasizes the importance of investing in human-AI interaction research in tandem with model improvements in light of the insight that autonomy paradigms interact with model capabilities to determine a system’s risk profile.