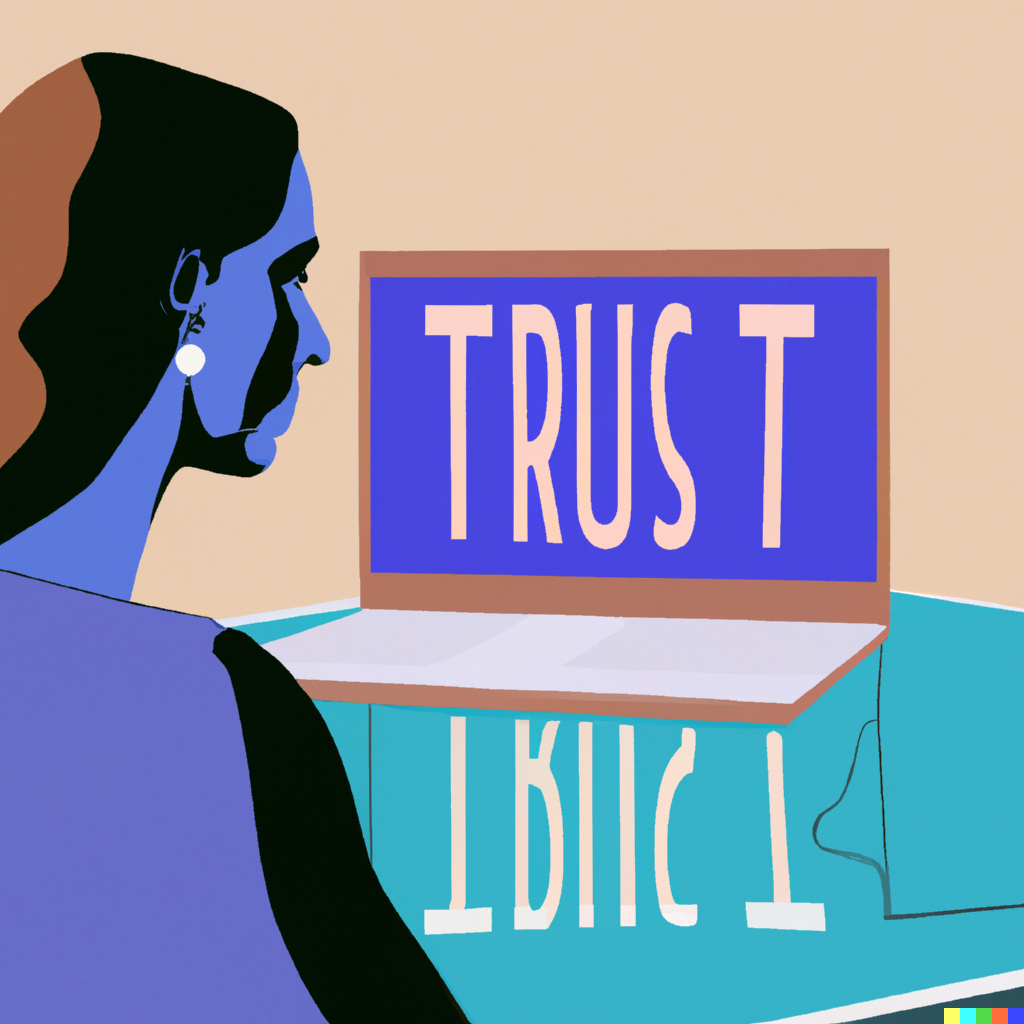
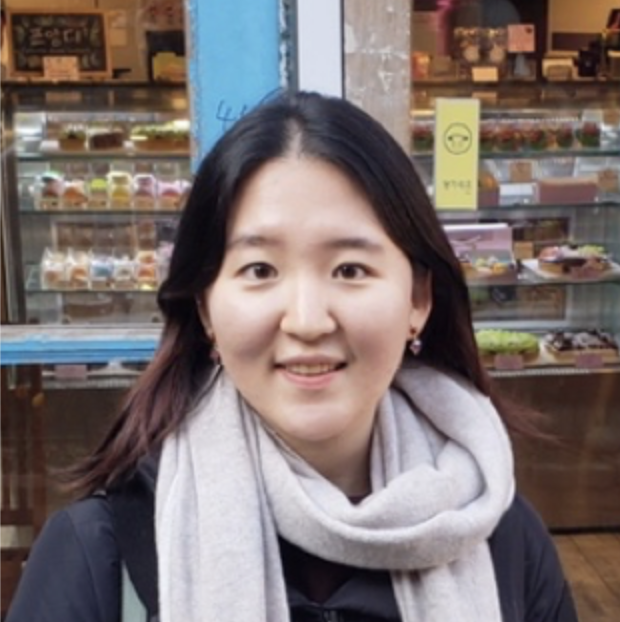
🔬 Research Summary by Sunnie S. Y. Kim, a PhD student in computer science at Princeton University working on AI transparency and explainability to help people better understand and interact with AI systems.
[Original paper by Sunnie S. Y. Kim, Elizabeth Anne Watkins, Olga Russakovsky, Ruth Fong, and Andrés Monroy-Hernández]
Overview: Trust is a key factor in human-AI interaction. This paper provides a holistic and nuanced understanding of trust in AI by describing multiple aspects of trust and what factors influenced each in a qualitative case study of a real-world AI application.
Introduction
Appropriate trust is crucial for safe and effective interactions with AI systems. However, there is a lack of empirical studies investigating what factors influence trust in AI and how in real-world contexts. Most research investigates how a certain factor affects trust in controlled lab settings. While they provide valuable insights into the relationship between trust and the factor of interest, their research design does not allow for capturing the contextual aspects of trust or discovering new trust-influencing factors. In this paper, we address these two gaps and deepen the understanding of trust in AI through a qualitative case study of a real-world AI application.
Concretely, we interviewed 20 end-users of a popular, AI-based app for bird identification. We inquired about their trust in the app from many angles, asking questions about their context of app use, perception and experience with it, and intention to use it in hypothetical, high-stakes scenarios. Afterward, we analyzed the collected data with the widely-accepted trust model of Mayer et al. In the next section, we describe participants’ trust in AI in three parts: (1) trustworthiness perception and trust attitude, (2) AI output acceptance, and (3) AI adoption.
Key insights
(1) Trustworthiness perception and trust attitude
Overall, participants assessed the app to be trustworthy and trusted it. We drew this conclusion based on participants’ responses regarding the app’s ability, integrity, and benevolence—the three factors of perceived trustworthiness in Mayer et al.’s trust model. Participants assessed that the app possesses all three, based on their positive prior experience with it, its popularity, and the domain’s and the developers’ good reputation.
(2) AI output acceptance
However, we observed a more complex picture of trust when we examined participants’ app output acceptance decisions. Participants did not accept the app’s outputs as true in every usage instance. To the extent possible, they carefully assessed the outputs, using their knowledge about the domain and engaging in verification behaviors. When unable to verify, some participants disregarded the outputs, even though they described the app as trustworthy.
(3) AI adoption
Finally, we examined participants’ AI adoption decision-making by asking whether they would use the app in hypothetical, high-stakes scenarios with health-related and financial outcomes. We found that while participants always used the app in their actual use setting, they made different decisions for the high-stakes scenarios based on various factors: the app’s ability, familiarity, and ease of use (AI-related factors); their ability to assess the app’s outputs and use the app (human-related factors); and finally, task difficulty, perceived risks and benefits of the situation, and other situational characteristics (context-related factors).
Trust in AI is multifaceted and influenced by many factors
In short, we found that end-users’ trust relationship with AI is complex. Overall, participants found the app trustworthy and trusted it. Still, they carefully assessed the correctness of individual outputs and decided against app adoption in certain high-stakes scenarios. This discrepancy illustrates that trust is a multifaceted construct that must be approached holistically. To get a full and accurate picture of trust, it is crucial to examine both general aspects, such as trustworthiness perceptions and trust attitudes, and instance-specific aspects, such as AI output acceptance and adoption decisions.
We also highlight that many factors influence trust in AI. In the below table, we organize the factors we identified based on whether they are related to the human trustor, the AI trustee, or the context. Human-related factors include domain knowledge and other factors influenced by it, such as the ability to assess the AI’s outputs, the ability to assess the AI’s ability, and the ability to use the AI. AI-related factors include internal factors such as ability, integrity, and benevolence; external factors such as popularity; and user-dependent factors such as familiarity and ease of use. Context-related factors include task difficulty, perceived risks and benefits of the situation, other situational characteristics, and the reputation of the domain and the developers. While this is not a complete set of factors that can influence trust in AI, what we observed in our case study, we hope it helps researchers and practitioners anticipate what can influence trust in AI in their context of interest.
Human-related | AI-related | Context-related |
Domain knowledge Ability to assess the AI’s outputs Ability to assess the AI’s ability Ability to use the AI | Ability Integrity Benevolence Popularity Familiarity Ease of use | Task difficulty Perceived risks and benefits Situational characteristics Domain’s reputation Developer’s reputation |
Between the lines
Our qualitative case study revealed a comprehensive picture of real end-users’ trust in AI, adding nuances to existing understandings. Yet, much remains to be explored. More research is needed on how trust is initially developed and changes over time and how trust in AI varies across stakeholders and user groups. In doing so, we urge the field to move from studying one or a few factors in lab settings with hypothetical end-users to studying multiple factors in real-world settings with actual end-users. This shift is necessary for understanding the interactions between factors and contextual influences on trust. We hope our paper, especially the way in which we delineated trust from its antecedents, context, and products, and the trust-influencing factors we identified, aid future research on other types of AI applications.