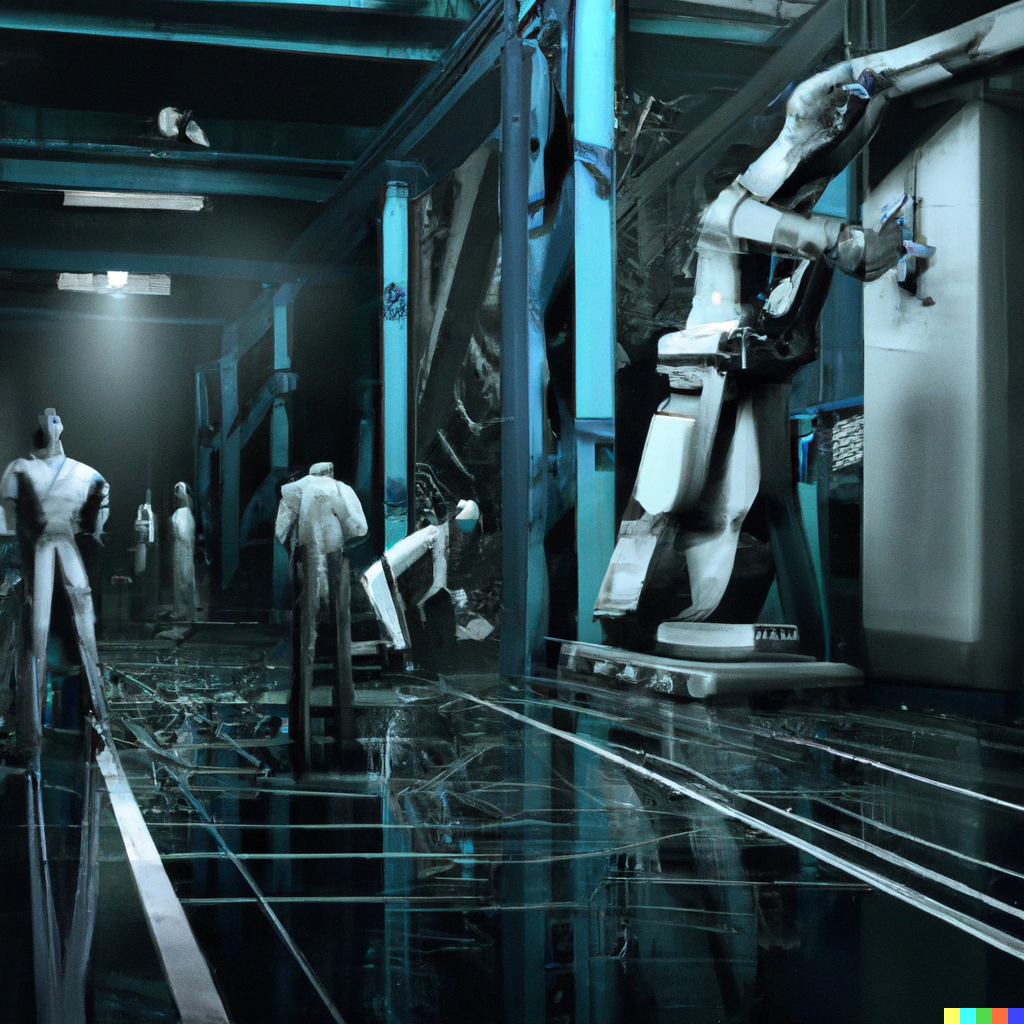
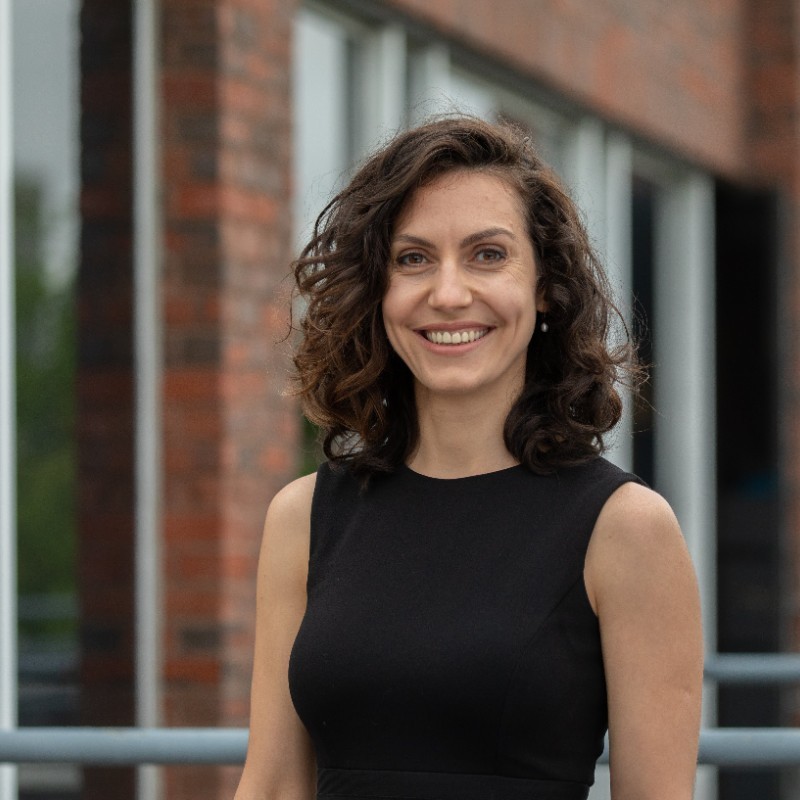
🔬 Research Summary by Katya Klinova, the Head of AI, Labor and the Economy at the Partnership on AI, where she works on developing mechanisms for steering AI in service of improving job access and job quality.
[Original paper by Katya Klinova]
This is a summary of a chapter from Bullock, Justin B. and others (eds), The Oxford Handbook of AI Governance (online edn, Oxford Academic, 14 Feb. 2022), https://doi.org/10.1093/oxfordhb/9780197579329.001.0001
Overview: A growing number of leading economists, technologists, and policymakers express concern over excessive emphasis on using AI to cut labor costs. This chapter attempts to give a comprehensive overview of factors driving the labor-saving focus of AI development and use. It argues that understanding those factors is key to designing effective approaches to governing the labor market impacts of AI.
Introduction
Figuring out how to enable the beneficial advancement of AI while protecting and expanding access to good jobs is a pressing AI governance challenge. Rising to this challenge does not require banning labor-saving AI—the composition of available jobs can evolve, with some jobs getting automated as long as new and better jobs replace them in quantities sufficient to employ the world’s working-age population. An abundance of well-paying, stable, and dignified jobs will be necessary until humankind can robustly and on a global scale decouple the elimination of jobs from the elimination of dignity, status, and access to income.
If AI is excessively used to economize on human labor in a world that functions as our world does today, this can lead to decreasing wages and increasing job precarity for many working people. To avoid that fate, a common suggestion is to develop AI that complements rather than displaces labor. This suggestion is not easy to act on for at least two reasons.
First, robust workers-centering methods for practically differentiating between labor-complementing and labor-saving AI are hard to come by. At the Partnership on AI, where I work, we tried to help close this gap by developing PAI’s Guidelines for AI and Shared Prosperity, available at https://partnershiponai.org/shared-prosperity.
Second, there are many powerful underlying factors channeling AI towards labor substitution and away from labor complementarity. Some get more attention than others; some are harder to measure than others but potentially no less influential. This chapter reviews them in turn.
Key Insights
To map the factors influencing what kinds of uses AI is put towards, I rely on Lawrence Lessig’s taxonomy of four modalities regulating any activity: legislation, markets, social norms, and architecture of the AI field. I argue that all four influence AI’s eventual impacts on labor demand.
The impact of legislation and markets on AI trajectory gets more attention in the economic literature. Hence several well-described insights highlighting, for example, how distorted labor-capital tax ratios and overly strict labor mobility policies overblow private incentives for automation. There are also harder-to-quantify factors that influence AI trajectory: which visions of the future capture the imagination of AI researchers? What problems are considered worth tackling? How are they defined, and what serves as a metric of success? What cultural artifacts underpin those?
Examining these questions uncovers multiple drivers of the AI field’s emphasis on “beating” humans at their basic abilities, potentially leading to a state Lant Pritchett aptly described as using some of the world’s scarcest talent (of AI researchers) to make decent jobs harder to find.
Governing AI for inclusive economic outcomes requires paying attention to the broad policy environment (not just “AI policy” but also migration, tax, and other relevant policies), as well as to harder-to-pin down factors driving the focus of the AI field on chasing human parity, such as:
- orienting benchmarks of the AI field, often tied to human performance;
- sociotechnical imaginaries collectively performed by the AI field, heavily inspired by certain kinds of sci-fi stories;
- structural features of the AI field, where commercially-oriented private sector out-influences academia.
Government policies incentivizing the development of labor-saving AI
Aside from public R&D and industrial policies frequently discussed in conversations about “AI policy,” many other policies are relevant in AI governance, particularly those that shape incentives faced by firms developing and deploying AI. Those policies include the rules around taxation, migration, interest rate, corporate governance, and more. Currently, in the US and many other OECD countries, those policies tilt the playing field towards incentivizing labor-saving uses of AI. For example, rich countries tend to tax capital, software, and automation investments at much lighter rates than investments in labor. They also typically heavily constrain immigration, creating artificial labor scarcities, which further boost commercial incentives to invent and use labor-replacing machines. Notably, those machines often end up displacing or making jobs more precarious domestically within rich countries and in lower-income countries struggling to provide sufficient employment opportunities for their young and growing labor forces.
Definitions of Success and Choice of Problems to Tackle
The AI field’s orientation is influenced by its members’ choice of problems to work on and the commonly used definitions of progress. Whether the field addresses social or commercial challenges and what kind of achievements are associated with reputational gains and prestige influence the impacts the field generates on society.
Chasing state-of-the-art performance on benchmark datasets has emerged as a common goal in the subfields that constitute today’s AI R&D. Some commonly used benchmarks in computer vision, language processing, and reasoning unambiguously map to the goals of imitating basic human abilities. Corresponding leaderboards explicitly recognize which AI models surpassed a “human baseline,” normalizing and incentivizing a “competition” between humans and AI models. There is currently a lack of benchmark datasets deliberately designed to evaluate the ability of an ML model to assist a human worker and boost her productivity in non-exploitative ways.
Sociotechnical imaginaries driving AI Development
Influential ideas about the desirable technological future also serve as an orienting force for AI researchers to choose what problems to work on. Those ideas are shaped in part by popular science fiction stories. Particularly notable among those is Star Trek, which popularized the ambition to build technology that enables material abundance, freeing people from the economic need to sell their labor.
What gets dangerously lost in attempts to build Star Trek-inspired technology is that we do not know whether, in real life, the automation of all or the majority of paid tasks (if it is feasible at all) would actually result in broadly shared prosperity. History suggests that the probability of economic and political power being voluntarily redistributed from technological “winners” to the less fortunate is remarkably low. In the past, this redistribution was often prompted by extended and brutal struggle, with never certain outcomes. The situation today is made more complex by the political opposition to cross-border redistribution of income. In other words, even if the government of a certain country eventually gains the ability to levy taxes on its technological leaders in amounts sufficient to replace the labor incomes of its citizens, prevailing global norms deem it completely acceptable, and even expected, to deny non-citizens access to these tax revenues.
Between the lines
A commonly raised (and well-founded!) objection to the call to deliberately govern AI in service of shared prosperity argues that it is too difficult to predict whether a given class of AI technologies would boost or dampen the demand for human labor. For example, applications based on large language models can complement and displace human labor. This is a valid argument, but it does not remove the imperative to examine the factors influencing what kind of uses AI is put towards. As this analysis shows, at present, we are likely heavily incentivizing the labor-saving uses of AI. If we do not deliberately adjust the commercial incentives faced by AI-creating and AI-using firms or introduce alternative visions for the AI field, we might well end up having eliminated too many stable, well-paying jobs long before we build robust global mechanisms for providing people with access to alternative sources of income.