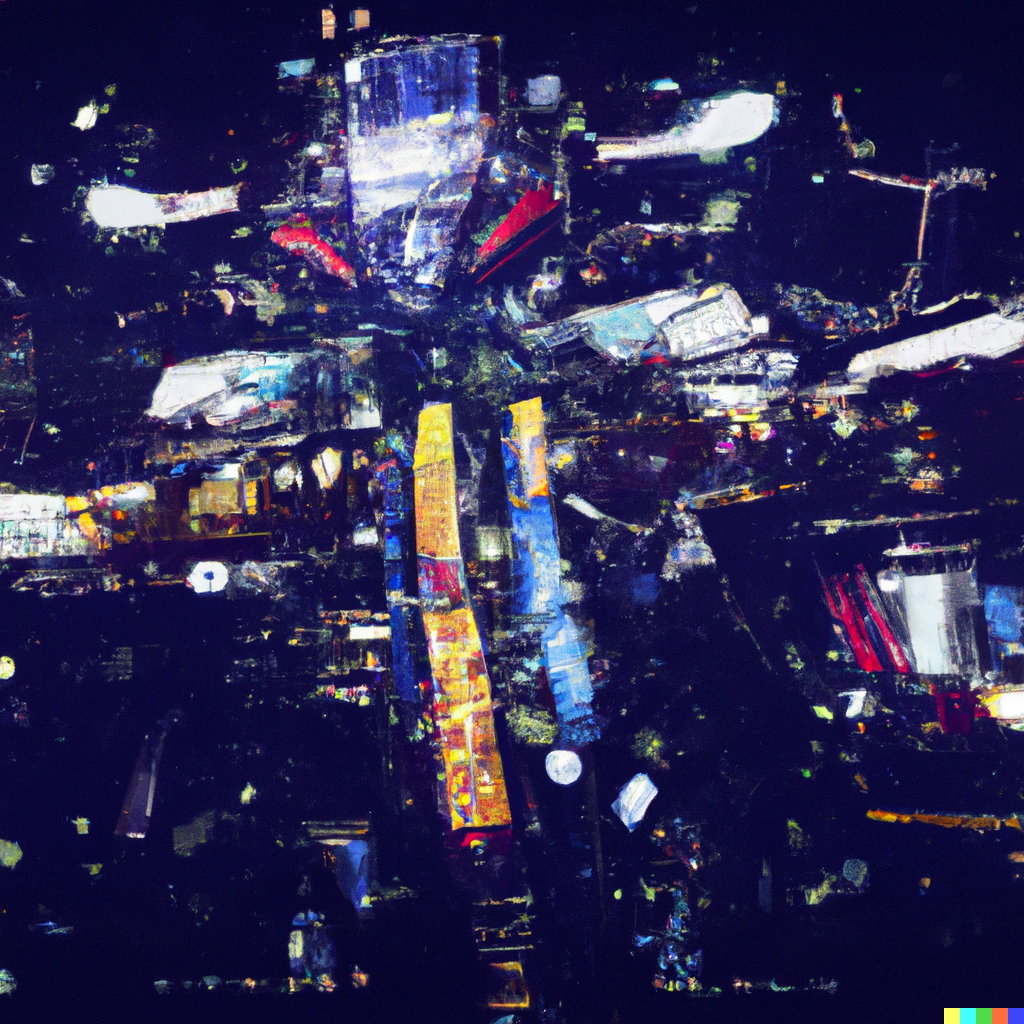
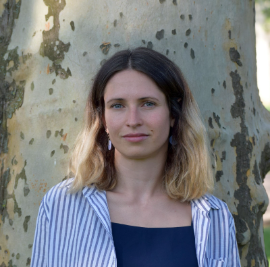
🔬 Research Summary by Dasha Pruss, a postdoctoral fellow at the Berkman Klein Center for Internet & Society and the Embedded EthiCS program at Harvard University. Dasha’s research focuses on algorithmic decision-making systems in the US criminal legal system.
[Original paper by Dasha Pruss]
Overview: Algorithmic risk assessment is often presented as an ‘evidence-based’ strategy for criminal justice reform. In practice, AI-centric reforms may not have the positive impacts their proponents expect. Through a community-informed interview-based study of judges and other legal bureaucrats, this paper qualitatively evaluates the impact of a recidivism risk assessment instrument recently adopted in the state of Pennsylvania. I find that judges overwhelmingly ignore the new tool, largely due to organizational factors unrelated to individual distrust of technology.
Introduction
Amid the chaos of the pandemic’s early months, Pennsylvania criminal courts were instructed to begin consulting the Sentence Risk Assessment Instrument when sentencing crimes. The actuarial tool uses demographic factors like age and number of prior convictions to estimate the risk that an individual will “re-offend and be a threat to society” – that is, be reconvicted within three years of release from prison.
The instrument was developed to help judges identify candidates for alternative sentences, with the ultimate aim of reducing the prison population. However, through interviews with 23 criminal judges and other legal bureaucrats throughout the state, I found that that has not happened. In fact, judges routinely ignored the tool’s recommendations, which they disparaged as “useless,” “worthless,” “boring,” “a waste of time,” “a non-thing,” and simply “not helpful.” Others weren’t even aware that their courtrooms were supposed to be using it.
Recidivism risk assessment instruments are used in high-stakes pre-trial, sentencing, or parole decisions in nearly every US state. These algorithmic decision-making systems, which infer a defendant’s recidivism risk based on past data, are often presented as an ‘evidence-based’ strategy for criminal justice reform – a way to reduce human bias in sentencing, replace cash bail, and reduce mass incarceration. Yet there is remarkably little evidence that risk assessment instruments help advance these goals in practice.
The discourse around tools like the Sentence Risk Assessment Instrument has focused on their technical aspects, particularly racially biased predictions. Studies of risk assessment tools also tend to be conducted without the input or expertise of communities impacted by incarceration. By contrast, this research focuses on how judges actually use the tools, using interview questions developed with input from the community organization Coalition to Abolish Death by Incarceration (CADBI). This work sheds new light on the important role of organizational influences on professional resistance to algorithms. This helps explain why AI-centric reforms can fail to have their desired effect.
Key Insights
The importance of human discretion
Studies of risk assessment instruments tend to assume, with no empirical basis, that human decision-makers rely uncritically on predictive instruments – which are often advisory. In practice, judges differ widely in their adherence to algorithmic recommendations and follow them inconsistently for different types of defendants.
In some places, risk assessment instruments have been found to exacerbate the racial bias in judges’ decisions. A pretrial risk assessment tool in Kentucky – intended as a bail reform measure – increased racial disparities in pretrial releases and ultimately did not increase the number of releases overall because judges ignored leniency recommendations for Black defendants more often than similar white defendants. Likewise, judges using a risk assessment instrument in Virginia sentenced Black defendants more harshly than others with the same risk score.
In other contexts, human discretion has been found to correct for algorithmic bias. In Pennsylvania, a recent study about racial bias in an algorithm that screens child neglect showed that call screeners minimized the algorithm’s disparity in screen-in rate between Black and white children by “making holistic risk assessments and adjusting for the algorithm’s limitations.” Virginia’s risk assessment instrument would have led to an increase in sentence length for young people had judges adhered to it; however, because judges systematically deviated from recommendations, some of the instrument’s potential harms (and benefits) were minimized.
Of course, another way that human discretion can interact with algorithms is to choose not to interact with them. Sociological work shows that algorithm aversion — the reluctance to follow algorithmic recommendations — can happen when individuals feel that their agency or power is being threatened by a new technology. This is artfully illustrated by Sarah Brayne in her ethnography of LAPD officers using PredPol and by Angèle Christin in her ethnography of prosecutors and judges using a pretrial risk assessment instrument. Police officers and legal professionals reported feeling threatened by how these new technologies could be used to surveil their performance and limit the role of their discretion, resulting in professional resistance to algorithmic systems.
Understanding how these possible forms of human-algorithm interaction apply in a given case requires empirical research in the context of application and attention to the social and organizational factors at play.
Why do judges ignore the tool?
The judges I spoke with, who I interviewed with guidance from CADBI, ignored the tool’s recommendations for various reasons. The most common was simply that judges found the tool to “not be particularly, um… helpful.” This is partly due to the work of activists, lawyers, and academics who, over years of public testimony hearings, successfully pressured the Pennsylvania Sentencing Commission to remove the most controversial parts of the instrument, which included directly showing judges’ risk scores and detailed recidivism risk distributions. The implemented version of the tool encourages judges to order “additional information,” typically a presentence investigation report, for low- and high-risk defendants, with the presumption that information contained within these reports will, in turn, influence a judge’s decision to assign an alternate sentence.
However, none of the judges I spoke with expressed interest in changing their report-ordering behavior, and I found that the norms for ordering reports varied widely by county. In many counties, including Pennsylvania’s most populous Philadelphia and Allegheny counties, the reports contain information judges can get simply by talking to the defendant, so judges often lamented that the reports themselves were unhelpful. In other counties, presentence investigation reports already contain an additional, controversial “black-box” risk assessment; judges in those counties explained that they saw no need for an additional risk assessment instrument. Over half of the judges I spoke with also said they would have preferred to receive more meaningful information at sentencing time, such as which interventions have the best outcomes for cases involving drug use.
It was also common for judges to be unfamiliar with what the Sentence Risk Assessment Instrument did or where to find its recommendations. As one judge said, “I never knew where that information would be provided for me. Was it going to come in an email? A news blog? A winter weather alert? I had no idea.” This is partly because the Pennsylvania Sentencing Commission’s information campaign was derailed by the pandemic’s start, but my findings also indicated systemic problems with how information is disseminated to judges. In a particularly revealing moment, one judge told me they were attending a virtual training session over video call — during our interview.
Most judges were also concerned about the tool’s potential for racial bias and dehumanization. One judge said they were concerned about “having a formula that takes away my ability to see the humanity of the people in front of me.” Another judge, who identified as Black, was critical of the tool’s discriminatory potential: “Who’s making the determinations? Who’s interpreting the statistics? You can say anything with statistics.” Finally, many judges felt that the tool was worse than the discretion of experienced judges — in one judge’s words, “I was elected to be a judge, not a robot.” These concerns, however, varied widely and were secondary reasons for not using the tool; even judges who were self-described “cheerleaders” for risk assessment instruments were dismissive of this particular tool.
Potential unexpected harms of a “useless” tool
Were it to be used, however, the Sentence Risk Assessment Instrument could have harmful downstream consequences. The Commission “expressly disavows the use of the sentence risk assessment instrument to increase punishment.” However, as several judges pointed out, it is possible to infer a defendant’s risk score from the “additional information” designation given to low- and high-risk defendants, and empirical evidence from other states suggests that judges are more likely to use risk information to detain individuals longer.
Moreover, were judges to follow the tool’s recommendation to order reports for low-risk defendants, who often have minor sentences, the tool could have the unintended effect of detaining these defendants longer pre-trial — ordering a report can take 60 days. As one judge remarked, “I’m not letting them [the defendant] sit eight more weeks in jail because some computer program said so.” Probation officers, who are typically in charge of creating presentence investigation reports, told me they feared they were going to get “a flood of cases,” but that “thankfully that has not happened” because they were already overwhelmed with cases and lacked the resources to handle such a surge.
Between the lines
Algorithm aversion from an organizational perspective
A standard explanation for algorithm aversion is individuals’ lack of confidence in algorithms. Distrust is, no doubt, an important part of this story, particularly with respect to public resistance to the Sentence Risk Assessment Instrument. But lack of confidence does not explain the resistance I saw from judges. In fact, most judges I interviewed wanted more data at sentencing time.
A more adequate explanation for why judges ignore the tool has to do with the organizational influences that led to the tool’s development, policies about the contents of presentence investigation reports, and structural problems in how information is disseminated to judges. This research thus strengthens a call to further understand algorithm aversion in real-world contexts rather than solely in laboratory settings. I believe that studying algorithm aversion – and other dynamics of human-algorithm interaction – from an organizational perspective is a promising future area of research; such work requires attention to social context and the knowledge and participation of affected stakeholders.
A resource argument against risk assessment instruments
The Sentence Risk Assessment Instrument was the locus of considerable time and taxpayer dollars; it was in development for nearly a decade following a 2010 state legislative mandate for adopting a risk assessment tool for sentencing. Despite having no impact — a finding corroborated by the Pennsylvania Sentencing Commission’s own initial data analysis — the final version of the tool satisfies this mandate, producing the false impression that some evidence-based measure has been taken to address Pennsylvania’s crisis of mass incarceration and racial disparities in sentencing.
Some activists still see the final weakened tool as a win, viewing a harmless risk assessment instrument as better than a harmful one. In my view, however, this case illustrates the kinds of insidious algorithmic harms that rarely make headlines, adding to the growing body of empirical support for the abolition of recidivism risk assessment instruments. In practice, these algorithm-centric reforms have no significant impacts on sentencing, are resource-intensive to develop and implement, and merely pay lip service to addressing the mass incarceration crisis. For decades, grassroots organizations such as CADBI have promoted low-tech policy changes, including abolishing cash bail, releasing elderly populations from prison, and reinvesting money in schools and communities. Unlike risk assessment instruments, such measures do not rely on individual judges’ alignment with policy goals and have robust empirical support for reducing prison populations.