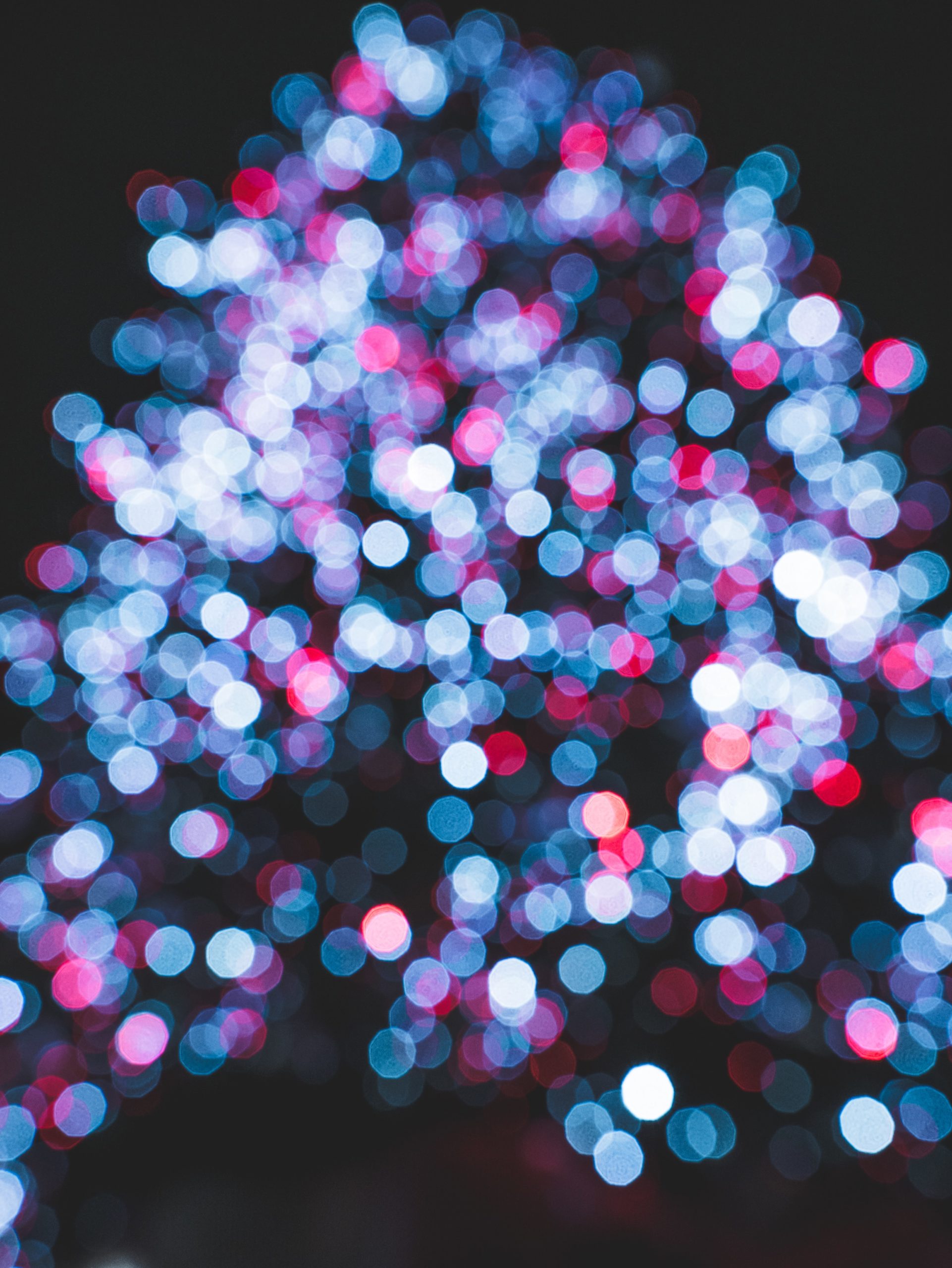
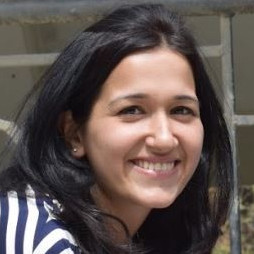
š¬ Research summary by Vidhi Chugh, an award-winning AI/ML innovation leader and an advocate for the ethical and responsible use of AI. She has conducted several workshops demonstrating how to integrate ethical principles into AI-enabled products.
[Original paper by  Jessica Morley, Anat Elhalal, Francesca Garcia, Libby Kinsey, Jakob Mökander , Luciano Floridi]
Overview: With increasingly ubiquitous AI comes the greater need for awareness of ethical issues. However, the current regulations are inadequate to save humanity from the possible AI harm and hence enter a stream of guidelines, frameworks, and ethics codes. This paper talks about the effective operationalization of AI ethics in algorithm design.
Introduction
The gap between esoteric ethical AI principles vs the practical implementation is proving varied ethics frameworks futile. Though the development of principles-based policies and frameworks was a significant step in the evolution of AI governance, they continue to be abstract from an implementation perspective. The team doing the groundwork i.e. developers and engineers need to objectively translate principles into practice. The paper describes how current translational tools are either too flexible leading to a waste of effort or too strict lacking ārightā interpretation and implementation of ethics. The authors introduce āEthics as a serviceā by drawing the analogy from the cloud computing model and share how this moderation is helpful in ethical algorithm design.
Ethics – abstract concept or objective tool?
The key to effective ethical AI implementation lies in enabling AI practitioners with not only what to do but also how to do it. The translational tools have been helpful in raising awareness and interpreting principles within research forums and organizations, but the impact and external validation in terms of helping disadvantaged groups are yet to be gauged.
According to the Global Inventory of AI Ethics Guidelines, managed by Algorithm Watch, currently, 160 documents exist that talk about – beneficence, non-maleficence, autonomy, justice, and explicability principles. The authors highlight with an example how the mention of āAI systems may be discriminatoryā is ineffective and vague in embedding ethics into the design. The various risks arising from such broad and generic guidance include ethics washing, ethics lobbying, ethics dumping, and ethics shirking among many. While these principles intend to lay the foundation, the tools focus on the āhowā of technical specifications.
Hence, the need of the hour is to bridge the gap between the abstract principles and technical tools leading to the evolution of the concept āEthics as a Serviceā.
Limitations of traditional tools
- Open for manipulation, it depends on the practitionerās understanding of the principle as against the societyās preferred understanding
- Diagnostic vs prescriptive:
- For example, flags biased data but do not suggest measures to mitigate
- Parameters in assessing fairness, transparency, or accountability are set by the practitioners and demand objectivity
- How to identify who is the decision-maker – tool or the user?
- Ownership of associated risks and injustices, thereof
- Viewed as one-time compliance evaluation: Induces a false sense of security unless monitored timely during the project lifecycle.
As ethical implications vary based on the domain, context, specific product, country, algorithm, and even stage of deployment, hence it is difficult to assess the non-deterministic impact and design a one-approach solution that fits all.
The paper proposes the ethical AI practice as a reflective development process, highlighting that the practitioners need to be apprised of their own subjectivity and bias as well.
Effective operationalization requires the organization to ensure:
- Inclusion and discursion: Not just the agreement with all practitioners involved but also the effect of the product on users need to be reviewed periodically
- The repeatable process to attain the ethical justification and environmental sustainability within the specific context
- Appropriate oversight measures during validation, verification, and evaluation stages
External audits
The paper highlights below frameworks quoting their significant role in operationalising ethics:
- Aequitas: an open-source toolkit for bias and fairness audit
- Turingbox: a platform that audits the explainability
- AI auditing framework developed by UKās Information Commissioner’s Office that ensures organizations are compliant with data protection requirements and honor fairness, accuracy, security, and fundamental rights
- PwCās Responsible AI framework
However, it doubts the role of external auditsā efficacy as they are conducted after deployment, further discerned by the legal issues arising from consumer data protection and trade secrets.
Quoting the limitations related to data access and appropriate technical documentation to assist external independent and objective analysis, the paper brings out Ethics as a Service.
The authors draw an analogy from the cloud computing model:
- Software as a Service: Third-party dictates ethical principles, guidelines, processes, and audits to ensure a positive outcomeĀ
- Infrastructure as a Service: Practitioners responsible for internal processes and principles, runs the risk of being too flexible
- Platform as a Service: A bridge between devolved and centralized governance maintaining responsibility among different stakeholders:
- Independent multi-disciplinary ethics advisory board
- The internal company employees
Source: Original paper
Between the lines
We have to ensure that the assumptions, data collection, data limitations, proxy data curation, etc need to be well documented to assist the external audits, besides internal checks and code reviews. Notably, the role of external AI ethics audits is relatively new and would play a crucial role in regulating AI products, as has been evident in the financial industry for a long period. The amalgamation of the two approaches introduced in the paper as Ethics as a Service is one of the many steps that are needed to develop human-centric trustworthy AI systems.