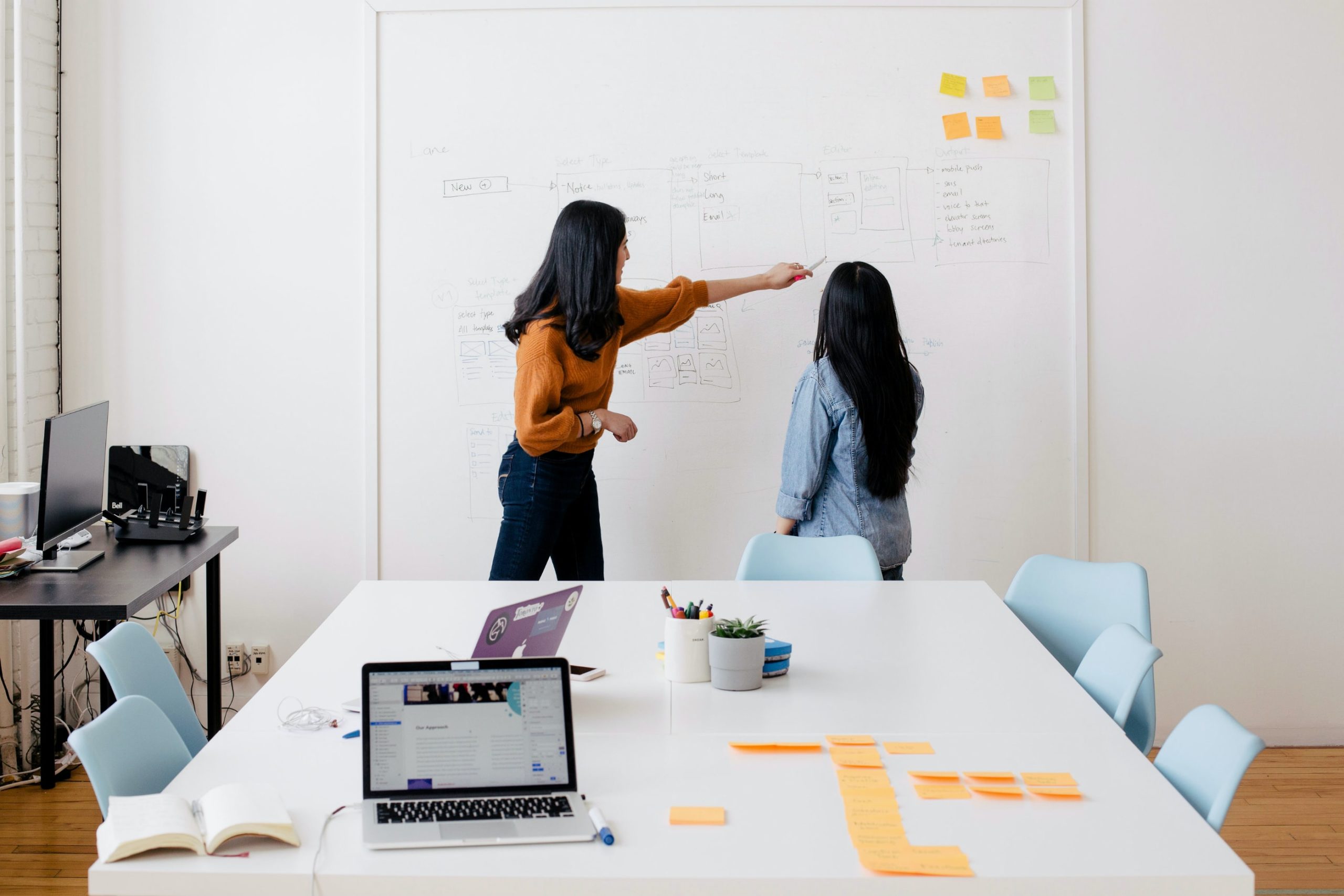
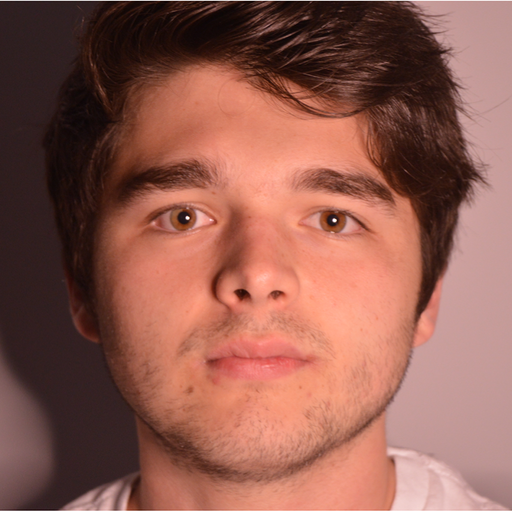
🔬 Research summary by Connor Wright, our Partnerships Manager.
[Original paper by Claire Su-Yeon Park, Haejoong Kim, Sangmin Lee]
Overview: With new online educational platforms, a trend in pedagogy is to coach rather than teach. Without developing a critically evaluative attitude, we risk falling into blind and unwarranted faith in AI systems. For sectors such as healthcare, this could prove fatal.Â
Introduction
With the proliferation of online education opportunities, a new tendency in higher education is to focus more on coaching rather than teaching when it comes to AI. Allowing students to be able to evaluate information rather than simply being spoon-fed exam material critically will prove essential to guiding an appropriate AI future. This will prove pertinent to the healthcare field, where we ought to treat technology as a tool rather than a replacement. To best explore this, I’ll first touch upon the new trend emerging in pedagogy before exploring the dangers of uncritical uniformity, later applying it to healthcare. To end, I’ll comment on how the better our skills are, the better this will be reflected in AI systems themselves.
Key Insights
A new trend in pedagogy
Given the rise of new online education opportunities after the pandemic, higher education appears to be in a transition phase. Higher education is becoming more proactive rather than reactive, especially regarding AI. The shift swings away from simply disseminating knowledge to guiding students’ critical thinking journey.
Recommender algorithms already allow students to personalise the content they view and discover new information. Hence, we are seeing a stronger trend in independent learning in addition to any course material, placing students in a more active role than before the pandemic. Said transition thus points towards equipping students with the tools to evaluate information and not just being able to reproduce it on an exam paper.
Uncritical uniformity
Without being critical, the users of recommender algorithms could fall into reproducing the interests of the developers. If they are not careful, the subtle shifts towards the “specific bias” (p.98) a system could contain will go undetected—for example, engaging in newspaper websites that recommend news items associated with their political leanings. Here, if we don’t question, we could fall party to isolating ourselves in our own views.
Building on this, being uncritical leads to unwarranted blind faith in AI systems. Computers cannot think critically for us, but they can reflect our biases. For example. The Korean Scatter Lab-designed chatbot Lee Luda reproduced homophobic and transphobic comments when asked specific questions (Figure 1). Adopting an uncritical attitude to this technology would’ve proved detrimental and allowed this technology to continue to operate without punishment. Nevertheless, the company was castigated with a KRW 13.3 million fine.
Virtual education and healthcare
Involved within these considerations is how, without a critical attitude, it may become difficult to tell the difference between what a student believes and what the online learning system wants them to think. Being constantly fed particular articles eventually becomes difficult to label as reflective of the user or the system itself. Without critically evaluating such information, we become paralysed in our efforts to discern and assert what they believe in.
This proves poignant within the healthcare system. Without developing a critically evaluative outlook, we risk not being able to intervene in AI-driven decisions that could lead to the endangerment of patient welfare. For example, for those who arrive at a hospital with flank pain, the STONE algorithm uses its “origin/race” factor to predict the likelihood of kidney stones in patients. It subsequently adds three points (out of 13) to non-white patients, which could lead to non-white patients not being referred to a specialist even when they need treatment. Hence, while this technology is beneficial, it should be treated as a tool that augments rather than replaces human intelligence.
Between the lines
AI is omnipresent within our lives, from YouTube recommender algorithms to allow us to unlock our phones through facial recognition. Consequently, equipping the standard citizen and students with the dexterity to evaluate AI systems is a must. The better our skills, the more these will be reflected in the AI, its outcomes and how we deal with any negative consequences that arise.