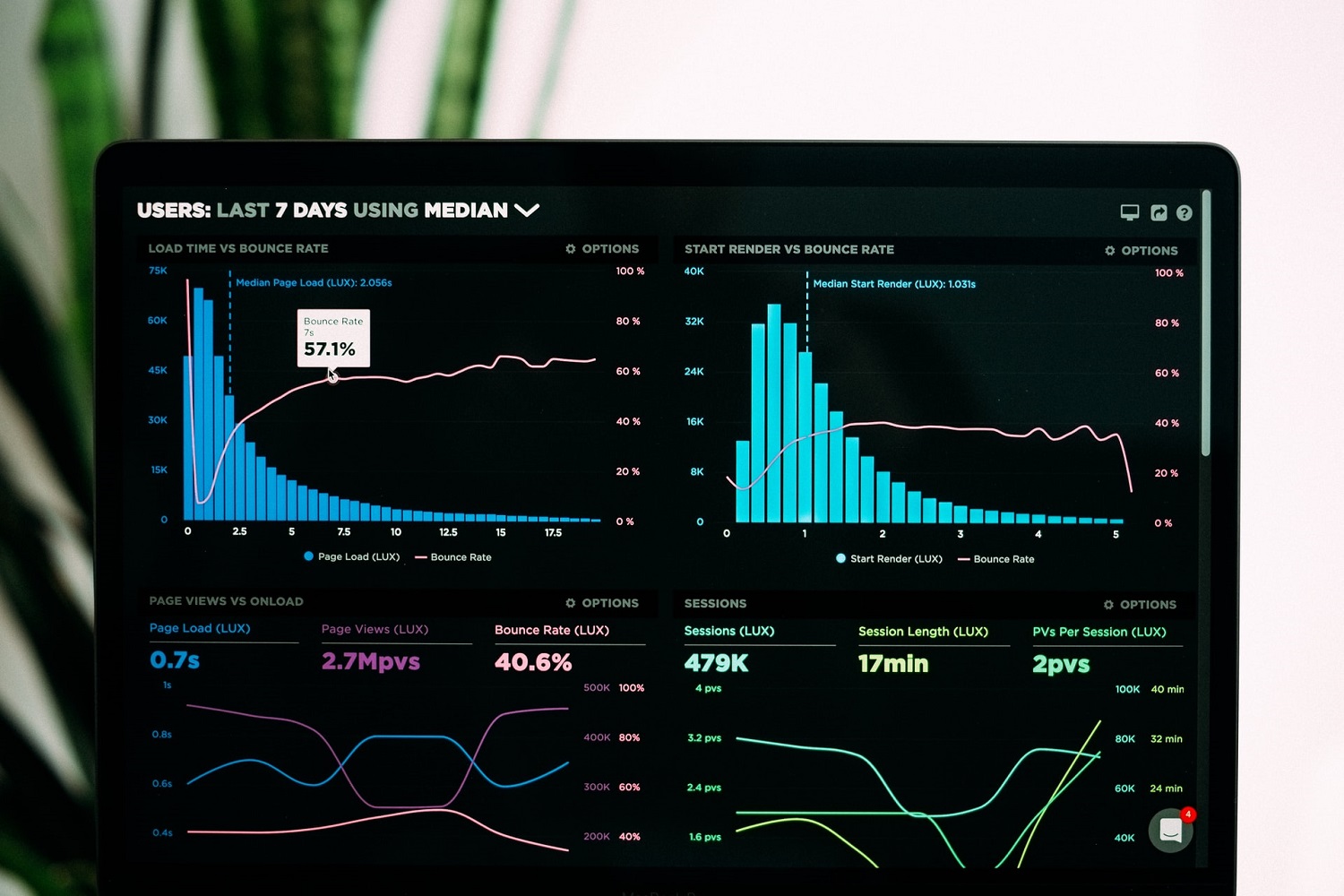
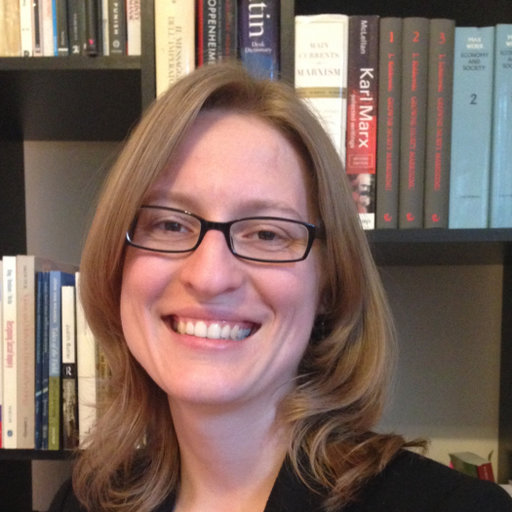
🔬 Research summary contributed by Dr. Iga Kozlowska (@kozlowska_iga), a sociologist working on Microsoft’s Ethics & Society team where she’s tasked with guiding responsible AI innovation.
✍️ This is part 6 of the ongoing Sociology of AI Ethics series; read previous entries here.
[Original paper — titled Critique and Contribute: A Practice-Based Framework for Improving Critical Data Studies and Data Science, by Gina Neff, Anissa Tanweer, Brittany Fiore-Gartland, Laura Osburn]
Overview: Neff and co-authors, through participant observation, examine how academic data science teams grapple with the ethical and social challenges of their work. The authors address data science critiques common in critical data studies and show how these concerns are manifest in the day-to-day practices of data science practitioners. They conclude by encouraging social science and humanities scholars and data scientists to work more closely together for the benefit of each discipline and to produce more ethical ways of knowing.
Data science is a critical field in the organizational ecosystem of AI development. After all, AI is nothing without data. Data scientists, machine learning scientists, and engineers work closely in academic and industry settings to build AI systems. In order to build more ethical algorithms, we need to take a closer look at data and how data scientists work with data.
Neff et al.’s ethnographic work does just this. They examine four key critiques of data science posed by social science and humanities scholars critical of data science approaches that claim objectivity and deny the sociality of data-based knowledge production. These are:
(1) data as interpretation,
(2) data as context,
(3) data as mediated by social–technical arrangements, and
(4) data as the medium of negotiation.
Data Science Critiques
Let’s examine each in turn. First, data is inherently open to interpretation. This means that what we call data and how we compose datasets inherently requires human judgement and subjectivity. It is not something that can be stripped out of data in order to be “objective.”
Second, data loses its meaning and value when ripped from its context. For big data projects to be interpretable and reproducible, data scientists need to provide context around how datasets were constructed, where, when, and how the data were collected, and for what purpose. There are many ideas on how to do this in the machine learnings space (see, for example, datasheets for datasets).
Third, we make sense of data through the full assemblage of software, hardware, tools, instruments, protocols, documentation, and, most importantly, people that are engaged in producing data. In other words, data becomes interpretable and useful only through the social relationships through which it’s mediated. The “storytelling, guesswork, and negotiation” that takes place among various stakeholders involved in a data science project (customer, data scientist, engineer, subject matter experts, project managers, marketers etc.) shapes the data.
Finally, data inherently contain value judgements as they structure relationships between people. For example, making trade-offs between what type of data to collect and whose data to analyze may privilege one group of people over another. These are choices that need to be made consciously and in a principled way.
What Does This Mean for Ethical AI?
The authors provide some suggestions on how data science can be improved based on lessons from critical data science studies. These same lessons can be applied to AI development since data science is at the heart of training machine learning algorithms.
First, the authors encourage practitioners to acknowledge communication – talking about data, translating data for different audiences – as a central activity of data science, much like data cleaning and data analysis. By not devaluing this “communicative labour,” AI teams can fully embrace the social nature of their work rather than pretend that it lies somehow outside of social relationships and culture.
In addition, the authors stress that it’s important to remember that making sense of data is a collective process. This should prompt AI developers to invite various stakeholders who will be interfacing with the AI system once it’s deployed into the development process early on, ideally at the design stage. The interpretability and usability of the AI product are never self-evident and may be different for different people in different contexts or from different backgrounds. Lack of strong user research or other practices (see, for example, community juries) that engage a variety of direct and indirect stakeholders can mean the success or failure of the system as envisioned by the product team.
Third, we must remember that data about people requires us to think about data dignity. Before the data is even collected, AI teams need to consider how and from whom the data will be gathered. Questions like who gets to be calculated, what choice do they have over it, and how they can control their self-representation through data are all critical to a successful AI project.
Finally, the authors suggest we think of data as stories. This is a bit counterintuitive because we tend to think of data as numbers and stories as made up of words. The former is quantitative and the latter is qualitative. But for each data-based project, there is a narrative about what we expect the data to tell us and who the intended audience is. Listening to how data is weaved into the stories that we tell about the project or product helps us be clear about the goals and values built into it. Being transparent about these intentions with end-users helps them decide if our story rings true or hollow based on their own values and needs.
Conclusion
Fundamentally, data is social, just like all technologies based on measuring the world. This means that AI is social too. Paying attention to the ways in which AI is embedded in and shaped by our social relationships does not detract from its scientific-ness. It does not mean it’s more biased or less good. Quite the opposite. Noticing how human values, judgements, and cultures shape our work is central to building more fair, reliable and accountable AI. Recognizing that computational sciences aren’t separate and apart from social contexts and relationships gives us a chance to address these social forces head-on and with our eyes wide open. As the authors point out, humanists and social scientists can play an important role in helping engineering teams deal with these social issues. But only if we save them a spot at the table.
Neff, G., Tanweer, A., Fiore-Gartland, B., & Osburn, L. (2017). Critique and Contribute: A Practice-Based Framework for Improving Critical Data Studies and Data Science. Big Data, 5(2), 85–97. 10.1089/big.2016.0050