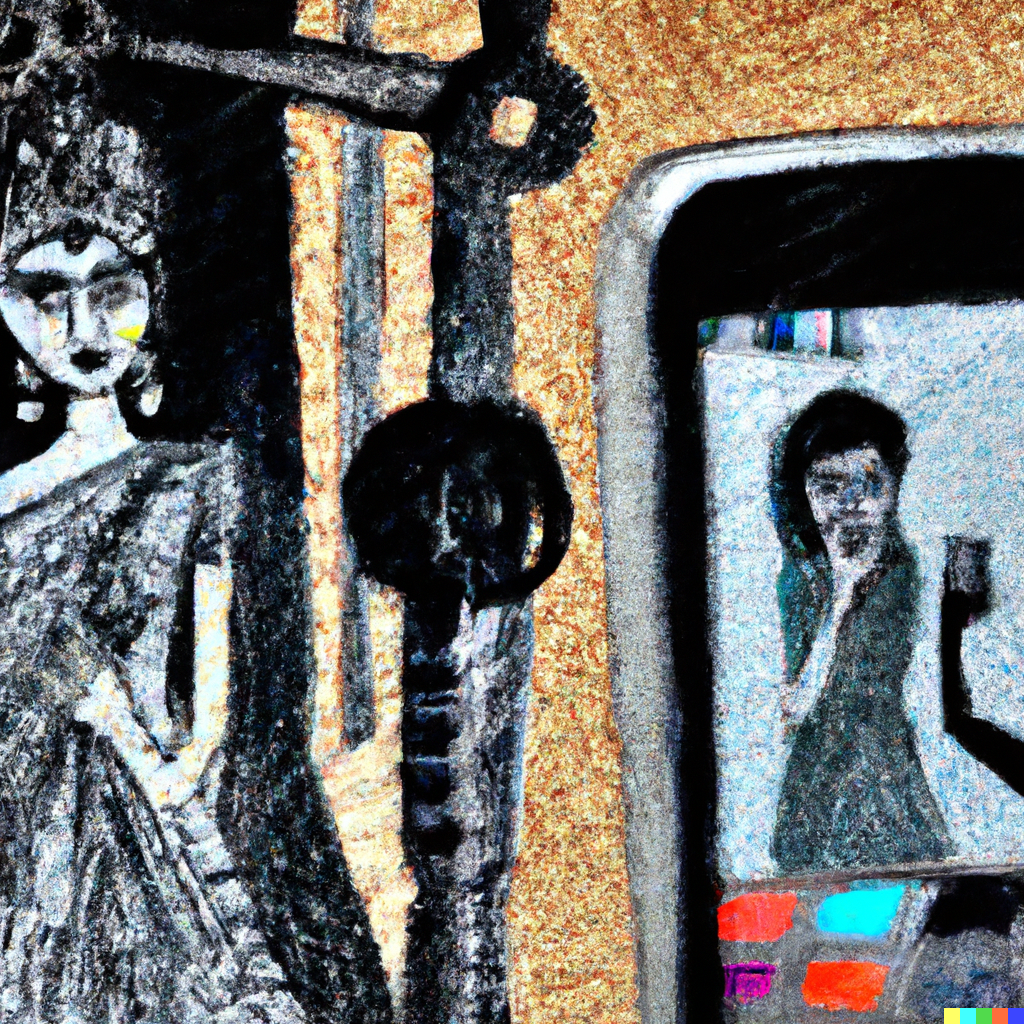
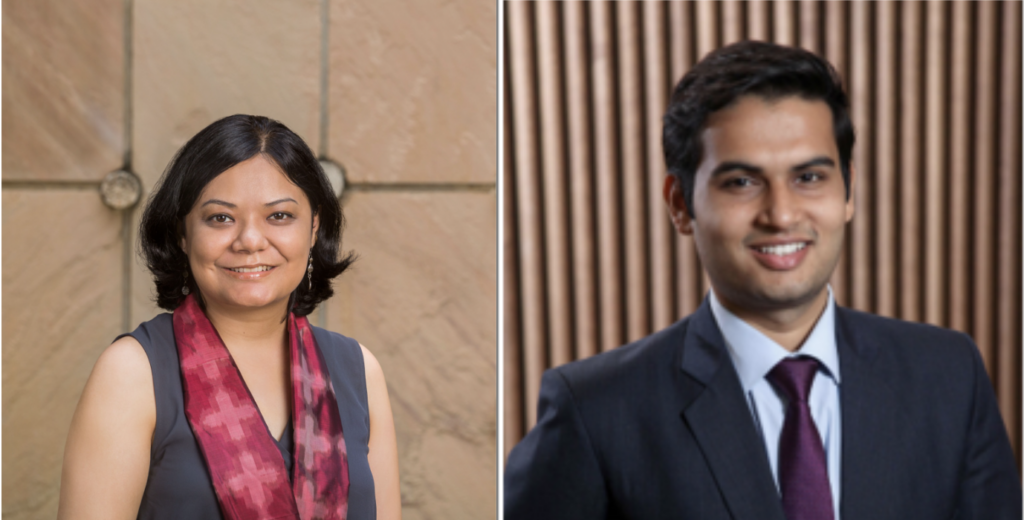
🔬 Research Summary by Smriti Parsheera and Gaurav Jain.
Smriti Parsheera is a Fellow at the CyberBRICS Project, FGV Law School, Rio de Janeiro and a PhD candidate at the Indian Institute of Technology Delhi.
Gaurav Jain is a sector economist with International Finance Corporation and was previously a Young Leader in Tech Policy with the University of Chicago Trust in India.
[Original paper by Gaurav Jain and Smriti Parsheera]
Overview: The widespread adoption of facial processing systems poses several concerns. One of these concerns emanates from claims about the accuracy of these technologies without adequate and context-specific evaluation of what accuracy means or its sufficiency as a metric. Focusing on the Indian context, this paper takes a small step in that direction. It tests the face detection and facial analysis functions of four commercial facial processing tools on a dataset of Indian faces. The goal was to understand the facial processing outcomes for different demographic groups and the likely implications of those differences in the Indian social and legal context.
Introduction
India recently acquired the status of being the most populous country in the world. It is also a country that is seeing the rapid adoption of facial processing technologies (FPT) for purposes as wide as law enforcement, airport entry, know-your-customer (KYC) verification, and attendance in educational institutions. Given this context, it is problematic to learn that we still know very little about how these technologies perform on the diverse features, characteristics, and skin tones of India’s 1.34 billion-plus population.
In this paper, we audited the face detection and analysis functions of four commercially available FPT tools, Microsoft Azure’s Face, Amazon’s Rekognition, Face++, and FaceX, on a dataset of Indian faces. We did this using publicly available images of election candidates sourced from the website of the Election Commission of India (ECI). Specifically, we looked at how these tools perform in face detection, gender classification, and age estimation functions on the ECI dataset of Indian faces.
Our findings pointed to significant variations in the performance of these tools. There were also clear group-specific trends observed across the tools. Further, a comparison with the results of previous studies, including the 2018 Gender Shades study by Buolamwini and Gebru, showed the lack of transferability of findings from audits done in other international contexts.
Key Insights
Setting the context
The developers and adopters of FPTs often rely on claims about these tools’ efficiency, performance, and accuracy to make a case for greater adoption. However, very little evidence exists about the actual performance of facial processing tools on the diversity of Indian faces. At the same time, researchers have observed that even the framing of the debate around unfairness and bias in facial processing is often shaped in contexts that are very removed from the Indian one. Therefore, a need was felt to build upon the work in the field of algorithmic auditing studies while keeping in mind India’s specific legal, societal, and institutional context.
A new dataset of facial images
One of the factors why empirical research on the performance of facial processing in India remains limited may be the scarcity of appropriate image datasets. We analyzed the available datasets of Indian faces. We found them lacking for our purposes because of either covering only a limited number of unique individuals or the lack of diversity among the covered subjects. Accordingly, we created a new dataset of facial images, the ECI Faces Dataset, for this research. The ECI Faces Dataset consists of 32,184 observations with facial images, age, and gender of candidates who had filed nominations to contest in Indian Parliamentary and State Assembly elections during an identified period of 18 months.
Ethical considerations
While creating the ECI Faces Dataset, the paper considered the public availability of the data and the decision then by the individuals to be part of public life. However, given the sensitivity of facial biometric data and possible intrusive applications, several other steps were taken to minimize direct or indirect harm.
Following the principle of data minimization, the data collection did not include any additional data points (like financial records of candidates), even though these were publicly available. To ensure geographic representation, the selected study period covered a General Election that implies the representation of candidates from all the constituencies in the country. Finally, to protect individual privacy, the paper does not display any personally identifiable information or illustrative images. The authors also decided not to put the cleaned and collated dataset in the public domain to avoid aiding potentially undesirable secondary uses of the data.
Findings
The paper audited the face detection, gender classification, and age estimation functions of the four selected facial processing tools. Microsoft reported the highest detection error rate of 3.17% for face detection, implying an inability to detect over a thousand faces in the dataset correctly. This figure was similarly high for the Indian company, FaceX.
All the tools reported higher errors in the gender classification of females compared to males and a complete inability to classify persons of the third gender correctly. The highest female error rate was 14.68% in the case of China-based Face++. This was observed as much higher than the classification errors of the same tool for females of other nationalities in previous studies using a similar methodology.
Age classification errors were also high. Despite considering an acceptable error margin of plus or minus 10 years from a person’s actual age, age prediction failures are in the range of 14.3% to 42.2%.
Between the lines
The paper presents an empirical evaluation of the performance of facial processing tools on Indian faces. Its main findings relate to the demographic group and tool-specific gaps in the performance of such systems and the lack of transferability of findings from audits in different international contexts.
Further work on this subject could examine how facial processing interacts with other axes of diversity in Indian society, such as caste, tribe, religion, and skin tones, and the intersection of these multiple factors. Another direction could be to expand the scope of review beyond face detection and analysis also to include face recognition systems that carry out verification and identification functions.
Lastly, the paper emphasizes that accuracy is a necessary but not sufficient condition for the use of facial processing, which has to be bounded within a legal, fair, and accountable framework.