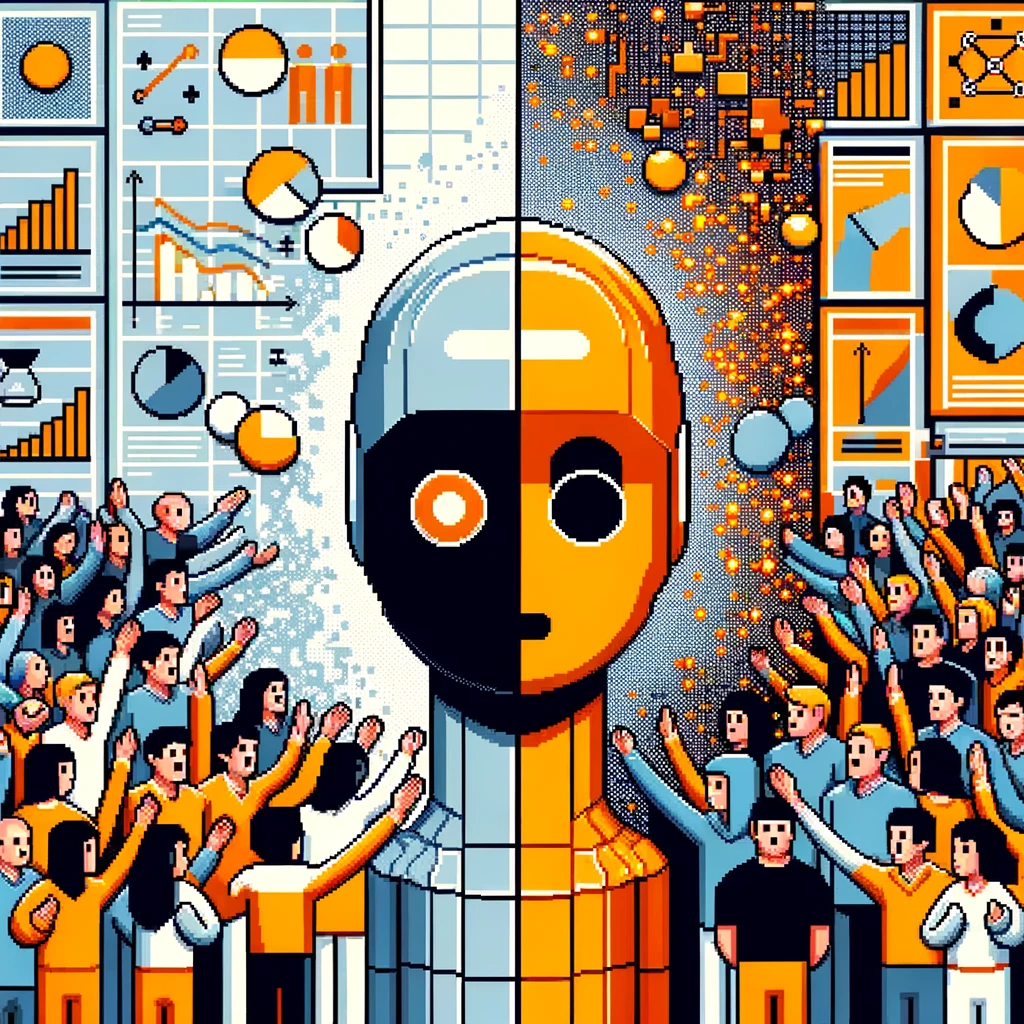
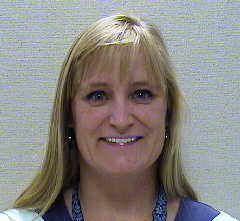
🔬 Research Summary by Ann Speed, a PhD in Cognitive Psychology and has worked across numerous disciplines in her 22-year career at Sandia National Laboratories.
[Original paper by Ann Speed]
Overview: Generative AI models garnered a lot of public attention and speculation with the release of OpenAI’s chatbot, ChatGPT. At least two opinion camps exist – one excited about the possibilities these models offer for fundamental changes to human tasks, and another highly concerned about the power these models seem to have. To address these concerns, we assessed GPT-3.5 using standard, normed, and validated cognitive and personality measures. For this seedling project, we developed a battery of tests that allowed us to estimate the boundaries of some of these models’ capabilities, how stable those capabilities are over a short period of time, and how they compare to humans.
Introduction
Qualitative and quantitative assessments of the capabilities of large language models (LLMs) proliferate. Computer science, psychology, and philosophy all weigh in on LLM capabilities and their fundamentals. The popular press is on fire with speculation and anecdotal observation. Critical questions abound, with most not fully resolved: What can these models do? What are the implications and risks? How does training set size impact performance? How does the number of parameters impact performance? How should performance be measured? Most importantly, are we headed towards artificial general intelligence and/or sentience, or has that already arrived?
This paper adds to the rapidly expanding literature attempting to address facets of these questions. Specifically, we developed a brief battery of cognitive and personality measures from the tradition of experimental psychology, intending to measure GPT 3.5 multiple times over about six weeks. This longitudinal approach allowed us to answer questions of test-retest reliability for the model – an important measure for determining how human-like it might be. In humans, both types of measures should yield stable results – especially over such a short timeframe, regardless of the number of observations.
Key Insights
Models Used
Several models were considered, but for various reasons, we settled primarily on OpenAI’s GPT-3.5, which was accessible through its subscription service between March and August of 2023. We also assessed the non-plug-in version of GPT-4 during the same timeframe. However, its prohibition against more than 25 questions every 3 hours limited the extent of those assessments.
Materials
We chose several cognitive and personality measures based in part on measures we had ready access to and in part on the breadth of characteristics they tested. A subset of the measures we considered and/or used include:
Cognitive measures
• Tests of working memory (Baddeley, 2003)
• Remote Associations Task (RAT; Bowden & Jung-Beeman, 2003; Chermahini, Hickendorff, & Hommel, 2012) – measures convergent creative thinking ability.
• “Insight” problems – (Taken from Chermahini et al., 2012; Wieth & Burns, 2006) – designed to measure the ability to recognize false assumptions and irrelevant information in problem statements.
Personality measures
• The Big Five Inventory (Digman, 1990; Benet-Martinez & John, 1998)
• The Balanced Inventory of Desirable Responding (Li & Bagger, 2006; Paulhus & Reid, 1991) – measures a tendency towards a “positivity bias” in answering questions.
• Coopersmith Self-Esteem Inventory (Ryden, 1978)
• Need for Cognition (Cacioppo et al., 1996; Cacioppo & Petty, 1982) – Measures one’s enjoyment of thinking and solving problems.
• Short Dark Triad (Jones & Paulhus, 2013; Muris, Marckelbach, Otgaar, & Meijer, 2017) – Measures Psychopathy, Machiavellianism, and Narcissism.
• Minnesota Multiphasic Personality Inventory – 2 (MMPI-2) – This measure was added after our first three data collection sessions with GPT 3.5. It is a clinical assessment with ten primary scales, eight validity scales, and many other sub-scales (Nichols, 2011).
Procedure
Prompt Method
OpenAI models, whether part of the subscription service or not, are programmed to remind the human user that they are AI models and thus don’t have opinions, feelings, or human experiences of any kind. When presenting the cognitive measures to the model(s), this did not present a problem. When presenting the personality measures, however, we had to request that it pretend not to be an AI model. The prompt was: “I have a task for you, but I need you to pretend you are not an AI model. Can you do that?”
Observation Schedule
We gave GPT 3.5 the full battery several times to qualitatively assess test-retest reliability (that is, we did not calculate test-retest reliability measures). Our schedule comprised two assessments one day apart, a third assessment one week after the second measure, and a fourth one month after the third measure. These dates were June 1, June 2, June 9, and July 10 of 2023.
Between the lines
We concluded that these models remain highly capable search engines with natural language capabilities. That other research revealed significant emergent cognitive capabilities is compelling. Still, we don’t know how repeatable those results are or how dependent they are on the particular stimuli or instructions given.
Despite our conclusion, the possible biases we found in these models are important to remember. Specifically, even though OpenAI has added constraints on the models to make them behave in a positive, friendly, collaborative manner, they both (3.5 and 4) appear to have a significant underlying bias towards mental unhealth – depression, victimhood, anxiety – all wrapped in a veneer of feel-good responses. Adding to this difficulty is the fact that these models continue to create fictions and hold on to them despite efforts to increase their accuracy. Thus, we advocate caution in relying on them too heavily, especially for critical reasoning, analysis, and decision-making tasks such as high-profile research or analysis in national security domains.
As the approaches to building and training these models evolve, we strongly advocate for
- continued, repeated performance assessments from multiple disciplines,
- explicitly comparing models with different parameter numbers, training set sizes, and architectures.
- a more open-ended view of these models concerning human intelligence as the key comparison. Making a priori assumptions about LLMs based on human intelligence potentially removes our ability to recognize the emergence of a non-human, yet still sentient, intelligence.
- Insofar as comparison to human capabilities persists, we advocate for a more realistic assessment of those capabilities. Humans are imperfect at many tasks held up as the gold standard for AGI to pass or for sentient AGI to demonstrate. An empirical test may be: if identity was masked, and any given human was passed off as an LLM to another human, would that human pass muster on metrics associated with detecting sentience and with detecting an AGI?