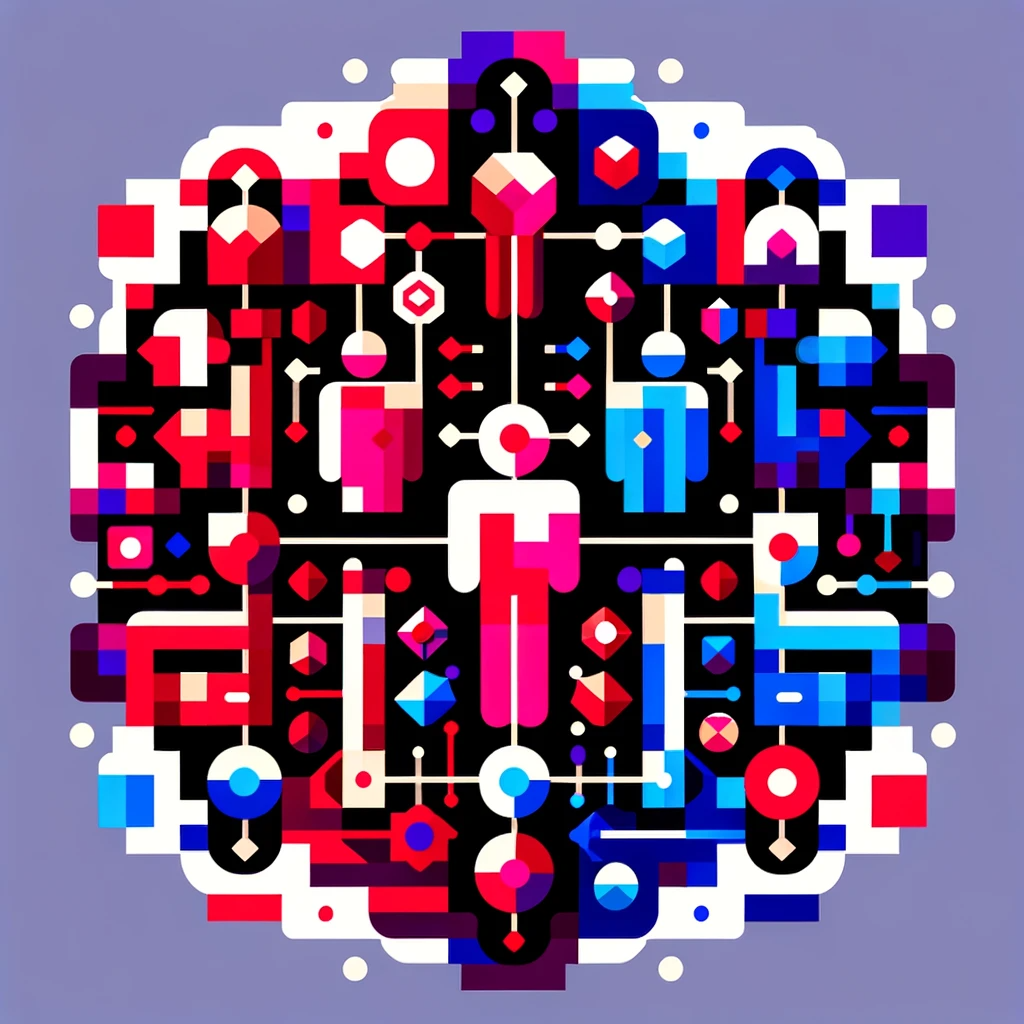
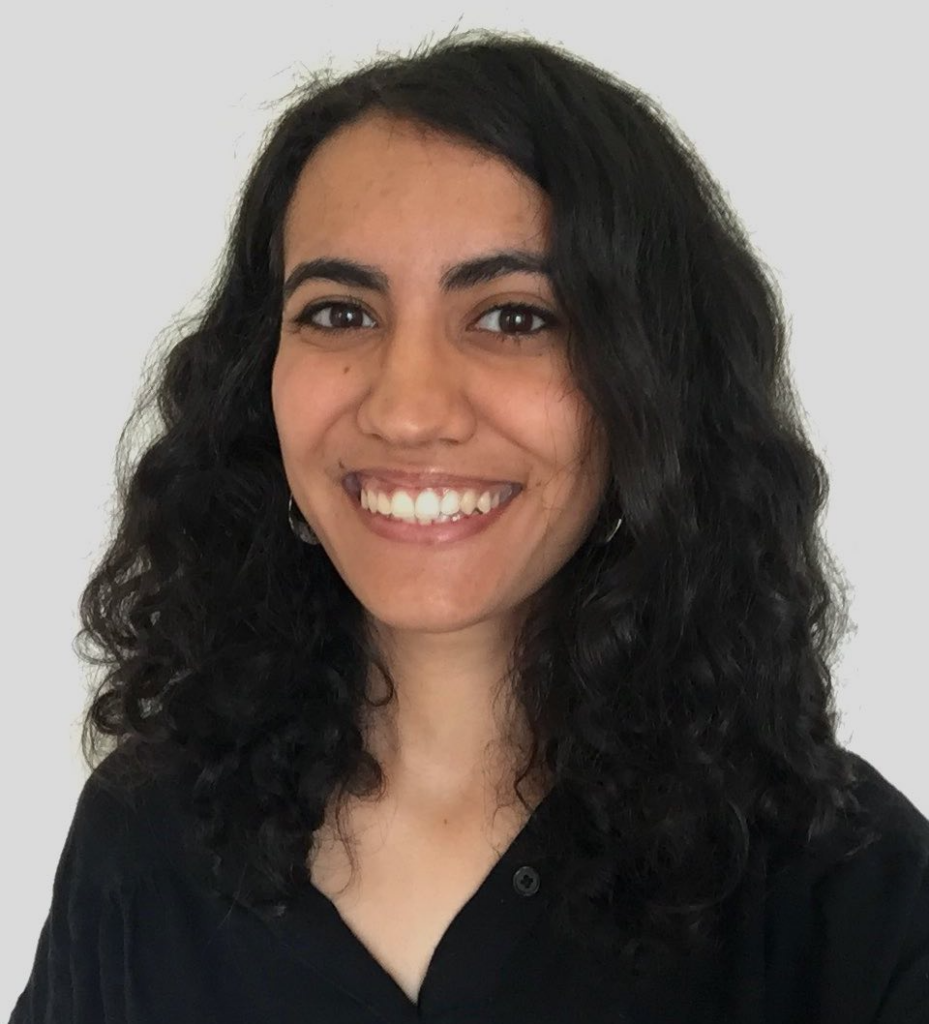
🔬 Research Summary by Amina Abdu, a Ph.D. candidate at the University of Michigan School of Information, where she researches how computational tools shape policy decisions.
[Original paper by Amina A. Abdu, Irene V. Pasquetto, and Abigail Z. Jacobs]
Overview: Technologies can exacerbate racial discrimination, but detecting that discrimination depends on how racial categories are defined—a fraught task in and of itself. This paper uses qualitative content analysis to understand how algorithmic fairness researchers conceptualize racial categories and how they justify their decisions, revealing the values embedded within these decisions. This work sheds light on the unique role of the algorithmic fairness community in driving racial classification beyond traditional race-making institutions like the state.
Introduction
Anti-discrimination efforts, including those undertaken by the algorithmic fairness community, require some notion of what groups are discriminated against. However, defining those groups requires care, in particular, because racial classification has historically been weaponized toward a variety of goals, from restricting immigration to manipulating voting access. While there are numerous historical examples, these dangers are not only in the past: the state of Louisiana, for example, recently undertook an effort to manipulate racial classification to disenfranchise Black voters by reclassifying multiracial Black residents as non-Black. Racial classification has primarily been a state project in the past, but we show how algorithmic fairness researchers and practitioners have started to participate in racial classification.
This study uses qualitative content analysis to characterize racial classification practices in the algorithmic fairness literature empirically. In particular, we identify the racial categories that algorithmic fairness researchers use and how they justify using them. We find that there is significant variation in how researchers conceptualize race and in which racial categories they use. Moreover, we find that these decisions are rarely justified. We then examine the values associated with how race is conceptualized in algorithmic fairness research. We find that while legal conceptualizations of race persist, reflecting the persistent power of state classification practices, values from computer science are transforming racial classification practices. Finally, we propose interventions to make these values visible as the algorithmic fairness community is increasingly important in shaping racial classification.
Key Insights
Our analysis focuses on papers about race and fairness published at the ACM Conference on Fairness, Accountability, and Transparency (FAccT), a prominent conference within the algorithmic fairness community. We perform a manual content analysis of papers published between 2018 and 2020 to understand how the community’s racial classification norms emerged during the initial years of the conference. Our resulting sample consists of 60 papers.
Variation in Race Categories
There needs to be more consistency in adopting racial categories within the algorithmic fairness literature. We find no evidence that algorithmic fairness researchers defer to common norms or institutional standards for defining race (for example, U.S. Census categories). The categories used vary from paper to paper, sometimes even within a single paper. Rather than deferring to historically influential standards, current norms within the algorithmic fairness community grant researchers substantial flexibility in how they decide to operationalize race. We identify 14 distinct racial classification schemas used, which fall into five broad categories: Black/white, white/non-white, Black/non-Black, more than two races, and skin tone. The most common of these, used in 11 papers, is a binary classification schema that distinguishes between white and Black or closely related categories like Caucasian and African-American. Thus, while there is no consensus or standard view of the full set of racial categories relevant in algorithmic fairness research, there is widespread agreement about the importance of the Black and white categories.
The inconsistency in how researchers construct racial categories reflects deeper inconsistencies in how the algorithmic fairness community broadly understands race. Race is conceptualized in several ways across papers. For example, prior work suggests that algorithmic fairness researchers often conceptualize racial groups as “protected classes,” emphasizing boundaries between groups regarding legal protection. While this was the most common way of describing race in the literature, the legal conceptualization of race appeared in fewer than half of the annotated papers. Other papers conceptualized race, for example, as a status category, a set of socially relevant categories, or a marker of observable differences. Underpinning these inconsistencies are divergent views of what types of categories are relevant for analysis.
A Lack of Justification
Beyond wide variation and a lack of consensus, we find that researchers typically do not justify their choice of race categories. Only about a fifth of papers provide any reasoning behind their chosen classification schema. Although most papers in our sample do not justify their choices, there is something to learn from those that do. We find that researchers may justify binary classification schemas by appealing to technical considerations, like computational efficiency or algorithm constraints. Researchers also justify their decisions by appealing to data availability and quality, demonstrating the influence of data collectors. Some justifications center on the contextual relevance of a chosen schema, for example, its relevance to the problem being studied or the social context. These appeals to context highlight the fact that variation in racial classification decisions is not necessarily a problem. Different choices of race categories may reflect important differences in the social groups that are relevant to understanding and intervening in discrimination. Finally, we find that notions of scientific rigor and appeals to prior work are used to justify decisions. This reveals how norms and decisions might become naturalized within the algorithmic fairness community over time and points us toward the importance of attending to these norms.
Values in Classification
We find that many notions of race are used to encode particular values, revealing the value-laden nature of racial classification within academic scholarship. Consequently, illuminating hidden assumptions about the nature of race can help surface the values embedded in algorithmic fairness research. Our analysis reveals that while the algorithmic fairness community has attempted to orient itself around ethical principles, it is also shaped by disciplinary values from computer science, like performance and generalizability. Race is frequently abstracted within algorithmic fairness frameworks so that these frameworks can be generalized to other settings. For example, the notion of race as an abstract “sensitive attribute” rarely appears in papers that emphasize justice but are often associated with papers that express performance-related values.
Between the lines
The algorithmic fairness community has emerged as an important site of racial classification. Our findings show that, within the algorithmic fairness literature, racial categories are adopted inconsistently and with little expectation of justification. Yet, decisions about racial classification are value-laden and have material consequences for allocating social, economic, and political resources. The history of state race-making and racial statistics outlined reveals that racial categories are susceptible to manipulation and have been weaponized to advance state goals.
While we find evidence that legal frameworks persist within algorithmic fairness research, we also see that values from computer science are reshaping racial classification in meaningful ways. As the work of categorization falls into the hands of algorithmic fairness practitioners, care must be given to ensure that both old and new avenues for manipulation are addressed. Interventions should go beyond the individual level. Instead, we propose institutional reforms that center visibility practices and careful operationalizations of race. By highlighting the role of values and institutional factors in shaping racial categories, we hope that this work can enable the algorithmic fairness community to re-examine its practices around racial classification to align the field’s interventions with its values.