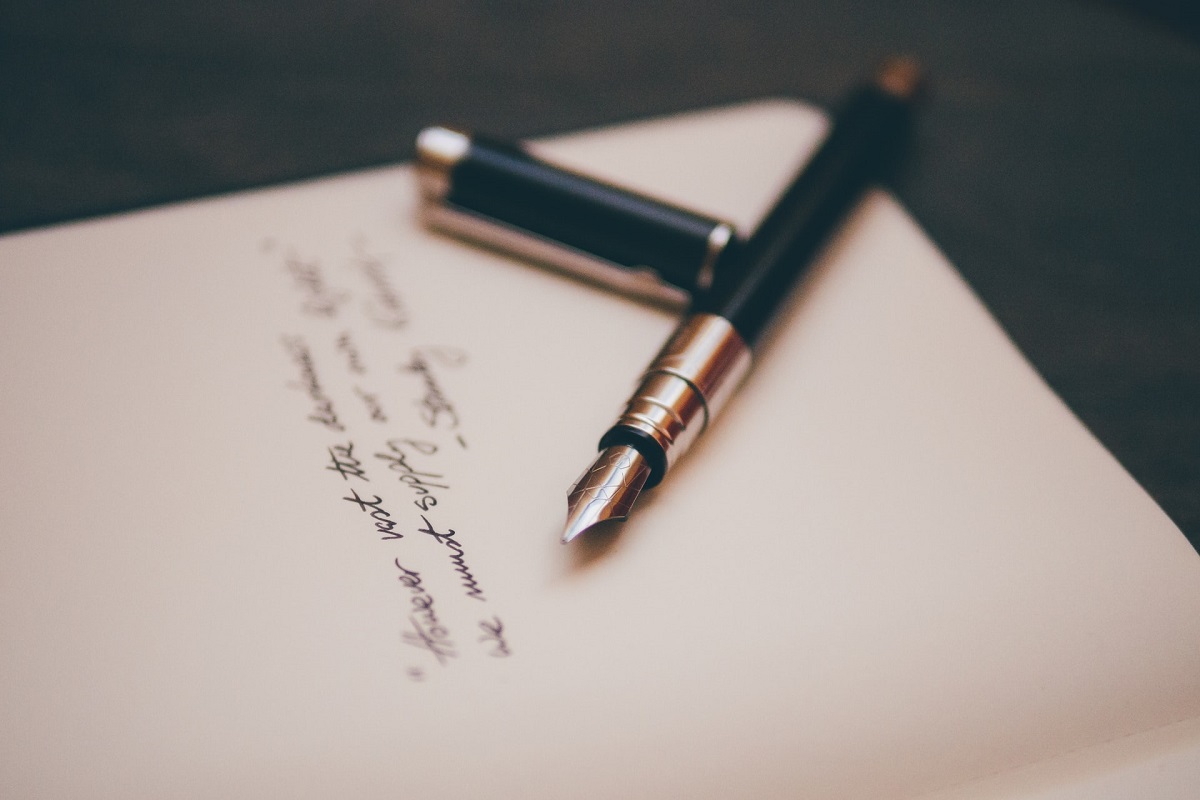
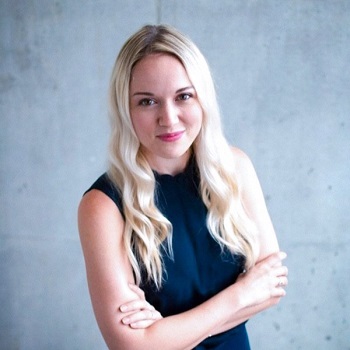
🔬 Research summary by Victoria Heath (@victoria_heath7), our Associate Director of Governance & Strategy.
[Original paper by Nils Kobis, Luca D. Mossink]
Overview: Can we tell the difference between a machine-generated poem and a human-written one? Do we prefer one over the other? Researchers Nils Kobis and Luca D. Mossink examine these questions through two studies observing human behavioural reactions to a natural language generation algorithm, OpenAI’s Generative Pre-Training (GPT-2).
Introduction
Science tells us that the essence of nature is empathy.
Who wrote that? An algorithm or a human being? *Spoiler alert* That philosophical sentence was generated by an open-source algorithm called the New Age Bullshit Generator, a rudimentary example of a text or natural language generation algorithm (NLG). From autocompleting our emails to creating news stories, NGLs are increasingly present. Not only can these systems be used to create new forms of plagiarism but they can also be used to create and disseminate mis/disinformation. Therefore, our ability to decipher what is created by an algorithm and what isn’t is important.
While previous studies have tested the capabilities of algorithms to generate creative outputs, there is a gap in research observing people’s reactions to these outputs. Researchers Nils Kobis and Luca D. Mossink sought to fill that gap by using poetry generated by OpenAI’s advanced NLG, the Generative Pre-Training (GPT-2) model, to measure and analyze:
1) People’s ability to distinguish between artificial and human text
2) People’s confidence levels in regards to algorithmic detection
3) People’s aversion to or appreciation for artificial creativity
4) How keeping humans “in-the-loop” affects points 1-3
Studies 1 and 2: General methodology
Before diving into the results of Kobis and Mossink’s research, let’s take a quick look at their methodology. They created two different studies, Study 1 and Study 2, that each had four parts:
- Part 1 entailed “creating pairs of human-AI poems.” In Study 1, these were written by participants in an incentivized creative-writing task. In Study 2, they used professionally written poems. In Study 1, the researchers selected which creative outputs would be used for judging, something they refer to as keeping a human in the loop (HITL) while in Study 2, they tested HITL as well as what would happen if the poetry was randomly sampled (HOTL). In both studies, they used GPT-2 to generate poetry from an algorithm.
- Part 2 entailed “a judgement task” modelled after the Turing test, in which a judge tries to decipher between two participants which is the machine and which is the human. In Kobis and Mossink’s studies, participants acted as “third party judges” tasked with indicating which creative text they preferred. The researchers also told some participants which poems were written by a human (i.e. transparency) but kept that information hidden from others (i.e. opacity).
- Part 3 entailed a financial incentive to “assess people’s accuracy in identifying algorithm-generated creative text,” an aspect to this study that makes it unique.
- Part 4 entailed the judges indicating “their confidence in identifying the correct poem.” In Study 1, there was no incentive. In Study 2, however, judges received financial incentives for “correctly estimating their performance.”
Studies 1 and 2: Results
In Study 1, judges showed a preference (57%) for human-written poems over the GPT2-generated poems. Contrary to the researchers’ hypothesis, “judges did not reveal a stronger preference for human-written poetry when they were informed about the origin of the poems.” Results also showed that judges were able to accurately distinguish the poems about 50% of the time—indicating that people are “not reliably able to identify human versus algorithmic creative content.” On average, however, the judges were overconfident in their ability to identify the origin of the poems. “These results are the first to indicate that detecting artificial text is not a matter of incentives but ability,” they conclude.
While their findings from Study 1 were generally replicated in Study 2, they observed that when the machine-generated poems were randomly sampled (HOTL) vs selected by humans (HITL) there was a stronger preference for the human-written poems. There was also a notable increase in preference for algorithm-generated poems in the HITL group. Further, they found that people were more accurate in identifying the artificial poetry when the pieces were randomly chosen (HOTL).
Discussion: Should we fear the robot poet?
Nils Kobis and Luca D. Mossink’s research generally affirms what other studies have shown: people generally have an “aversion” to algorithms, especially algorithmically generated content that people perceive as “emotional” rather than “mechanical.” This could indicate that certain creative professions, like journalism, are more likely to be disrupted by AI in comparison to others, like poetry or music. Another significant finding of this research is the influence humans can have on perceptions of artificial content. “We provide some of the first behavioural insights into people’s reactions to different HITL systems,” they explain. This should inform discussions around algorithmic accountability. While keeping humans in the loop can help “monitor and adjust the system and its outcomes,” Kobis and Mossink write, it also allows us to “crucially shape the conclusions drawn about the algorithm’s performance.”
Further research is required into humans’ behavioural reactions to NLG algorithms. By using an incentivized version of the Turing Test, they argue, we could learn more about the use of NGL algorithms in other creative domains, such as news or social media. Kobis and Mossink also argue that creating studies comparing HITL and HOTL is necessary to produce “reliable and reproducible findings on the nexus of human and machine behavior.” They conclude the article by pointing out that although algorithms’ ability to mimic human creative text is increasing, “the results do not indicate machines are ‘creative.’” Creativity requires emotion, something machines don’t possess (yet).
Between the lines
When it comes to AI and creativity, there are significant issues we must contend with and questions we must ask. Like, how do AI-generated creative outputs fit within the realm of copyright and intellectual property law? Should the developer of an algorithm own the copyright of its creative outputs? Creative Commons (CC), the nonprofit organization behind the open CC licenses, says there isn’t a straightforward answer. “It brings together technical, legal, and philosophical questions regarding “creativity,” and whether machines can be considered “authors” that produce “original” works,” wrote CC’s Director of Policy Brigitte Vezina in 2020. Pending further research and debate, they argue, any outputs by an algorithm should be in the public domain.
While this research affirms that humans aren’t fond of artificial text, it also indicates that we can’t really tell the difference—something that will only increase as these systems become more sophisticated. Therefore, it’s important that we prepare ourselves for a future in which these systems impact creative industries, both positively and negatively. Personally, I dread the day I’ll be replaced by an algorithm that can more efficiently (and affordably) spit out witty Tweets and attention-grabbing headlines. Thankfully, that day is not today.