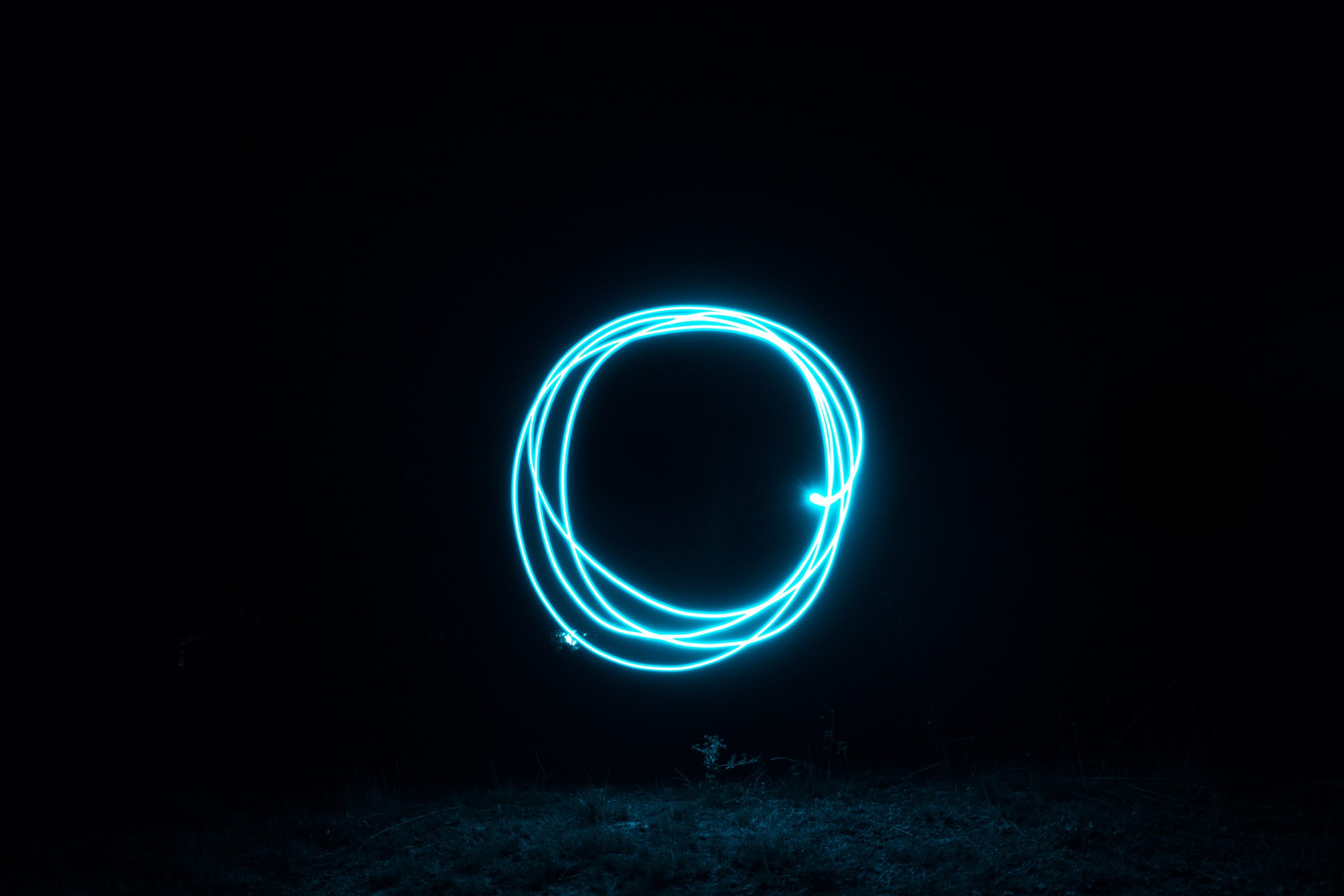
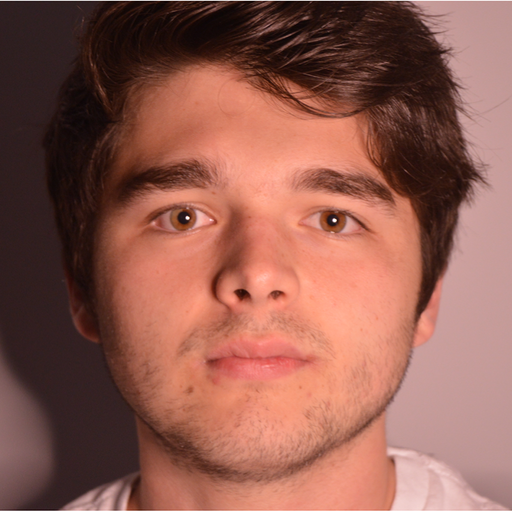
đŹ Research summary by Connor Wright, our Partnerships Manager.
[Original paper by Kathy Baxter]
Overview: With ethical AI certainly being a hot topic in the business world, how can this be achieved? The Ethical AI Practice Maturity Model sets out 4-steps towards achieving the end goal of an âend-to-end-ethics-by-designâ model. With that in sight, the need for company-wide participation and the passion for building ethical AI are a must.
Introduction
Does your company engage in AI products? Does it have an ethical AI team? If not, how would such a team be established? The Ethical AI Practice Maturity Model aims to answer the latter. Stretching from the inception of an ad hoc review from a group of employees, to having ethical AI awareness coursing through a companyâs veins, it offers us a roadmap. Calling on company-wide engagement alongside a passion for ethical AI, the end goal includes having ethical thresholds necessary for the AI product to pass in order to be launched. The best way to illustrate how this can be achieved is to go through the model itself.
Key Insights
- Ad Hoc
Questioning of the AI process at hand begins to take hold. Certain issues arise and are then brought into question on an ad hoc basis. The question no longer becomes âcan we do this?â, but instead âshould we do this?â. The resultant conversations can prove good fuel for informal talks about the technology, helping to clarify the importance of these problems. Once these issues are known and employees can see them being dealt with, trust can start to be developed between those designing the AI and the wider company.
However, the desired confidence takes time to develop. So, building an ethical AI team that accumulates âsmall winsâ can help consolidate their position in the AI process. Churning out results, big or small, will help create more advocates throughout the business and cultivate pivotal involvement from those at the top.
- Organised and repeatable
Arriving at this stage means executives are now on board, and responsible AI practices are now being rewarded. As a result, the next step lies in convincing internal stakeholders to join the process as well. Demonstrating why getting involved is crucial by explaining the risks involved with AI is a sure way to get more employees to sign up. Moreover, contextualising AI in the company context and within its ethical principles to explain its importance could prove even more gripping.
What executives must not do is simply âethics washingâ company employees. This entails placing broad ethical principles, such as âAI must always do the right thingâ, and sticking it on the companyâs website. Instead, how these company principles that apply to AI will be achieved is paramount for forming a successful ethical AI team.
Hence, the stage also includes the formation of the team itself. Given the different situations the team will face, different expertise will be required. Accordingly, the team should be comprised of diverse skill sets, backgrounds, and understandings. Furthermore, the metrics for evaluation should not be classic ârevenue generationâ and the like, but rather making sure the AI systems are safe and not being penalised when they identify ethical risks.
Given the need to identify these risks, considerations on questions of scale can be helpful. To join the team, what is the base knowledge all employees should have of AI? How would you design formal training to convey this knowledge? Would teams working on AI be able to loop in the ethical AI team? Whatever the answers to these questions are, it needs to be sustained and managed in the long run.
- Managed and Sustainable
The training required for the desired base level of knowledge must only include mandatory elements for all employees if itâs necessary. The companyâs ethical principles ought to be common knowledge, but knowing how to mitigate AI system bias is only relevant for data scientists. Managing what the training allows employees to find is the next important step.
Coming across an AI ethical risk is not to be frowned upon completely. No AI system can be 100% bias-free, so saying what bias there is, how itâs being mitigated, and the potential harms it could cause is the best way to deal with the problem. Any damages that are then caused (which can vary depending on the person) need to have appropriate channels to be brought up. Should your business stretch across different countries, the ethical review must ensure the AI system includes other languages and cultures. Dealing with bias for your American clients will not be the same when approaching your Taiwanese partners.
- Optimised and innovative
The final stage is the one to be most desired to achieve. The ethical AI team is no longer a central hub but rather dispersed throughout the whole company. Products and resources require that ethical debt is resolved to be realised, ensuring an âend-to-end-ethics-by-designâ model. However, this does not mean that striving for perfection is halted. With âpracticeâ being the keyword, ethical AI practice never reaches its conclusion. New innovations bring new techno-ethical issues, requiring even more elaboration from the diverse backgrounds of the ethical AI team. This stage may be the end goal, but the end goal is a refined process, not a product.
Between the lines
In my view, ethical AI practice is both necessary and sufficient to operationalise principles like transparency, fairness and equality. Subsequently, any ethical review needs to happen early in the design process; otherwise, thereâs no time to make significant changes. Should this not be the case, the rise of âethical debtâ from unethical AI models, though almost invisible during the AI design, will become very tangible in the form of harm to the public. The Ethical AI Practice Maturity Model gives a company a roadmap to follow and harbours the vital point that change must come from all. Bravery is required, and it all starts with that first small win.