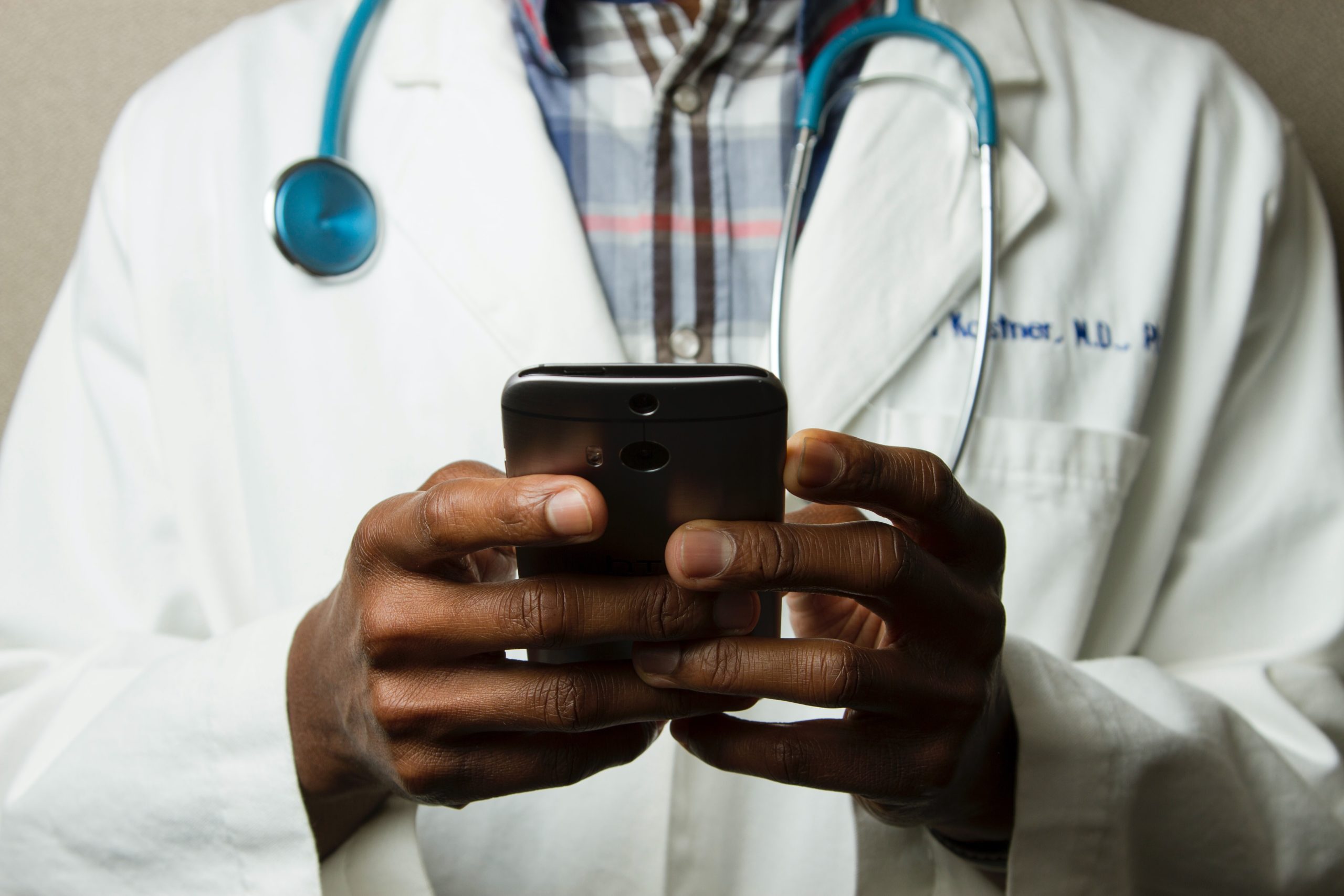
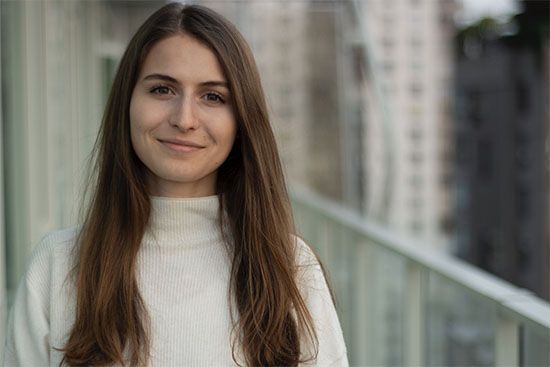
🔬 Research Summary by Paolina Buck, an operations and business development advisor for multiple early-stage startups and is passionate about how we can build a collaborative future between emerging technologies and humanity.
[Original paper by Samantha Lynne Sargent]
Overview: The paper discusses the rise of implicit biases in datasets and algorithms in healthcare as well as suggestions for potential steps to mitigate them. The author introduces the case study of ImpactPro, illustrating how issues such as using the wrong measures to evaluate data, outdated practices in healthcare, beliefs and embedded prejudices, as well as the failure to recognize existing biases, lead to bias in datasets and hence in algorithms. The article also provides suggestions on how to mitigate these biases in the future, concluding that bias in healthcare is not an isolated issue of AI but rather a general problem in healthcare that needs to be solved in order to rebuild trust in the system.
Introduction
Artificial Intelligence (AI) and Machine Learning (ML) were long seen as the potential solution to human flaws in our medical system. They can digest large amounts of data within a short amount of time, find patterns, make predictions and come to conclusions for which humans’ would have taken hours, days or even weeks to discover (if they would have even discovered them). It is undeniable that AI has brought many positive changes, nevertheless, it has also shown its potential to perpetuate biases and flawed/outdated decision-making processes ingrained in existing procedures. In the article “Using ImpactPro as a Case Study for Healthcare Practitioners’ Duties to Engage in Anti-Bias Measures” Sargent investigates how bias in healthcare algorithms can affect populations, how the ecosystem in which algorithms function matters and finding a gold standard for mitigating bias in AI in healthcare. By introducing the ImpactPro study she illustrates how bias can arise by using incorrect proxy measures for complex matters such as health needs and through outdated decision-making practices. To counteract these trends she provides recommendations such as the education of practitioners on implicit bias in their practices and AI outputs, the need to build trust with marginalized groups and diversifying datasets to include underrepresented populations.Â
Key Insights
One fits all?
Sargent points out that even though AI and ML have brought many positive changes to the healthcare field it has also been shown that they have the potential to perpetuate and reproduce biases whether it is through outdated behaviour, stereotyping or the way data is collected. In her paper “bias” is referring to “consciously and unconsciously held negative feelings or opinions towards marginalized groups, rooted in historic discrimination, that influence the way a person behaves and thinks” (Sargent, 2021). It becomes obvious that AI is not a simple “one-fits-all” solution and reflects just how complex decision-making in healthcare is. Acknowledging the vast diversity the applications of AI can have in healthcare, the paper uses the case study of ImpactPro as a specific example of bias against marginalized populations in AI and potential countermeasures.
The ImpactPro(blem)
ImpactPro was deployed to “identify patients with complex health needs who would benefit from greater attention and additional resources”. Unfortunately, another goal of the algorithm besides to “better serve patients” was to “in part” cut costs which led the algorithm to use healthcare costs as a proxy measure for healthcare needs. Even though race or gender was not part of the parameters the algorithm developed a bias against recommending Black patients to the health program as this population group generated different healthcare costs than White patients (e.g. emergency costs vs. surgical and outpatient costs) leading to fewer healthcare spendings. The algorithm was unable to take into account socio-economic factors such as income or the barriers faced in terms of accessing healthcare, and historic factors such as the patients’ trust in the healthcare system.
Hence, it wrongly concluded that Black patients are less sick than White patients based on the amount of money they spend on healthcare. Using this flawed correlation between these two labels is called “labelling bias”, healthcare spending was incorrectly chosen as a proxy measure and unable to represent much more complex medical needs.
Main Issues of the ImpactPro Algorithm:
- Using healthcare costs as a proxy measure for healthcare needs leads to labelling bias
- Lacked the ability to consider socio-economic and historic factors that explain the lower healthcare spending of Black patients
- The algorithm became biased against recommending Black patients for complex needs program, based on lower healthcare spendings
The researchers identified this flaw and created a second algorithm that replaced the label on which the algorithm was operating with a “quantifiable criterion of health”, a common way of fixing “labelling bias”.
Not just an AI issue
Finding a “quantifiable criterion of health” required the study authors to have a deep understanding not only of the technical side but also of the healthcare field to pick the relevant label, showing how intertwined and complex the application of AI in healthcare is. Furthermore, it is important to know that the algorithm did not operate in isolation. Patients who scored in the 97th percentile (according to the ImpactPro algorithm) were automatically identified for enrollment in the health program and patients over the 55th percentile were referred to their primary healthcare provider for further decisions.
When recommended to a healthcare provider they were able to counteract the label bias of the first ImpactPro algorithm due to their access to electronic health records and insurance claims gaining a more holistic picture of the patient’s situation.
Nevertheless, compared to the second version of the ImpactPro algorithm the healthcare providers still showed more bias. Furthermore, healthcare practitioners were not as biased as the first version of the ImpactPro but still showed more bias in their decision making toward Black patients compared to the second version of the ImpactPro algorithm.
Sergeant points out that the practitioners would have had the opportunity to correct the algorithm’s bias without any technical knowledge by approaching its decisions more critically and being “less biased in their own decision making”. This example illustrates the true responsibility of the different stakeholders in the healthcare ecosystem as they have the power to counteract biased outputs provided by algorithms through critical thinking and by acting as “overseers of and collaborators with AI technologies”.
Further sources for Bias in Healthcare:
- Healthcare practitioners appear to be quite susceptible to implicit biases due to reliance on stereotypes in their decision making
- Emphasis on “group-level information” instead of individual-level data
- False belief in strong personal objectivity through “vast knowledge of scientific data”
- Demonstrated implicit and explicit pro-white biases in western countries (the ImpactPro study was focussed on an American context, but there is evidence that for example in Canada First Nations, Inuit, and Metis patients are also facing bias and discrimination when seeking healthcare)
- Additional implicit and explicit biases regarding obesity, gender, age
Going Forward
Based on these observations Sergeant suggests the following best practices to take a step towards the elimination of bias in healthcare. These suggestions also align with “the ethical principles for AI best practices, including those put forward by the Montreal Declaration for the Responsible Development of Artificial Intelligence” and “ HLEG’s AI Guidelines on trustworthy AI”.
Steps to mitigate implicit bias in healthcare practices: | Steps to mitigate implicit bias in AI in healthcare: |
Educate physicians regarding possible implicit biases in their practices and their susceptibility to them; promote educational projects. Education on how historic data used for diagnosis can be biased and not applicable to minority groups | Based on education regarding potential implicit biases in physicians’ practices, enable recognition of potential bias outputs of algorithms through critical thinking Use opportunity to scrutinize and question individual outputs generated by AI |
“Individuating”: collecting information about a patient that goes beyond social category information “Perspective taking”/Social Intelligence: For example imagining the patient’s emotions to understand their needs better | Apply “individuating” and “perspective-taking” when evaluating an algorithm’s output, looking closely at a patient’s individual history. Apply social intelligence, such as recognizing emotions to bridge algorithm’s inability of viewing different perspectives |
Increase diversity in the healthcare provider/practitioner population | Increase diversity in the healthcare field, in the AI field and who is included in datasets that are used to deploy AI |
If suspicions arise that an algorithm might be biased, software can be investigated by groups such as the Algorithmic Justice League. Checks like these should be routine and part of a continuous monitoring process |
Sergeant acknowledges that there are still crucial issues that need to be addressed, such as how to increase the quality of health data overall while respecting the patients’ privacy. She states that her proposal is aimed at frontline AI users who are also in direct contact with patients and that these larger regulatory and improvement questions are beyond the scope of this paper. Nevertheless, she notes that it is possible that by increasing bias awareness among frontline healthcare workers (with the ability to diagnose and refer patients) trust in the health care system could be rebuilt also for minority groups which consequently would lead to “better and more inclusive data sets” used for healthcare algorithms.
Between the lines
The paper concludes that it is crucial to understand that AI is not “the solution to our […] human faults” but rather a reflection of past actions and practices. Sergeant emphasizes that issues like bias are not a new “AI problem” and are deeply embedded in the healthcare ecosystem and “ exist without the use of and reliance on AI technologies”. These technological and behavioural challenges are part “of an existing duty to promote equity and reduce bias in all aspects of care” to rebuild the trust in our healthcare system and give especially vulnerable patient groups better treatment and quality of life.