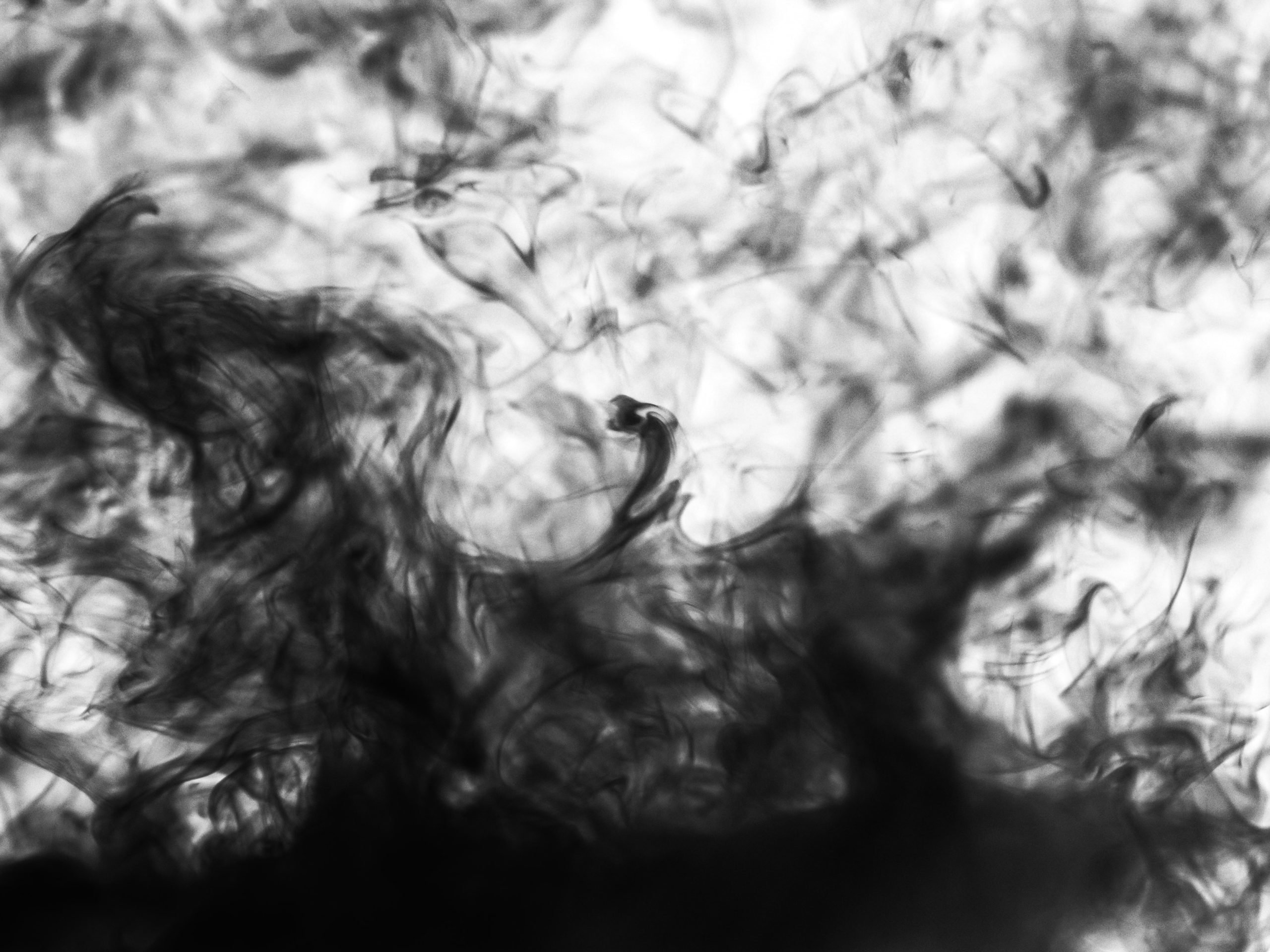
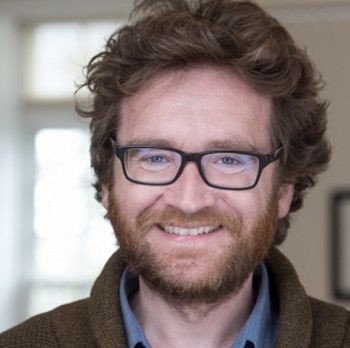
🔬 Research summary by Dr. Andrea Pedeferri, instructional designer and leader in higher ed (Faculty at Union College), and founder at Logica, helping learners become more efficient thinkers.
[Original paper by Maya Krishnan]
Overview: “Explainability”, “transparency, “interpretability” … these are all terms that are employed in different ways in the AI ecosystem. Indeed, we often hear that we should make AI more “interpretable” and/or more “explainable”. In contrast, the author of this paper challenges the idea that “interpretability” and the like should be values or requirements for AI. First, it seems that these concepts are not really intuitively clear and technically implementable. Second, they are mostly proposed not as values in themselves but as means to reach some other valuable goals (e.g. fairness, respect for users’ privacy). And so the author argues that rather than directing our attention to “interpretability” or “explainability” per se, we should focus on the ethical and epistemic goals we set for AI while also making sure we can adopt a variety of solutions and tools to reach those goals.
Introduction
As we mentioned in a previous summary, back box’s opaqueness poses both epistemic (are the algorithms in fact reliable?) and ethical (are the algorithms ethical?) challenges. Relatedly, they also seem to violate people’s claim-right to know why a certain algorithm has produced some predictions or automated decisions that concern them. For many the epistemic and ethical risks back box’s opaqueness poses could be mitigated by making sure that AI is somehow interpretable, explainable and/or (as some say) transparent. Contrary to the received view on this issue, the author of this paper challenges the idea that there is a black box problem and denies that “interpretability”, “explainability” or “transparency” should be values or requirements for AI (see also here).
Key Insights
The author of this paper challenges the idea that there is a black box problem and that “interpretability”, “explainability” or “transparency” should be criteria for evaluating an AI system.
The first problem the author points out is that the terms above (“explainability”, “transparency, “interpretability”) are often unclear and poorly defined. Let’s take “interpretability”: AI is interpretable when, roughly, it is understandable to consumers. The author notices that this definition is not really helpful: it does not clarify what “understandable” means and does not offer any insight on what the term could mean when applied to algorithms. Also, there is confusion on what should be understandable. Here are a few candidates: the prediction of the algorithm itself, the inner workings of the AI that produced its prediction, or the reasons why / the justification for the algorithm making that prediction. Which one is key for human understanding?
Many seem to believe that explaining how an algorithm reached a certain outcome is tantamount to making AI understandable. However, it should be noted that causal explanations are not the same as justifications. That is, the reason or justification for a given outcome might not clearly map into the causal path that brought the algorithm to that conclusion. As the author puts it, “[t]his point is particularly apparent in the case of neural networks. The causal process by which some input triggers a certain pathway within the network does not straightforwardly map on to justificatory considerations.” Indeed it would be like asking “a person why they have given an answer to a particular question and they respond with an account of how their neurons are firing”. The causal story is not a rational explanation per se. Thus, if the explanation we look for tells us only about the causal path that gets the algorithm to a certain conclusion, this story would not provide the right level of explanation needed to rationally understand that very outcome.
Finally, the author notices that interpretability, explainability and the like are a means to an end, i.e. ensuring that AI is ethical and trustworthy. The author recommends that we focus on those goals instead of treating interpretability and the like as they were ends in themselves. Since there might be other ways to reach those goals, it seems unhelpful to focus just on one set of solutions.
Between the lines
The paper rightly points out that we need a more coherent and precise analysis of concepts such as interpretability and explainability. And the author also clarifies that “[w]hile this paper questions both the importance and the coherence of interpretability and cognates, it does not make a decisive case for the abandonment of the concepts.” We agree with this too, as we appreciate the importance of ensuring explainability in AI as a way to both assess whether algorithms are ethical, robust and reliable, and to protect people’s right to know and understand how assessments are made. To do so, however, we first need to make sure that we agree on what we mean by ‘explanation’ and on what kind of explanation is needed for real human understanding.