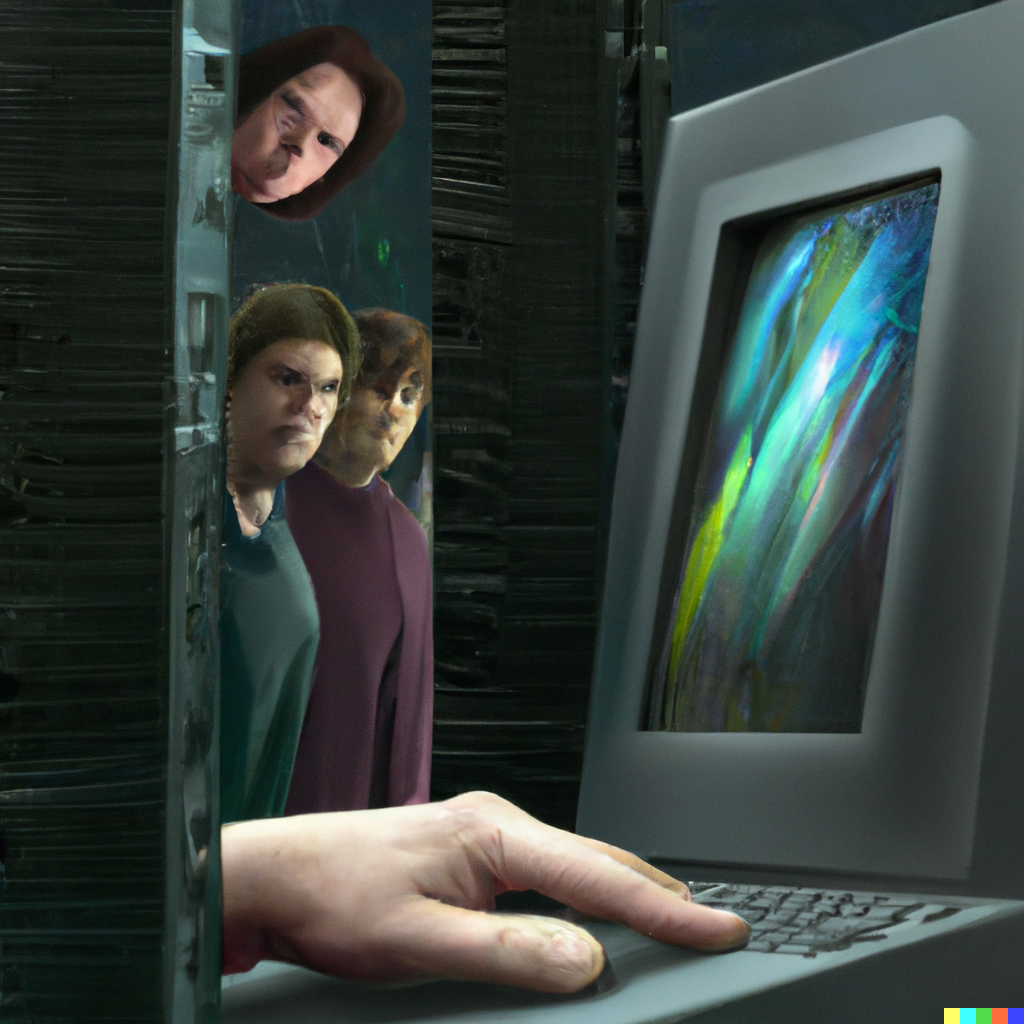
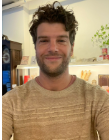
🔬 Research Summary by Dr. Henry Fraser, a Research Fellow in Law, Accountability, and Data Science at the Centre of Excellence for Automated Decision-Making and Society.
[Original paper by Henry Fraser and José-Miguel Bello y Villarino]
Overview: The European Union’s draft ‘AI Act’ aims to promote “trustworthy” AI with a proportionate regulatory burden. The final text of the Act is currently under negotiation between the European Commission, the European Parliament, and the Council of the European. This paper critically evaluates competing approaches to risk acceptability that are up for negotiation, explaining why any obligation to render risks from AI systems “acceptable” must be qualified by considering what is reasonable in all the circumstances.
Introduction
You are the developer of an AI system that will evaluate University applications throughout Europe. Under Article 9 of Europe’s draft AI Act, which may become law as early as 2024, you have an obligation to implement risk management because the system is “high-risk”. Risk management must ensure that any remaining risks are “acceptable.” What does that even mean? How do you decide when risks from high-risk AI systems (with potential impacts on safety, rights, health, or the environment) are acceptable?
The final text of the Act (actually called a Proposal for a Regulation Laying Down Harmonised Rules on Artificial Intelligence) is currently under negotiation between the three main branches of the European government: the Commission, the Council, and the Parliament. Among many other thorny issues for negotiation, negotiators choose between two competing approaches to risk acceptability. One approach, proposed by the European Commission, would require risks to be reduced “as far as possible” (AFAP) in design and development, with remaining risks subject to further mitigation. The Parliament, by contrast, proposes to introduce considerations of what is “reasonably” acceptable.
This paper critically evaluates the two approaches, exploring how AFAP has been interpreted in other contexts and drawing on negligence and other laws to understand what “reasonably acceptable” risks might mean. It finds that the Parliament’s approach is more compatible with the AI Act’s overarching goals of promoting trustworthy AI with a proportionate regulatory burden.
Key Insights
Why does risk acceptability matter?
Trustworthiness and proportionate regulatory burden are the AI Act’s two main goals. Because there are so many issues under consideration in negotiations about the Act – from the definition of AI to the responsibilities of foundation model developers – the approach to risk acceptability has mostly flown under the radar. That belies its importance. The rules about when risks are acceptable and when they do not determine how “trustworthy” AI systems really are and how much burden the AI Act will place on AI development.
It’s a bad idea to require AI risks to be reduced or eliminated “as far as possible.”
A requirement to reduce risks as far as possible, which the Commission’s version of the Act contemplates, is exacting if taken literally. AI outputs are known to be “emergent” (unpredictable), and it is always possible to implement just one more measure to reduce risk. Our research shows that the European Commission has historically taken a very narrow approach to the AFAP risk criterion in the context of medical devices. The Commission went so far as to require a change to the ISO standard for medical device risk management, stating that in Europe, medical device risks had to be reduced as far as possible “without there being room for economic considerations.” Our survey of industry responses to this change indicated that such a narrow risk acceptability criterion created uncertainty on where to draw the line for risk management. It seemed to encourage businesses to conceal their cost-benefit analysis around risk management rather than disregard economic considerations. The same problems are likely to arise in the AI context.
It makes sense to factor in the costs and benefits of risk management when judging the acceptability of AI risks
The Parliament’s proposed approach to risk management for high-risk AI would introduce considerations of reasonableness, proportionality, and the impact of risk management on the potential benefits of the AI system into risk acceptability judgments. Drawing lessons from negligence law (par excellence about when risks are unacceptable) and medical device regulation, our paper explains how principles of reasonableness could allow AI developers to make more principled risk acceptability judgments. It would allow them to factor in various kinds of cost-benefit and risk-benefit analyses, including whether the cost of a given risk management measure is worth the risk reduction, whether risk management negatively impacts the overall benefit of an AI system, and whether risks are significant enough to warrant expenditure of finite risk management resources.
Between the lines
The choice between the stringent “as far as possible” risk acceptability criterion and the more flexible approach permitted by introducing reasonableness should be informed by the overall architecture of the AI Act and by the issues of public policy that are at stake. The Act contemplates that its requirements, including risk management, will be met through certification against technical standards – mostly self-certification. It also states that risk management should consider the “state of the art,” including as reflected in standards. In effect, this means that technical standards and the state of the art play the role of a pressure valve: once you meet the state of the art, you can say you’ve reduced a risk “as far as possible.”
But why should it fall to technical standards bodies or to the big tech companies whose practices shape the state of the art to decide when risks to fundamental rights from AI are acceptable? It is not clear they have the expertise in human rights or the political legitimacy to exercise this kind of discretion over matters of public policy.
The benefit of a reasonableness approach is that it brings all the trade-offs involved in risk acceptability judgments to the fore. It assumes value-laden judgments. Ultimately, the legitimacy of these judgments will need to be supported by input from stakeholders and affected groups and by guidance from regulators with the requisite expertise and legitimacy.