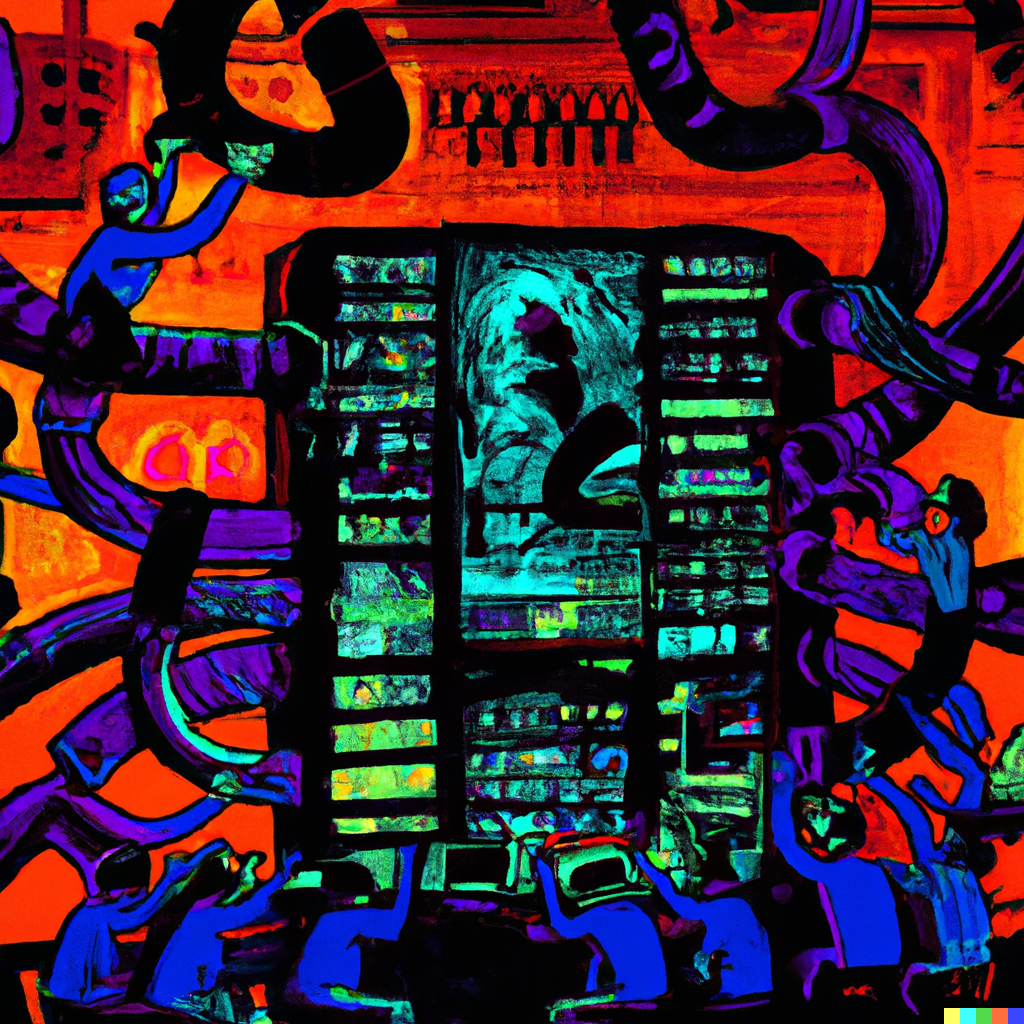
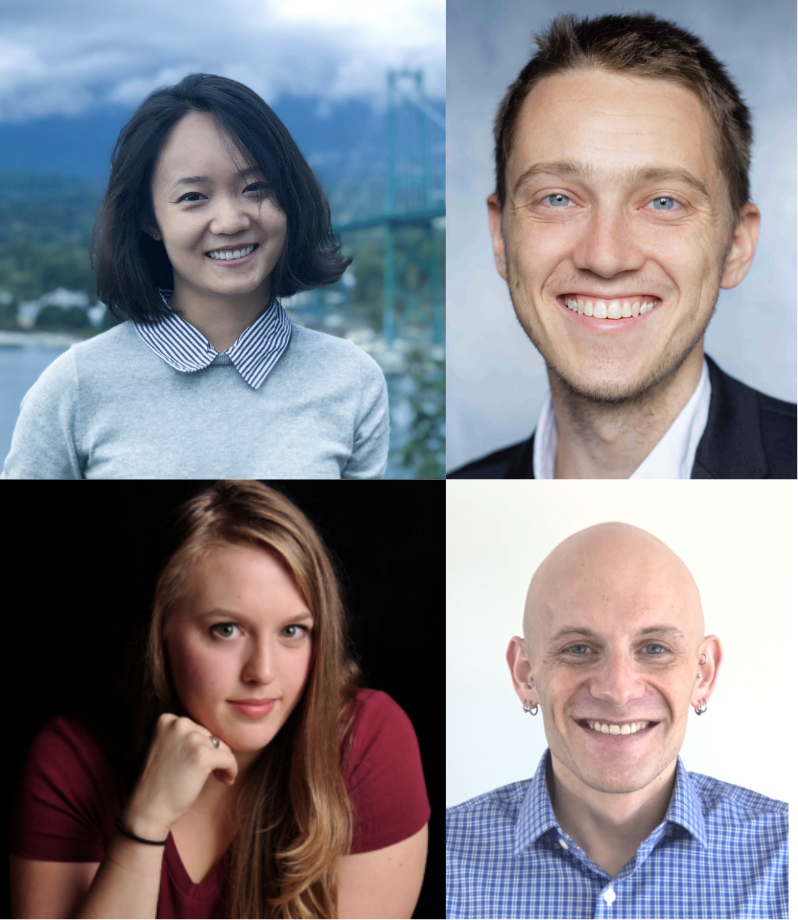
🔬 Research summary by Hanlin Li, Nicholas Vincent, Stevie Chancellor, and Brent Hecht.
Hanlin Li is a postdoc at UC Berkeley and an incoming assistant professor at UT Austin.
Nicholas Vincent is an assistant professor at Simon Fraser University.
Stevie Chancellor is an assistant professor at the University of Minnesota – Twin Cities who studies how to build human-centered AI systems using social media data.
Brent Hecht is an associate professor at Northwestern University.
[Original paper by Hanlin Li, Nicholas Vincent, Stevie Chancellor, and Brent Hecht]
Overview: Over the past few months, the public has learned something about AI that those of us in the field have known for a long time: Hundreds of millions of people around the world effectively have uncompensated side gigs generating content for AI models, often models owned by some of the richest companies in the world. Despite its vital role as “data labor” for these AI models, the public rarely has the ability to shape how these AI systems are used and who profits from them. This paper outlines steps that policymakers, activists, and researchers can take to establish a more labor-friendly data governance regime and, more generally, empower the data producers who make AI possible.
Introduction
With the large-scale deployment of AI systems, it became increasingly clear that hundreds of millions of technology users are supplying free labor to tech companies. ChatGPT, search engines, and other AI systems are possible only because of massive amounts of data generated by people worldwide. From editing Wikipedia to clicking the like button on social media sites to posting a blog, these online activities contribute content or engagement to the hosting platforms and lead to the accumulation of massive datasets that make AI models like ChatGPT and Stable Diffusion possible.
But too often, those who share valuable data and content online rarely know how their contribution will be used, let alone exert any control over the output technology.
In our recent paper, we asked what if we treat data creation as a form of labor? After all, we all act as data workers by contributing content, personal information, and behavioral traces to tech companies. These actions create value for these companies and allow them to profit, sometimes in billions of dollars. So we drew inspiration from research studies on labor, crowdwork, and computing to map out how practitioners, policymakers, advocates, and researchers can empower data laborers.
Here, we highlight three steps practitioners, policymakers, and advocates can take to progress toward a labor-oriented data economy: increasing transparency about data reuse, creating meaningful feedback channels between data producers and companies and sharing data-generated revenue.
Key Insights
What are some examples of data labor?
Data labor is everywhere. Our working definition describes data labor as activities that produce digital records useful for capital generation. When using search engines, our clicks and browsing behaviors help search engine companies improve their click-through rate, attract more users, and generate more advertising revenue. We help Reddit attract more user engagement and advertising by putting user-generated content like Reddit posts online. This content also enables other actors to build their products, like when AI firms use scraped datasets like the Common Crawl corpus to train generative AI models like ChatGPT.
The idea that data creation is a form of labor is not new. In 2018, Arreita-Ibarra and colleagues asked “Should we treat data as labor?”, given the emergence of these highly profitable data-driven technologies. Over the years, our team’s research has explored the value of data for tech companies in the context of recommender systems and social platforms. Our research shows that the labor that moderators put into managing content platforms is worth millions of dollars a year for companies like Reddit. Moreover, if data producers withhold their labor, data strikes can dampen the performance of data-driven technologies, particularly when they target specific technology features.
These are just a few examples of data labor. As web crawlers traverse the internet, any published digital content contributes to AI advances and tech development. Put another way, data scraping is entrapping more and more data producers into the labor force behind data and AI, often without their knowledge.
How does this concept guide action?
We have established that many online activities fall under data labor, but is there any difference between one over the other? Should we treat them differently when thinking about taking action to empower data producers? Drawing inspiration from past research on labor, crowd work, and computing, we constructed six dimensions to characterize data labor:
- Legibility: Do data laborers know their labor is being captured?
- End use-awareness: Do data laborers know how the output data is used?
- Collaboration requirement: Does the production of data labor involves collaboration?
- Openness: Is the resulting data open to the public?
- Replaceability: Is data labor easily replaceable?
- Livelihood overlap: Is data labor part of occupational activities?
The six dimensions of data labor are not intended to be all-encompassing. We aim to facilitate action-oriented conversations and advance discussion on the relationship between data, power, and social inequality. Here, we highlight three general directions informed by these dimensions (our paper has even more recommendations).
Increase transparency about data collection and reuse
Transparency about data collection and reuse is a necessity for data laborers to exert any power over technology companies. When people do not realize that they are performing data labor, this naturally inhibits their power to withhold or change this labor. Poor transparency further limits opportunities for collective action, e.g., a coordinated data strike where people agree to stop using a particular form of technology. Conversely, if one understands the downstream use cases of the data they produce, they may purposefully change their behaviors. For example, a research team at UMN examined Wikidata contributors’ motivations and found that they would be demotivated if their contributions were to be used primarily for profits.
To empower data producers, data collection and reuse transparency should be the first milestone for practitioners, policymakers, and advocates. Practitioners could develop tools highlighting how data contributed on a specific platform (e.g., Wikipedia and ArtStation) will be used by other systems (e.g., Google Search and Stable Diffusion). Policymakers can take a step further and mandate transparency about datasets’ origins, as seen recently in the EU AI Act and China’s proposed regulations on generative AI models.
Create meaningful feedback channels between data producers and companies
Transparency alone is not enough to shift power toward data producers. We must also create meaningful feedback and accountability mechanisms between data producers and companies.
To this end, researchers and advocates have proposed transformative data governance models to grant data producers control over their data output, e.g., data cooperatives, data intermediaries, and data trusts. One consistent theme from these proposals is stewardship, where an entity is responsible for representing data producers’ interests and determining what data use case is permitted. These collective approaches are beneficial because getting individual consent from everyone who contributed to a dataset can be challenging, if not impossible.
In the short term, policymakers can immediately impact by developing direct feedback channels between data producers and companies. Similar to how shareholder meetings are required for publicly traded companies, policymakers may mandate operators of data-driven technologies to have public communication channels with data laborers to solicit their input and feedback.
Share data-generated revenue fairly
Recent advances in generative AI models have brought the extractive nature of these technologies to the attention of content creators. With the monetary value of data increasing rapidly, the time is ripe to discuss how to share data revenue more broadly with data producers. There is an opportunity for practitioners to build new technologies enabling data producers to earn meaningful revenue from institutions like OpenAI. Previously, Shutterstock, a company that plans to sell DALL-E-generated images, announced its plan to compensate artists in response to the artist community’s criticism of the model’s unapproved reuse of publicly available artwork. While the details of the compensation mechanism are unclear, this example shows the possibility for data producers to get “back pay” from companies that monetize and benefit from their labor.
One key challenge to fairly distribute data’s revenue is assessing a fair value exchange between those who create data and those who monetize it. Researchers and policymakers have pondered this question long before the emergence of generative AI models (e.g., data dividends) and developed different models of data valuation. For example, some of our work assessed revenue generated by data and labor hours required for data generation. Such data value measurements will be important to inform the design of public policies and market incentives in the data economy.
Between the lines
The bottom line: control and collective benefits
The waves of protests and strikes by those whose work fuels AI technologies are just another reminder of our decade-long struggle with data governance. Previously, we have seen public outcries to social media platforms, targeted advertising, and data brokers when data producers are kept in the dark about how their data is being used and who it benefits.
While we seek to mitigate many other problems these massive data-driven technologies impose, like fairness and privacy issues, we should not ignore the lack of control and the economic inequity data producers face. To build a future in which data producers collectively shape and benefit from data-driven technologies, we recommend:
- Increasing transparency about data collection and reuse
- Creating meaningful feedback channels between data producers and companies
- Sharing data-generated revenue fairly