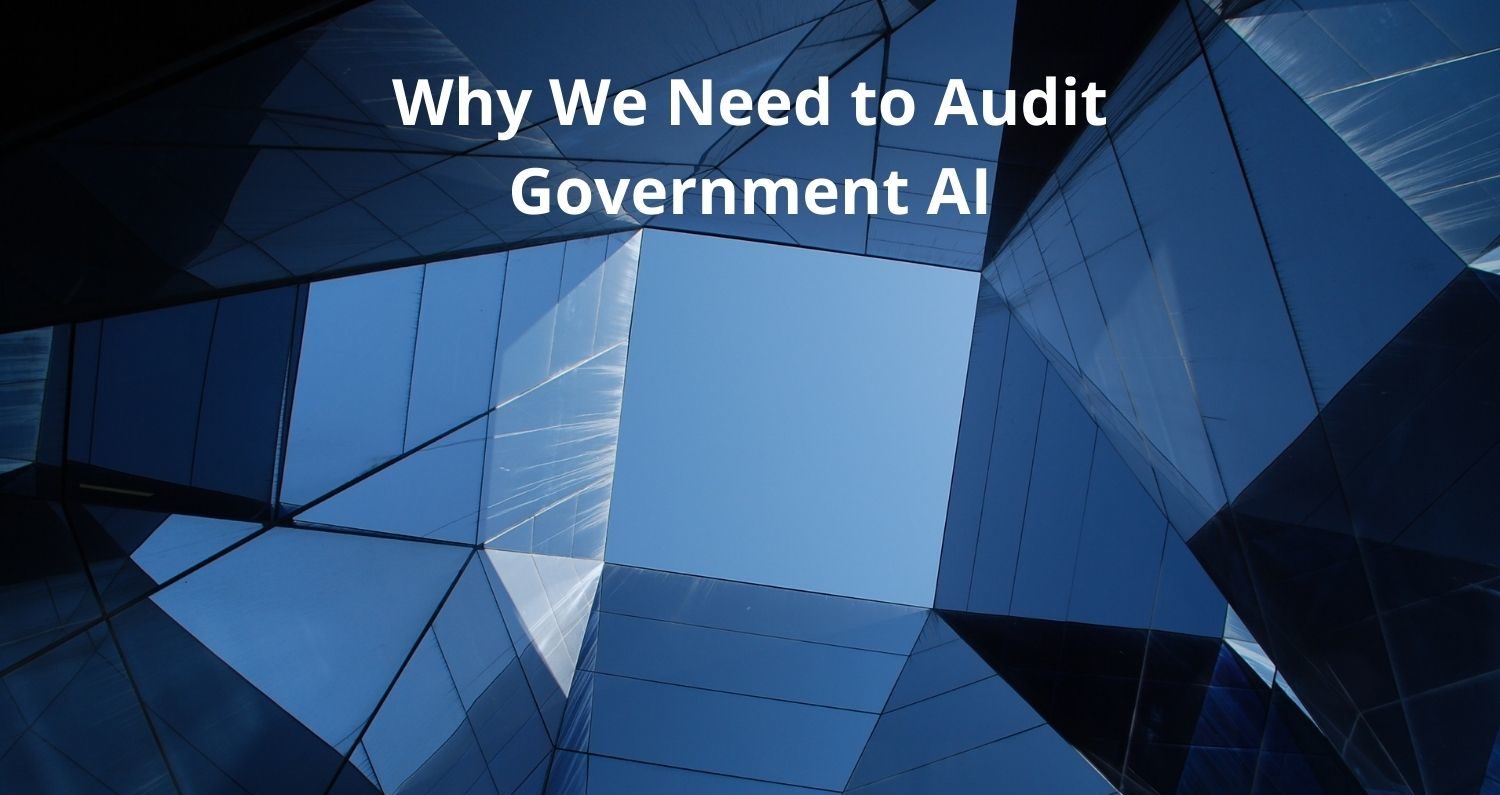
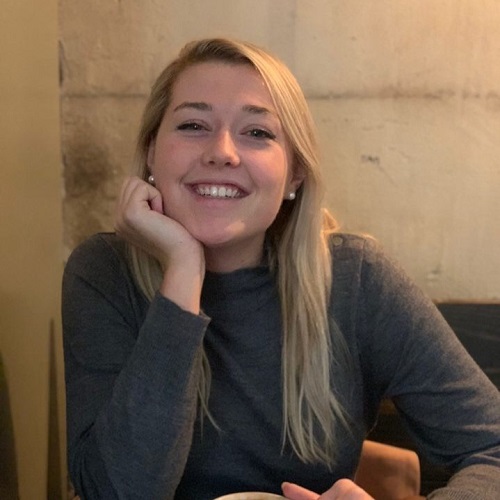
Guest post contributed by Alayna Kennedy, Public Sector Consultant and AI Ethics Researcher at IBM.
Artificial Intelligence (AI) technology has exploded in popularity over the last 10 years, with each wave of technical breakthroughs ushering more and more speculation about the potential impacts of AI on our society, businesses, and governments. First, the Big Data revolution promised to forever change the way we understood analytics, then Deep Learning promised human-level AI performance, and today AI offers huge business returns to investors. AI has long been a buzzword in businesses across the world, but for many government agencies and larger organizations, earlier applications of commercial AI proved to be overhyped and underwhelming. Only now are large-scale organizations, including governments, beginning to implement AI technology at scale, as the technology has moved from the research lab to the office.
Each of the waves of AI development has been accompanied by a suite of ethical concerns and mitigation strategies. Between 2016 and 2019, 74 sets of ethical principles or guidelines for AI were published, focusing on high-level guidance like âcreating transparent AI.â These high-level principles rarely provided concrete guidance, and often werenât necessary, since most large organizations and government agencies were not yet using AI at scale. In recent years, the AI Ethics community has moved past high-level frameworks and begun to focus on statistical bias mitigation. A plethora of toolkits, including IBMâs AIF360, Microsoftâs Fairlearn, and FairML, have emerged to combat bias in datasets and in AI models.
We now find ourselves in a new, less exciting wave of AI adoption â starting to implement AI at scale. Despite the hype of the first waves promising immediate returns, AI is just now starting to be widely applied in businesses that donât have strong technical capabilities of their own, including government agencies.
Governments are now using AI to make decisions within large scale government projects, including the deployment of humanitarian resources, who is granted bail, which citizens are subjected to increased police presence, whether or not reports of abuse are investigated, and who receives government-funded welfare. This latest wave of commercial application of AI brings its own concerns, not about the novelty of the technology itself but about the scale of its application.
Despite having a huge impact, governments do not have specific frameworks to audit ML projects within government agencies. Furthermore, most countries have no central oversight agency or policy that regulates AI and ML technology at scale.
The large-scale implementation of AI in governments requires corresponding efforts from the AI Ethics community to provide a method to audit and oversee AI at scale, across complex enterprises. In the past, the AI Ethics community has focused on high-level, and recently on bias mitigation. It is easy to assume that an ML model trained on a representative dataset tested for statistical bias and embedded fairness metrics will continue to perform ethically without oversight. In reality, the environment in which the model is operating is constantly changing, and auditors need to periodically reassess model performance and outcomes with thorough, multidisciplinary auditing teams to avoid unethical outcomes seeping into the models over time since ethics is not a âbolt-onâ, but a continuous process. Ensuring that ML algorithms behave ethically requires regulation, measurement, and consistent auditing.
As governments around the world scale up their investments in AI technology, they will also need to scale up their capability to assess, audit, and review those technologies for ethical concerns to avoid amplifying inequality. Large scale government enterprises require a systemized method to look across their portfolio of projects and quickly assess which are more vulnerable to becoming unethical. This allows appropriate allocation of auditing resources, continual monitoring of ML projectsâ outputs, and appropriately identiďŹes risky projects before they are fully developed and deployed. This auditing process needs to be agile, continuous, and quick enough to meet the government agenciesâ need for self-regulation. In the next wave of AI Ethics development, we need to pry our focus away from high-level principles and bias-only concerns and develop the mundane, practical tools to allow organizations to audit AI. As MIT Technology Reviewâs Karen Hao wrote, âLetâs stop AI ethics-washing and actually do something.â