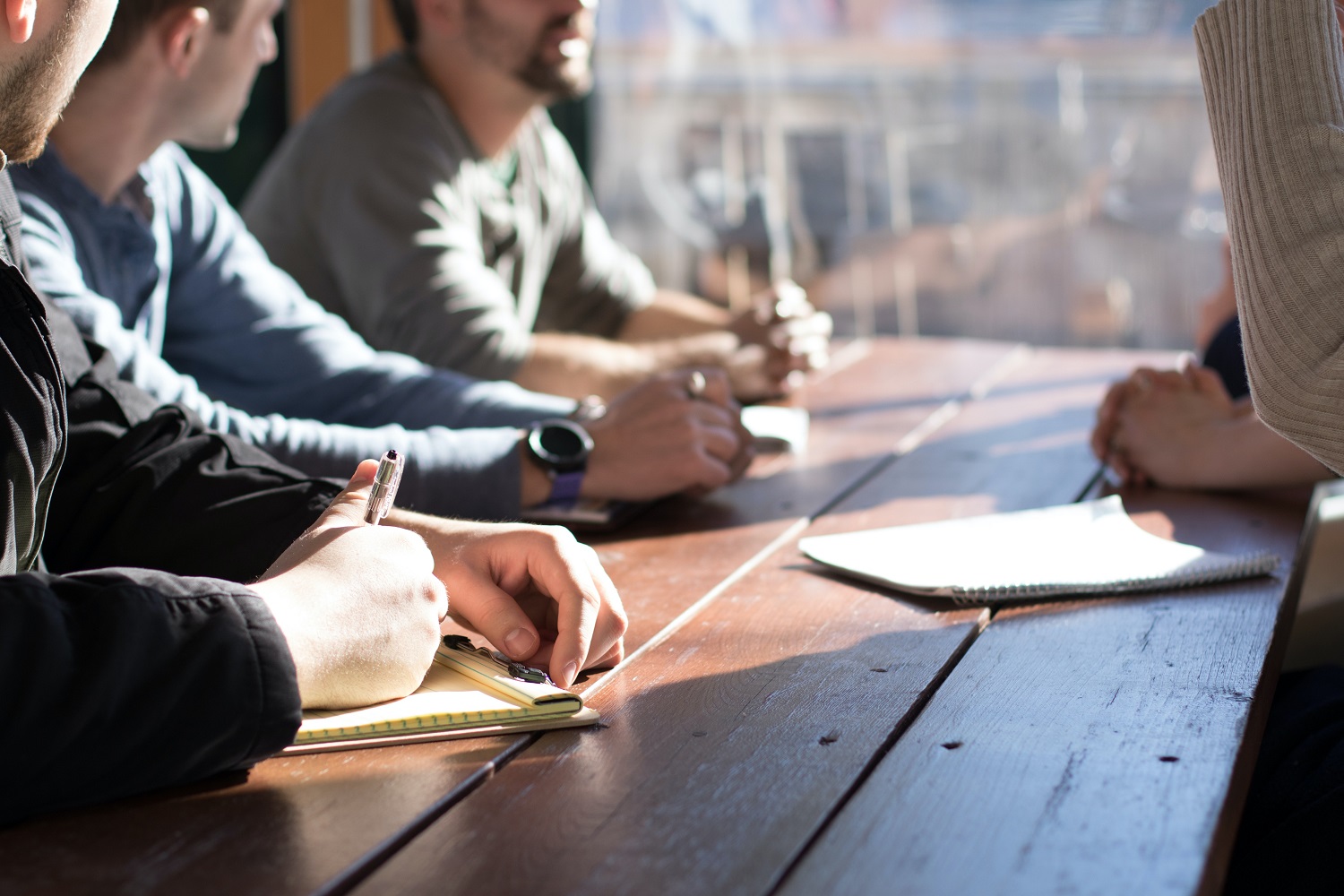
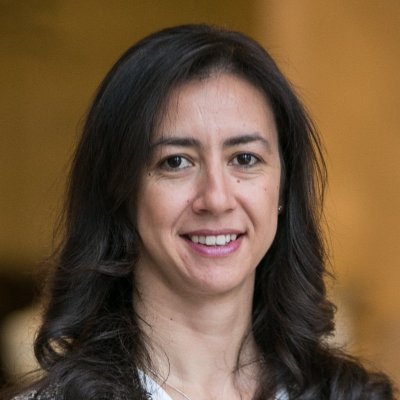
This guest post was contributed by Merve Hickok (SHRM-SCP), founder of AIethicist.org. It is part 1 of a 2-part series on bias in recruitment algorithms. Read part 2 here.
Humans are biased. The algorithms they develop and the data they use can be too, but what does that mean to you as a job applicant coming out of school or looking to move up to the next step in career ladder or considering a change in roles or industry?
In this two-part article, we walk through each stage of recruitment (targeting, sourcing/matching, screening, assessment and social background checks) and explore how some of the AI-powered commercial software used in each stage can lead to unfair and biased decisions.
In the fast-moving world of technology, AI has particularly expanded into many domains of our personal and business life. Whether you are aware of it or not, algorithmic decision making systems are now prominently used by companies as well as governments to make decisions on credit worthiness, housing, recruitment, immigration, healthcare, criminal justice system, pricing of goods, welfare eligibility, college admissions â just to name a few. Despite the high stakes and high impact of these decisions on an individual (not to mention the society as a whole), the landscape still greatly lags behind in developing oversight, transparency and accountability measures around these algorithmic systems. When your movie or music streaming service makes a recommendation, the issues with the accuracy of recommendations against your taste might be an inconvenience but not a big deal in the grand scheme of things. However, when AI systems make recommendations that you are not a good candidate for a certain role, then the issue becomes more serious.
Recruitment has always been one of those areas where people always run the risk of being on the receiving end of a biased decision. Given the historical context on discrimination in recruitment, there are a number of legislation actions which provide guidelines and red lines to organizations to make better choices. Title VII of the Civil Rights Act, for example, prohibits discrimination based on ârace, color, religion, sex, or national originâ that would result in disparate treatment or disparate impact. It also puts the liability and legal responsibility on employers to ensure that the tools being used are not creating such results. Moving inside the organizations, in an effort to be more fair, equitable and diverse many companies have taken upon themselves to improve their hiring processes and eliminate as much as possible perceived legacy structural issues through new technology. As great as these regulations and initiatives are, there is still a long way to go for both the legislation as well as the products and business processes to create more objective and less biased decisions in hiring. Today, companies find AI tools which are used in the full spectrum of hiring process attractive without understanding the potential issues these products might create, or without considering how these results might actually be in complete disagreement with what they want to do with their workforce and future.
The attractiveness of AI-powered recruitment products come from the fact they help companies reach many more candidates than they could reach with the more traditional ways (corporate career websites, referral programs etc.). On the same token, they also make it extremely easy for prospective candidates to submit their CVs to multiple roles at a time with the click of a button. The result is a mutual technology escalation from both the employers and candidates. Once the net is cast, these products help the companies to efficiently process those candidates through the recruitment funnel. The ability to process hundreds of applications in a matter of minutes with an automated system is not only a great benefit in terms of scalability but it also reduces the time to hire and hence potentially the cost to hire (assuming the choices were right) and gives hiring teams more space to develop strategies rather than constantly trying to stay on top of hiring transactions.
One other way these AI recruitment products are marketed is as an alternative to the biased decisions of hiring managers and recruiters and thus to provide a more standard processing of applications. The big issue with this statement is algorithms are not independent of their creators and their biases, nor are they independent of the historical data used to build its models. Algorithms are created by people, about people, for people. In other words, âAlgorithms are opinions embedded in codeâ (Cathy OâNeill, 2017) For long years now, companies have rolled out a number of initiatives to fight the subject and biased decisions involved in hiring decisions. These included training recruiters and hiring managers about unconscious bias so they would be aware of their bias and intentionally and proactively make decisions which are more objective; blinding/hiding certain fields in resumes or applications so hiring managers would not be biased with names, addresses, universities etc; forcing hiring manager and recruiters to have an equal number of male and female candidates in each stage of the recruitment funnel; creating roundtables of hiring committees where candidates are scored against objective criteria and the committee challenges each other on their scores. The list goes on and on.
All these initiatives have some merit in them, but the success varies with the intensity of the effort as well as the culture of the organization trying to make a change. So, when AI systems have potential to reduce bias and reduce cost at the same time, the knee-jerk reaction of some companies to jump on the wagon without asking too many questions is only too natural.
In 2017, LinkedIn CEO Jeff Weiner predicted the impact of automation and AI on recruiting. LinkedIn mapped different process steps of recruiting by comparing the Automation Potential against the Value added with Human Touch. The forecast map suggests the bottom left quadrant refers to those activities which add little incremental value with human touch and the top right quadrant refers to recruiting activities where human touch is required to interpret or augment AI-driven information. When you look at the bottom left quadrant though, you will see how that might be potentially problematic for you as a candidate. These activities range from candidate sourcing to pre-screening/assessments to candidate ranking to social profiling. Since this article was published in 2017, we have not only seen a mushrooming of products launched to meet these ends, but we have also seen recruitment marketing/targeting process to creep towards high automation potential areas too.
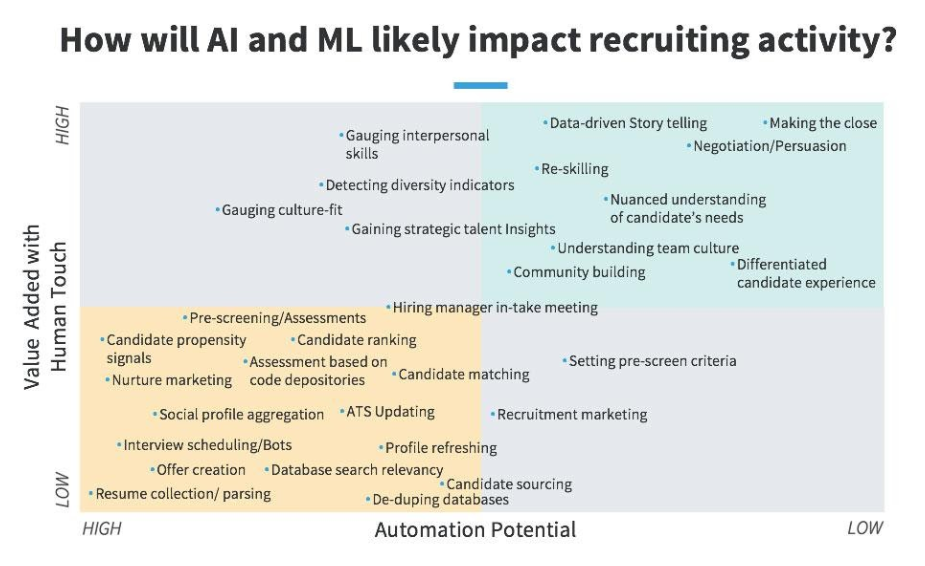
The AI application in each stage of recruitment may be a recommender system using âcollaborative filteringâ which makes recommendations based on historic preference of multiple users for items (clicked, liked, rated, etc.), or a âcontent-basedâ recommendation by matching for example key words in your profile or resume. The algorithm might also be a predictive system which uses historical data to find trends or patterns which are then used to predict usually the future, or the likelihood of something happening (for example analyzing the characteristics of applicants who were hired previously and predict your alignment with their success factors, and hence your future success in the job accordingly). It can generate scores or rankings for example for individuals. Alternatively, the AI system might be using a classification algorithm where it maps the input data (in this case candidates) into different categories or clusters. Imagine the recommender systems as Netflix/Pandora where the machine learning algorithm tries to learn your taste and choice by looking at your historical behavior interacting with that app, and it also analyses people whose choices are similar as yours and refines its recommendation; and the predictive systems as your credit scoring.
It is extremely crucial to remember that with AI systems any outcome or prediction is based upon the training data fed into the system. Nature, context and quality of training data for predictive tools can vary, ranging from click patterns, to historical application data, to past hiring decisions, to performance evaluations and productivity measures.
When you add the errors and biased decisions humans made in the past which made up the dataset to the efficiency of the AI systems, you can appreciate how algorithms can magnify the biased decisions. As they are quoting Timothy Wilson (Strangers to Ourselves (2004) in their paper the authors of “Discrimination In The Age Of Algorithmsâ (Kleinberg & Jens Ludwig & Sendhil Mullainathan & Cass R. Sunstein, 2019) suggest when humans are making decisions, âmany choices happen automatically; the influences of choice can be subconscious; and the rationales we produce are constructed after the fact and on the flyâ. The researchers then suggest âthe black-box nature of the human mind also means that we cannot easily simulate counterfactuals. If hiring managers cannot fully understand why they did what they did, how can even a cooperative manager answer a hypothetical about how he would have proceeded if an applicant had been of a different race or genderâ (Kleinberg et al, 2019). The historical record does not help the case for objectivity or fairness with regards to the employers either. In their analysis of trends in discrimination by performing a meta-analysis on 24 field experiments performed between 1990-2015, which included data from more than 54,000 applications across more than 25,000 positions, Quillian and etc al found there were no changes in hiring rates over time for black applicants over the last 25 years. (Quillian, Pager, Hexel, Midtbøen, 2017).
In the words of Meredith Whittaker, co-founder of the AI Now Institute, âAI is not impartial or neutral. In the case of systems meant to automate candidate search and hiring, we need to ask ourselves: What assumptions about worth, ability and potential do these systems reflect and reproduce? Who was at the table when these assumptions were encoded?â
So, here is a step-by-step review of recruitment activities where things might go wrong for you as an individual candidate, and where it might or might not become an issue for the employers or the companies which develop these products. This is not meant to be an exhaustive analysis of every bias in a recruitment process, but a high-level reminder to the job applicants as well as those involved in the process as recruiters, sourcing specialists and hiring managers. Recruitment process is often likened to a funnel, where the number of candidates is reduced at each stage of the process by different practices until a hiring decision is made.
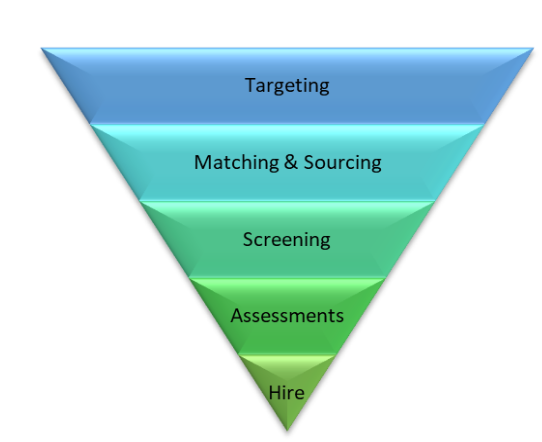
TARGETING:
This is the step when a recruiter tries to cast as wide as a net to the active and passive applicants which would be a strong match to the position that he/she is hiring for. In the digital age, this process has moved from advertising open positions and job descriptions in a companyâs corporate website and company profile to publishing it in different career platforms, and general and niche job boards. For any candidate, active or passive, it is crucial the person sees the posting and hence is aware of the opportunity. If you are not aware of the opportunity in the first place, your chances of getting the role is close to nil.
The data collected from your overall online activity provides the platforms with a way to create groups of users with shared attributes (or characteristics, preferences, interests, etc). Today employers have access to the same microtargeting tools advertisers long had on these job boards (like LinkedIn, Glassdoor, ZipRecruiter, Upsider to name just a few of the most known ones). They can select a number of targeting criteria like job seniority, age, gender, degree, etc, and advertise the job opening to candidates in the boardâs database.
In 2018, Facebook faced a lawsuit which alleged the social media platformâs practice of allowing job advertisers to consciously target online users by gender, race, and zip code constituted evidence of intentional discrimination. Notwithstanding the bias of the recruiter in selecting those criteria and their relevance to job success, the machine learning algorithm in these criteria can collect data on usersâ search histories or demographics, and use algorithms to predict which individuals companies might want to recruit and only show job postings to those candidates. So as an active or passive candidate if your previous job clicks were significantly more in say junior positions, or in a certain department, the chances you will be targeted for the new job opening are smaller for more senior positions or in different departments. The algorithm also learns from the recruiterâs behavior and which previous criteria was clicked and used more in previous postings and suggests those to the recruiter. If you are not intentionally making an effort to go over each criterion and verify it, soon your former behavior becomes a personalized default. âItâs part of a cycle: How people perceive things affects the search results, which affect how people perceive things,â Cynthia Matuszek, a computer ethics professor at University of Maryland and co-author of a study on gender bias in Google image search results says (Carpenter, 2015).
Facebook also offers a tool called âlookalike audienceâ where an employer might provide Facebook data on its current employees. As Pauline Kim describes it, Facebook takes the source audience, analyzes data about them and identifies other users who have similar profiles, and targets ads to this âlookalikeâ group to help employers predict which users are most likely to apply for their jobs (Kim, 2018).
On top of all these, the digital marketing platforms like Google or Facebook use their own marketing algorithms to decide which ads are more likely to be clicked by which users within each of their user groups. So just because a recruiter selected âall females in Chicago with 10 years of work experience in consultingâ does not mean all those females who fit in that category will see the job posting in their feeds. One experiment by the Carnegie Mellon researchers showed that Google displayed advertisements for a career coaching service for â$200k+â executive jobs 1,852 times to the male group and only 318 times to the female group So in other words, these platforms run their own predictions and further narrow the visibility of a job posting (Vincent, 2015).
MATCHING & SOURCING:
If your application made it to the next stage where the candidates are filtered on how much they aligned with the recruiterâs choices, the next set of bias arises from how the algorithm is structured in a way that rank-ordered lists and numerical scores may influence recruiters (Bogen and Rieke, 2018). If a recruiter sees a 95% compatibility vs an 85% compatibility to the job posting, he/she might not even bother to compare the two applications and actually read the totality of the resumes. This issue might snowball further if say the results show only the 10 top ranked applicants per page versus more and the recruiter does not even click to see the rest. On a separate note, when predictions, numerical scores, or rankings are presented as precise and objective, recruiters may give them more weight than they truly warrant, or more deference than a vendor intended (Joachims, Granka, Pan, Hembrooke, and Gay, 2005).
The problem of the algorithm learning from the recruiterâs previous use of filters for candidate matching (i.e. location, skill, previous company, within x mile radius) is also present in this stage and makes future recommendations accordingly.
Read part 2 here.
References:
Barocas, Solon and Selbst, Andrew D., Big Data’s Disparate Impact (2016). 104 California Law Review 671 (2016). https://ssrn.com/abstract=2477899 or http://dx.doi.org/10.2139/ssrn.2477899
Barrett, Lisa Feldman, et al. âEmotional Expressions Reconsidered: Challenges to Inferring Emotion From Human Facial Movements.â Psychological Science in the Public Interest, vol. 20, no. 1, July 2019, pp. 1â68, https://doi.org/10.1177/1529100619832930
Bendick, Marc & Nunes, Ana. (2011). Developing the Research Basis for Controlling Bias in Hiring. Journal of Social Issues. 68. 238-262. 10.1111/j.1540-4560.2012.01747.x. https://www.researchgate.net/publication/235556983_Developing_the_Research_Basis_for_Controlling_Bias_in_Hiring
Bogen, Miranda and Rieke, Aaron. Help wanted: An exploration of hiring algorithms, equity, and bias. Technical report, Upturn, 2018. https://www.upturn.org/reports/2018/hiring-algorithms/
Bolukbasi, Tolga et al. âMan is to Computer Programmer as Woman is to Homemaker? Debiasing Word Embeddings.â https://arxiv.org/pdf/1607.06520.pdf
Buolamwini, Joy and Timnit Gebru. âGender Shades: Intersectional Accuracy Disparities in Commercial Gender Classification.â FAT (2018). http://proceedings.mlr.press/v81/buolamwini18a/buolamwini18a.pdf
Carpenter, Julia, Google’s algorithm shows prestigious job ads to men, but not to women. Independent. 2015 https://www.independent.co.uk/life-style/gadgets-and-tech/news/googles-algorithm-shows-prestigious-job-ads-to-men-but-not-to-women-10372166.html
Chamorro-Premuzic, T., Winsborough, D., Sherman, R., & Hogan, R. (2016). New Talent Signals: Shiny New Objects or a Brave New World? Industrial and Organizational Psychology, 9(3), 621-640 https://doi.org/10.1017/iop.2016.6
Dastin, Jeffrey, Amazon scraps secret AI recruiting tool that showed bias against women. Reuters. 2018 https://www.reuters.com/article/us-amazon-com-jobs-automation-insight/amazon-scraps-secret-ai-recruiting-tool-that-showed-bias-against-women-idUSKCN1MK08G
Duarte, Natasha, Llanso, Emma and Loup, Anna, Mixed Messages? The Limits of Automated Social Media Content Analysis, Center for Democracy & Technology, November 2017, https://cdt.org/files/2017/11/Mixed-Messages-Paper.pdf
Electronic Privacy Information Center (EPIC). EPIC Files Complaint with FTC about Employment Screening Firm HireVue. 2019. https://epic.org/2019/11/epic-files-complaint-with-ftc.html
Geyik, Sahin Cem, Stuart Ambler, and Krishnaram Kenthapadi. âFairness-Aware Ranking in Search & Recommendation Systems with Application to LinkedIn Talent Search.â Proceedings of the 25th ACM SIGKDD International Conference on Knowledge Discovery & Data Mining (2019): https://arxiv.org/abs/1905.01989
Guo, Anhong & Kamar, Ece & Vaughan, Jennifer & Wallach, Hanna & Morris, Meredith. (2019). Toward Fairness in AI for People with Disabilities: A Research Roadmap. https://arxiv.org/abs/1907.02227
Heater, Brian, Facebook settles ACLU job advertisement discrimination suit. TechCrunch. https://techcrunch.com/2019/03/19/facebook-settles-aclu-job-advertisement-discrimination-suit/
Kim, Pauline, Big Data and Artificial Intelligence: New Challenges for Workplace Equality (December 5, 2018). University of Louisville Law Review, Forthcoming. https://ssrn.com/abstract=3296521
Kim, Pauline, Manipulating Opportunity (October 9, 2019). Virginia Law Review, Vol. 106, 2020, Forthcoming.https://ssrn.com/abstract=3466933
Kleinberg & Jens Ludwig & Sendhil Mullainathan & Cass R. Sunstein, 2019. “Discrimination In The Age Of Algorithms,” NBER Working Papers 25548, National Bureau of Economic Research, Inc. https://ideas.repec.org/s/nbr/nberwo.html
Kosinski, M., Stillwell, D., & Graepel, T. (2013). Private traits and attributes are predictable from digital records of human behavior. Proceedings of the National Academy of Sciences of the United States of America, 110(15), 5802â5805. https://www.pnas.org/content/110/15/5802
Lee, Alex. An AI to stop hiring bias could be bad news for disabled people. Wired. 2019. https://www.wired.co.uk/article/ai-hiring-bias-disabled-people
OâNeill, Cathy, The Era of Blind Faith in Bid Data Must End, TedTalk, 2017) https://www.ted.com/talks/cathy_o_neil_the_era_of_blind_faith_in_big_data_must_end?language=en
Raghavan, Manish et al. âMitigating bias in algorithmic hiring: evaluating claims and practices.â Proceedings of the 2020 Conference on Fairness, Accountability, and Transparency (2020): https://arxiv.org/pdf/1906.09208.pdf
Rosenbaum, Eric, Silicon Valley is stumped: A.I. cannot always remove bias from hiring, CNBC. https://www.cnbc.com/2018/05/30/silicon-valley-is-stumped-even-a-i-cannot-remove-bias-from-hiring.html
Schulte, Julius. AI-assisted recruitment is biased. Hereâs how to make it more fair. World Economic Forum. 2019 https://www.weforum.org/agenda/2019/05/ai-assisted-recruitment-is-biased-heres-how-to-beat-it/
Quillian, Lincoln, Pager, Devah, Hexel, Ole, Midtbøen, Arnfinn H.. The persistence of racial discrimination in hiring. Proceedings of the National Academy of Sciences Oct 2017, 114 (41) 10870-10875; https://www.pnas.org/content/pnas/114/41/10870.full.pdf)
Thorsten Joachims, Laura Granka, Bing Pan, Helene Hembrooke, and Geri Gay. 2005. Accurately interpreting clickthrough data as implicit feedback. In Proceedings of the 28th annual international ACM SIGIR conference on Research and development in information retrieval (SIGIR â05). Association for Computing Machinery, New York, NY, USA, 154â161. https://dl.acm.org/doi/10.1145/1076034.1076063
Title VII of the Civil Rights Act of 1964: https://www.eeoc.gov/statutes/title-vii-civil-rights-act-1964
Venkatraman, Sankar. This Chart Reveals Where AI Will Impact Recruiting (and What Skills Make Recruiters Irreplaceable), LinkedIn Blog, 2017. https://business.linkedin.com/talent-solutions/blog/future-of-recruiting/2017/this-chart-reveals-where-AI-will-impact-recruiting-and-what-skills-make-recruiters-irreplaceable
Vincent, James, Google’s algorithms advertise higher paying jobs to more men than women. The Verge. 2015 https://www.theverge.com/2015/7/7/8905037/google-ad-discrimination-adfisher
World Bank, Disability Inclusion. 2020. https://www.worldbank.org/en/topic/disability