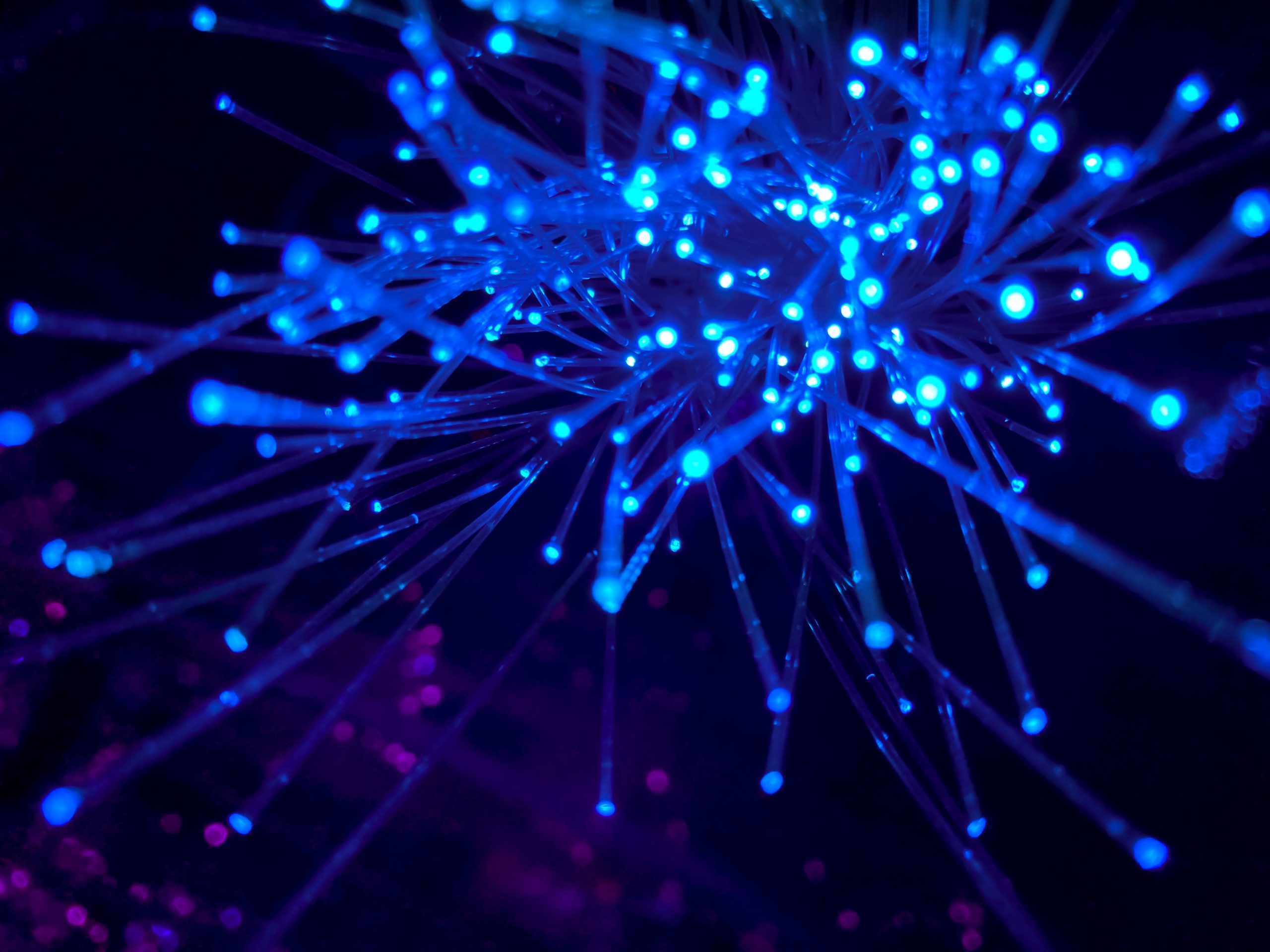

š¬ Research Summary by Verena Schoenmueller, an Assistant Professor in the Marketing Department at Bocconi University. Verenaās research focuses on how user generated content can reflect and affect consumers behavior. Some of the topics she works on include online reviews, fake news, and political brand affiliation.
[Original paper by Verena Schoenmueller, Simon Blanchard, and Gita Johar]
Overview: Many efforts have been made to identify fake news before they are spread on social media platforms. Instead of focusing on identifying fake news content or fake-news publishing domains, we focus on prevention via the identification of fake news sharers before they share. To do so, we use the words they use in their own past tweets and compare them to other actors in the fake-news ecosystem (such as fact-check sharers and random social media users). We find that fake-news sharers use language that is sufficiently distinguishable and can be used to improve our ability to target fake-news sharers.
Introduction
A large amount of past research has shown that fake-news sharers tend to be more likely to come from certain socio-demographic groups (e.g., white, male, older) or carry certain political ideology (i.e., conservative). Although such findings are helpful descriptively to understand who fake-news sharers are, they are less helpful predictively. For example, that fake news are more likely to be conservative does not imply that targeting conservatives is a useful way to identify fake-news sharers (i.e., this kind of reasoning is a common example of the logical fallacy āaffirming the consequentā). As it turns out, socio-demographics, and political affiliation have only limited predictive power in assessing whether any given user is likely to share fake-news.
In this paper, we investigate the use of language in users’ own past tweets to see whether such cues are useful to predict who will share fake news. We find that the language used by individuals in their social media posts (i.e., on Twitter) can offer additional information about their likelihood to share fake news. Compared to others in the eco-system (random users, politically active users, sharers of fact-checking links), we find that users who use a higher proportion of words pertaining to high-arousal negative emotions in their own tweets, and those who use words pertaining to existentially-based needs, are more likely to eventually share fake news.
Key Insights
Actors in the Fake News Ecosystem
Fake-news sharers operate on social media platforms composed of many actors with varying socio-demographics and political affiliation, such that attempting to predict who will share fake news using such observables becomes an exercise fraught with false positives. Consider, for example, Arnold Schwarzenegger – the Republican governor for California. Based on past descriptive research, he carries many of the markers of a fake-news sharer: heās white, older, male, Republican, and heās politically active on social media. The same would apply to social media posters of right-leaning media sources and those who take the time to correct others spreading fake news (i.e., fact-check sharers who post links to politifact or snopes), many of which likely carry similar socio-demographic and social media activity. As such, tentatively labeling individuals on the basis of such observables (age, gender, political affiliation and social-media activity) would be misguided especially when we consider that itās a small portion of social media users who eventually share fake news.
Linguistic Markers of fake-news Sharers and the other actors in the fake-news ecosystem
Given that socio-demographics and social media activity are unlikely to provide useful predictive ability to distinguish fake-news sharers from others who share similar characteristics, we explore whether psycholinguistic characteristics of fake-news sharers, as inferred from their past tweets, can help. We find that fake-news sharers display higher levels of high-arousal negative emotions (i.e., anger and anxiety) compared to random social media users or sharers of conservative news articles, but that these high levels are indistinguishable from those of fact-check sharers. That is, both those who share fake news and those who correct fake-news sharers (i.e., fact-check sharers) have a general tendency to use words relating to high-arousal negative emotions across their tweets. We do find that the use of words related to existentially-based needs (i.e., discussing death and religion frequently) is useful to distinguish who will eventually share fake-news – holding everything else constant (i.e., over and above demographics or political orientation). Based on these findings, the inclusion of emotions and personality traits in predictive models improves our ability to forecast a user’s propensity to share fake-news.
The predictive value of linguistic markers
Identifying potential fake-news sharers in the crowd of social media users
Using multiple samples of ārandomā social media (across users and time) users we investigate how much linguistic markers can help us in the prediction of the individual propensity to share fake-news. We find that the inclusion of psycholinguistic cues that incorporate the language used by social media users can help to more accurately predict fake-news sharers than solely relying on the large set of obvious predictors such as the political affiliation, gender, the followership of fake-news outlets on Twitter or the followership of mainstream media.
Distinguishing fake-news sharers from fact-check sharers
Not all non-sharers of fake-news are alike: we show that it is crucial to distinguish the different actors in the fake-news ecosystem. Most importantly, our results reveal that fact-check sharers may be difficult to distinguish from fake-news sharers due to their (in some respects) similar socio-demographics, social media activity, and even emotions. We find misprediction is clearly lower when taking into account linguistic markers. That is, a model with psycholinguistics is better at labeling fact-check sharers as non-sharers of fake-news compared to a model solely relying on socio-demographics.
Conclusion and Implications
Our work offers a comprehensive profiling of the different actors in the fake-news ecosystem using a holistic set of characteristics, including socio-demographic factors and psycholinguistic cues. We do so by capitalizing on the observed communication of users on social media platforms and applying methodological tools that allow an approximation of the relevant psycholinguistic cues in their language. We compare fake-news sharers to other actors that operate in the fake-news ecosystem, which allows us to uncover fundamental commonalities and differences between the main actors in the fake-news ecosystem and also address previous findings regarding the importance of political ideology in this context. Contrary to past research, we take a less binary view of fake-news sharers and investigate fake-news sharers in the context of a fake-news ecosystem in which many different actors operate. We find that fake-news sharers share linguistic markers with some actors (e.g., elevated levels of anger are shared with fact-check sharers); at the same time, the same markers can differentiate them from other actors in the fake-news ecosystem (e.g., sharers of conservative mainstream media). Our findings offer fruitful ground for future research on the understanding of fake-news sharers and can help in terms of proactively identifying those with a high propensity to share fake-news simply based on their past tweets.
Between the lines
Although descriptively interesting, focusing on the socio-demographic and political affiliation differences of fake-news sharers to non-fake news sharers (e.g., more likely to be white, male, older and conservative) has usefulness when it comes to predicting who is individually likely to share fake-news. We show that the way people write, as captured by their social media post, provides useful cues for models to predict who is most likely to eventually share fake news, improves the predictive accuracy of fake news-sharers, and minimizes risks of false-positives among those most trying to help (fact-check sharers). We hope that other researchers will encourage platforms and other researchers to move beyond socio-demographics and political affiliation in their attempts to find interventions that mitigate the spread of fake-news.