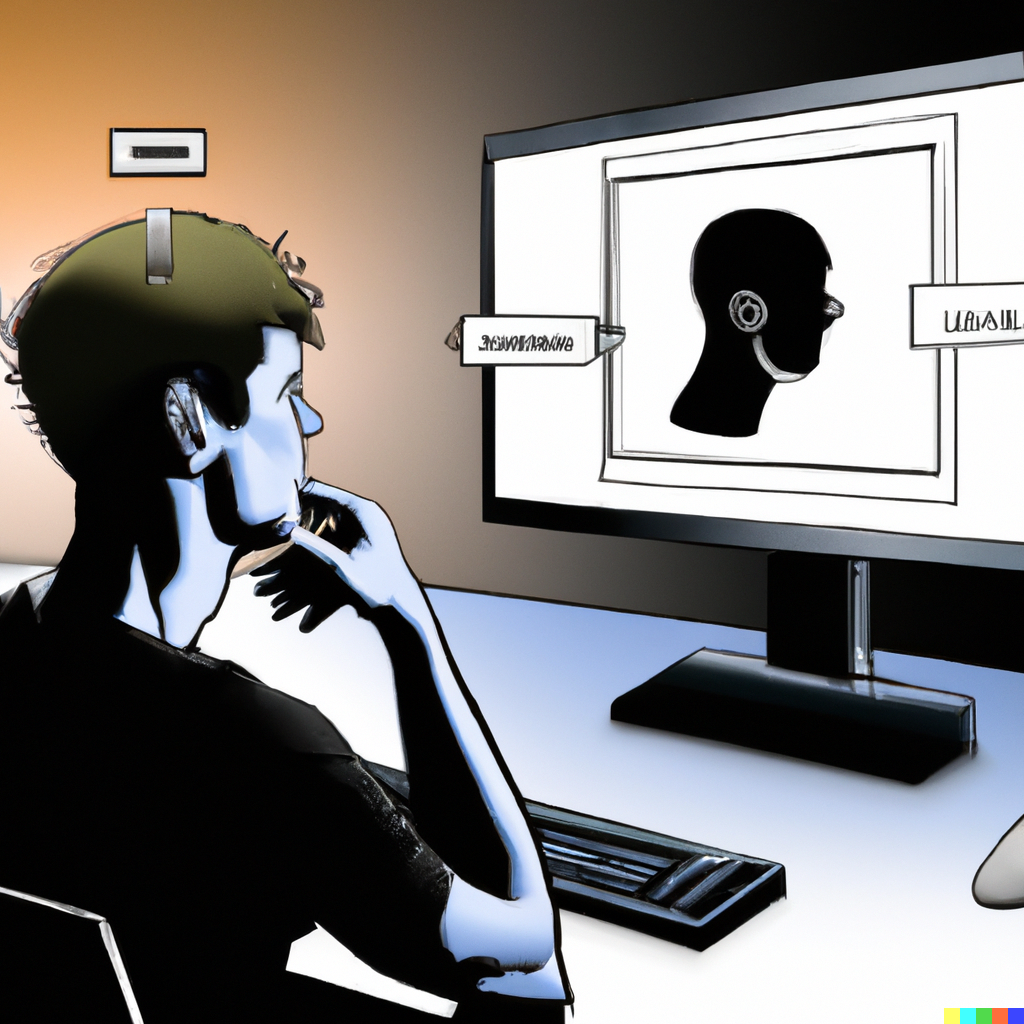
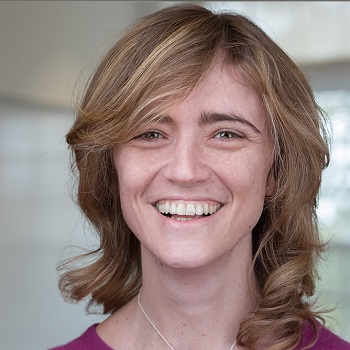
🔬 Research summary by Dr. Marianna Ganapini, our Faculty Director.
[Original paper by Marianna B. Ganapini, Francesco Fabiano, Lior Horesh, Andrea Loreggia, Nicholas Mattei, Keerthiram Murugesan, Vishal Pallagani, Francesca Rossi, Biplav Srivastava, and Brent Venable]
Overview: Nudging is a powerful tool that can be used to influence human behavior in a positive way. However, it is important to use it responsibly and ethically. This paper points to a way to responsibly use nudging in an AI-human collaboration environment. Considering different ways AI could nudge humans to make better, more informed decisions, we propose FASCAI as a promising framework for using nudges to improve decision-making and performance while respecting values such as human autonomy and ensuring human upskilling.
Introduction
Nudging is a concept that has been carefully studied in behavioral psychology and widely adopted in behavioral economics to understand and influence human decision-making. When we nudge, we subtly guide someone’s behavior or thought process in a specific direction. The literature has two primary types of nudges: System 1 and System 2. System 1 nudges take advantage of our quick, instinctive thinking. An example of this is placing fruits and vegetables on easily accessible shelves in a cafeteria to promote healthier eating habits.
On the other hand, System 2 nudges encourage us to engage our slow, reflective thinking. An instance of this technique is the nutritional labels found on packaged food that make us consider our dietary choices. This type of nudge does not push us to make specific choices but to be more reflective and rational in our thinking and decision-making.
The paper also introduces a third type of nudging: metacognitive nudges. These are designed to prompt introspection and push us to gauge our confidence level in accomplishing a specific task. For example, a metacognition nudge might ask us to rate our confidence in our ability to complete a math problem before we attempt to solve it. This can help us avoid making mistakes by taking on tasks beyond our capabilities.
Key Insights
The paper aims to introduce an architecture (FASCAI – Fast and Slow Collaborative AI) able to nudge humans to improve overall performance and decision-making. This framework is adaptable and can nudge humans differently depending on the situation. The architecture takes into consideration the human and the AI past performance (Past[H], Past[AI]) in similar tasks. It also considers the AI confidence level in its solution (low, medium, and high confidence). After that, the AI decides the right course of action. An example of this collaborative decision-making process is illustrated in this table:
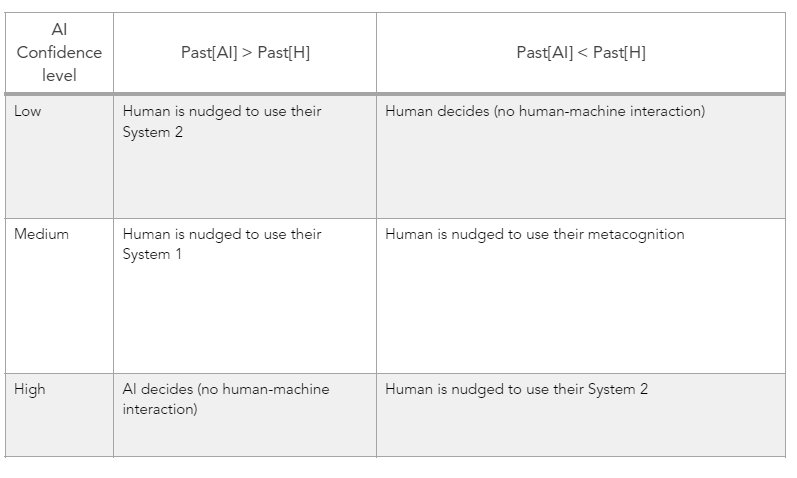
In our framework, we envision six possible situations based on three machine confidence levels and two comparison options for the human vs. machine past performance. The human-machine collaborative framework showcased here includes two instances without nudging. When the human has a superior track record, and the machine is not confident in its own recommendation, the machine lets the human decide in full autonomy without nudging them in any way. Also, it is possible to allow the machine to make the final decision without consulting the human in those cases when the machine is highly confident in its solution, and the human’s past performance is worse than the machine’s. In all other cases, the AI will nudge the human and do so in different ways.
The first nudging strategy prompts FASCAI to nudge the human toward relying on fast thinking (System 1). In this approach, the machine immediately presents its decision recommendation to the human, leaving no time for the human to formulate their initial decision. This would leverage the anchoring bias, making it challenging for the human to diverge from the machine’s suggestion, thereby discouraging deep System 2 thinking. Due to automation and the anchoring bias effect, this interaction mode typically leads humans to follow the machine’s recommendation, although they still retain the freedom to choose otherwise.
As a second interaction method, FASCAI may encourage humans to engage in slow thinking. Here, the machine refrains from immediately revealing the recommended decision and allows the human ample time to formulate their initial decision. This approach encourages humans to compare their own and the machine’s (possibly differing) decisions and determine whether to revise their choice using their slower cognitive processes. This theory is rooted in studies demonstrating that humans tend to engage their System 2 thinking when faced with conflicting options.
The third machine nudging type occurs when the machine motivates the human to employ their own metacognition, enabling them to make a deliberate and conscious choice. The machine patiently waits for the human to formulate their initial decision to achieve this. Once the human has done so, the machine provides the option for the human to view its recommendation, along with information about its confidence and past performance. This inquiry will likely stimulate the human’s introspective thinking, prompting them to assess their confidence level and whether they might gain from the machine’s guidance in reaching the final decision. This approach grants humans substantial autonomy and motivates them to exercise their own decision-making agency, allowing them to determine the most suitable approach for addressing the current task.
Indeed, in the context of the decision scenarios we’re discussing, we operate on the assumption that there are two fundamental principles we aim to uphold: human upskill and human autonomy. Our goal is to empower individuals to have substantial control over their decisions and to enhance their capacity for critical thinking, self-reflection, and introspection as they engage with machines in decision-making processes. To realize this objective, our approach involves using AI-driven System 1 nudges sparingly, resorting to them only when absolutely necessary, and instead favoring the utilization of System 2 and metacognition nudges in all other situations.
Between the Lines
This paper outlines an architecture design that would use nudging techniques in AI-human collaboration environments. Before this is architecture implementable, we need to address several additional research questions. Let me mention some of the areas where we plan to do more research. One of the main assumptions behind FASCAI is that AI can create an ‘anchoring effect’ in humans to steer their decisions towards a certain outcome. In fact, without a significant anchoring effect, we cannot expect machine nudging to achieve the outcome we hope for.
Similarly, it is important to test whether the machine’s anchoring effect on humans is similar in strength compared to the anchoring effect generated by human nudges. Both these questions are especially relevant for the System 1 nudge, where we want humans to be impacted by the nudge coming from the AI. Hence, we plan to test and control for these effects within the project’s scope.
A second pressing issue is whether or not humans are at all willing to accept machine nudging. Humans will likely tend to reject the suggestions coming from AI because they are guided by an anti-machine bias. In this case, they would not trust AI and be skeptical of its ability to deliver reliable results and provide good suggestions. Though some initial empirical results show that humans are sometimes prejudiced against machines, more testing is needed to confirm this.
Finally, in the paper, we strive to build FASCAI as a support system that ensures decision quality and fosters human upskilling and human agency. This desired outcome, however, would need to be tested to ensure that, in fact, human values are preserved in the way we envisioned.