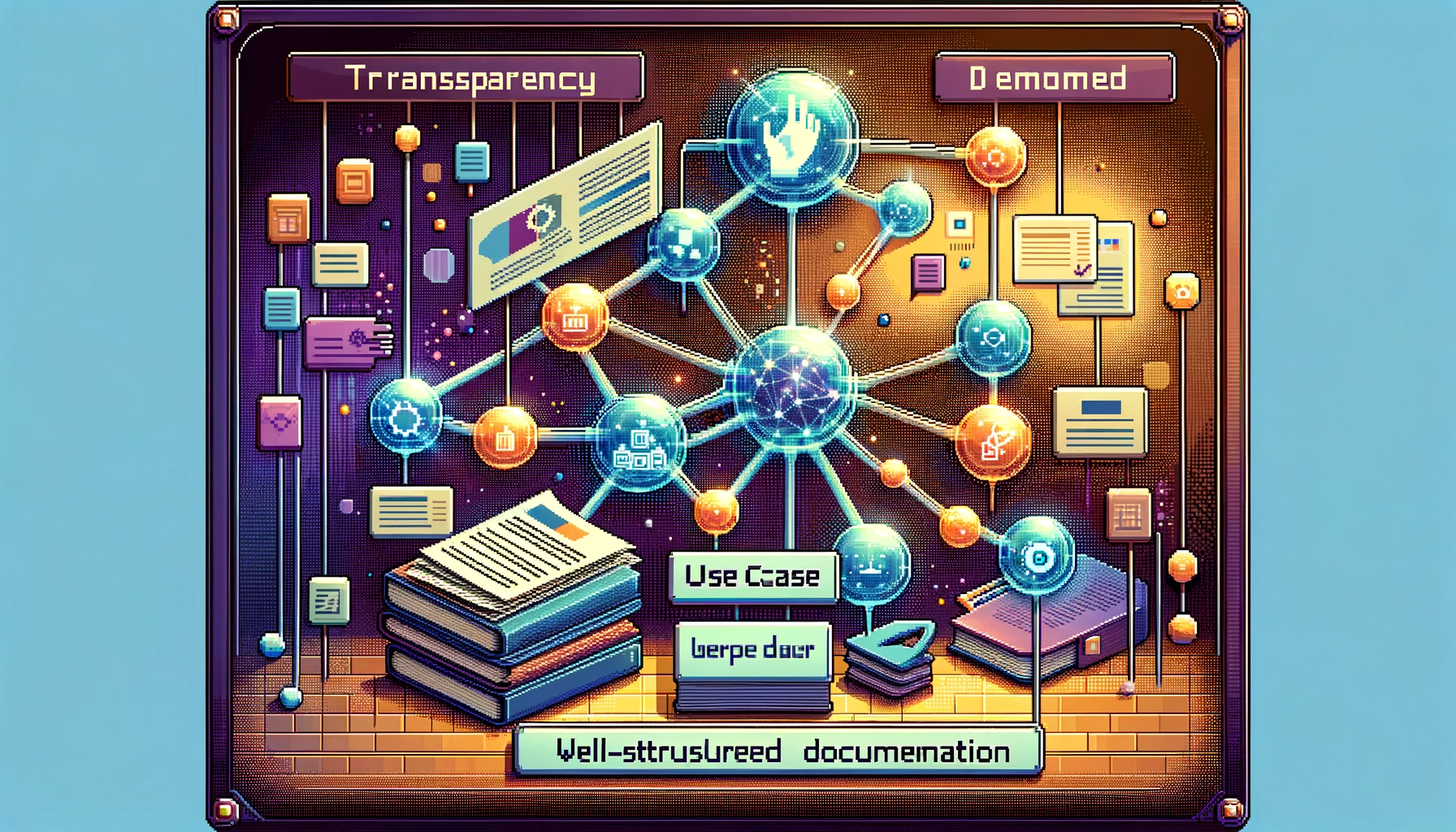
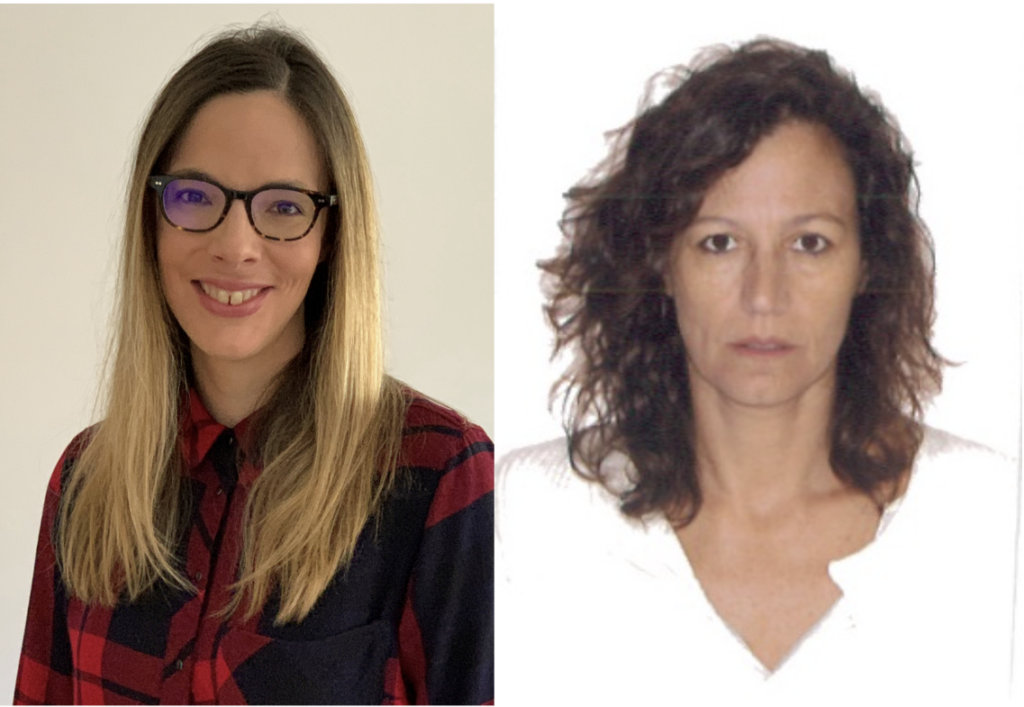
🔬 Research Summary by Isabelle Hupont and Sandra Baldassarri
Isabelle Hupont: Scientific Officer at the Joint Research Centre of the European Commission. Her research interests include emerging digital technologies, with particular emphasis on AI and eXtended Reality, and their impact on human rights and policy-making.
Sandra Baldassarri: PhD, researcher in Human-Computer Interaction and Affective Computing and Associate Professor at the Computer Science Department of the University of Zaragoza, Spain.
[Original paper by Isabelle Hupont, David Fernández-Llorca, Sandra Baldassarri, and Emilia Gómez]
Overview: Transparency in the form of well-structured documentation is a key element of trustworthy artificial intelligence (AI). This is highlighted in most prominent worldwide AI-related guidelines and policies, particularly in the pioneering risk-based AI Act as proposed by the European Commission (EC). This work presents “use case cards,” a UML-based methodology focusing on documenting an AI system in terms of its ‘intended purpose.’ The concept of ‘intended purpose’ is defined in the AI Act proposal as the use for which an AI system is intended by the provider, including the specific context and conditions of use, as specified in the information supplied by the provider in the instructions for use, promotional or sales materials, and statements, as well as in the technical documentation;
Introduction
The need for clear and comprehensive human-centered documentation of AI systems’ use is paramount in the rapidly evolving field of AI. This paper addresses this need by proposing a novel methodology: “use case cards.” This approach is a response to the European AI Act’s emphasis on the importance of documenting AI systems’ intended purpose. The researchers embarked on a collaborative journey involving AI policy experts and UX specialists to develop a standardized template for use case documentation. Their findings reveal that current AI documentation methods fail to address specific use cases in a standardized format. The ”use case cards,” therefore, fill a significant gap, offering a user-friendly and comprehensive tool for documenting AI systems in terms of their intended purpose, which is crucial for understanding their functionality and assessing their risk levels.
Key Insights
At the European Commission’s Joint Research Centre, in collaboration with the University of Zaragoza, we have developed “use case cards,” a new framework for documenting use cases related to AI systems. The framework is inspired by the European AI Act proposal, where the concept of “intended purpose” –closely linked to the one of “use case”– has a central role in determining an AI system’s risk level and, consequently, the regulatory requirements it has to conform with.
The need for a well-structured documentation of use cases
AI is becoming increasingly integrated into our daily lives. It is present in many applications, from decision-support systems that assist professionals in making informed decisions to conversational systems that facilitate human-like interactions with machines. With this exponential trend, there is a need for robust mechanisms to foster a better understanding of AI systems by all stakeholders. Transparency in the form of well-structured documentation practices is considered one of the key mechanisms towards trustworthy AI.
However, current methodologies for AI documentation, such as the popular “Model Cards” or “Datasheets for Datasets,” often focus on the technical characteristics of AI models/data and typically target AI technical practitioners. This approach, while valuable, leaves aside other important personas, such as policymakers or citizens who may not have a deep technical understanding of AI but are nonetheless impacted by its applications. Moreover, when it comes to documenting specific use cases and operational uses of AI systems linked to the concept of ‘intended purpose’ in the AI Act, these documentation approaches are generally limited to a brief textual description without a standardized format. This lack of standardization can lead to inconsistencies and gaps in understanding.
The “use case card” approach
Our “use case card” approach is grounded on the use case modeling included in the Unified Markup Language (UML) standard. Unlike other documentation methodologies, we focus on an AI system’s intended purpose and operational use. The proposed framework consists of two main parts:
- A UML-based table template tailored to cover the information elements linked to the AI system’s intended purpose and relevant for risk assessment. This template includes sections describing the system’s purpose, user interactions, and potential risks or ethical considerations.
- A supporting UML diagram designed to provide information about the system-user interactions and relationships: this visual representation helps to clarify the system’s operation and its impact on users.
This methodology allows for framing and contextualizing use cases in an effective way. It has the potential to be a useful tool for policymakers and AI providers for documenting use cases, assessing the risk level, and building a catalog of existing usages of AI.
Co-Design and Validation Process
The proposed framework results from a co-design process involving a relevant team of policy experts and scientists. We have validated our proposal with 11 experts with different backgrounds and a reasonable knowledge of the AI Act as a prerequisite. We provide the 5 “use case cards” based on real-world products used in the co-design and validation process. This process ensured that our framework was robust, comprehensive, and applicable to various AI use cases.
Conclusion
Our “use case card” approach provides a comprehensive and user-friendly way to document the use cases of AI systems aligned with the European AI Act. It is designed to be accessible to various stakeholders, including policymakers, AI practitioners, and the general public. By providing clear, concise, and relevant information about an AI system’s intended use and operational context, “use case cards” can help foster transparency, trust, and understanding of AI systems.
Between the lines
The findings of this research are significant as they address a critical gap in AI documentation – the lack of a standardized, comprehensive approach focusing exclusively on use cases. However, the research also opens up new questions and directions for further exploration. One gap is the practical application of these cards in diverse and complex real-world scenarios. How will different sectors/industries adapt these cards to their specific needs? Another area for further research could be exploring how these “use case cards” can be integrated into existing AI development workflows. Additionally, as AI technology and regulations evolve, the adaptability and scalability of “use case cards” will be crucial. This research lays a foundational stone in AI documentation but also prompts a continuous journey of adaptation and improvement in the field.