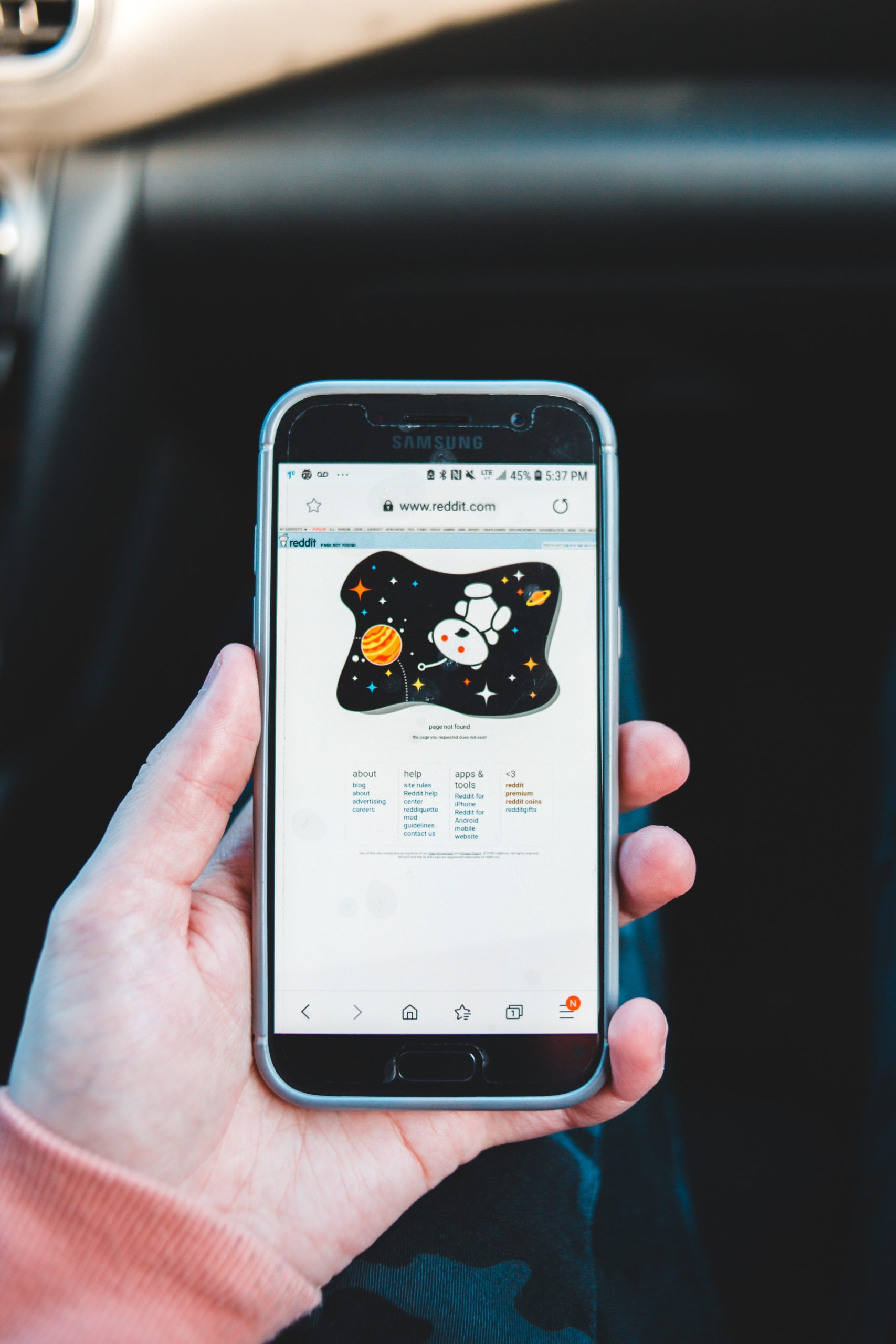
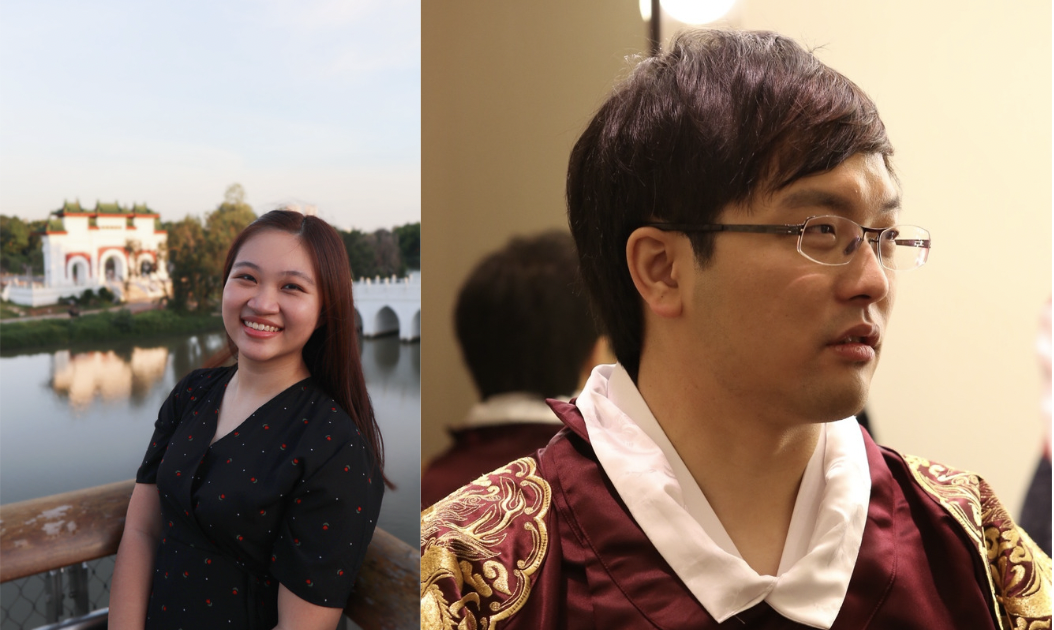
Summary contributed by Yun Yu Chong and Haewoon Kwak.
Chong Yun Yu is a recent graduate from Singapore Management University who is interested in understanding human behaviour through data.
Haewoon Kwak, an associate professor at Singapore Management University who work on social computing.
[Original paper by Yun Yu Chong and Haewoon Kwak]
Overview: While the contagious nature of online toxicity sparked increasing interest in its early detection and prevention, most of the literature focuses on the Western world. In this work, we demonstrate that 1) it is possible to detect toxicity triggers in an Asian online community, and 2) toxicity triggers can be strikingly different between Western and Eastern contexts.
Introduction
âToxicâ comments, which are often rude, vulgar, or discriminative, are prevalent on social media. According to Duggan (2017), 41% of US citizens experienced online harassment as of 2017. Moreover, these toxic comments are contagious; exposure to toxic comments leads to subsequent toxicity.
This contagious nature of online toxicity sparked increasing interest in the communities developing methods for early detection and prevention of hate speech (An et al. 2021). One such effort is detecting toxicity triggers (Almerekhi et al. 2020), which are the origins that initiate toxic discussions. Despite this growing interest, most studies have focused on the Western world, mainly due to the availability of natural language processing tools and data.
In this work, we demonstrate that 1) it is possible to detect toxicity triggers in an Asian online community, and 2) toxicity triggers can be strikingly different between Western and Eastern contexts by comparing the triggers in the Western and Asian online communities. For the Asian community, we focus on Singapore, a multicultural country where English is the dominant language. For the Western community, we study New York City, a multicultural city with a comparable population.
Key Insights
Data for our analysis
On Reddit, the r/singapore subreddit focuses on Singaporean experiences and news. With 473K members as of January 2022, it is the biggest online community for Singaporeans on the web. There are other online communities that focus on Singapore (e.g., HardwareZone.sg Forum), but they are all significantly smaller than r/singapore. For the comparison, we focus on the subreddit r/nyc (573k subscribers as of Jan 2022) is dedicated to discussing general NYC topics. We examine all posts and comments on both subreddits between January 2021 and July 2021.
Toxic comments in r/singapore
We use Perspective API to estimate the toxicity of comments. It successfully estimates toxicity for more than 98% of the comments in the dataset.
The above figure shows the distinguishing words in toxic and non-toxic comments. The top words in toxic comments, as seen in the top left corner of the figure, are all swearwords, while the top words in non-toxic comments, as seen in the bottom right corner, are mostly about COVID-19.
Also, we can see several themes that provide a glimpse of the possible toxicity triggers. Words about culture, race, and religion are prominent, and the three most dominant races in Singapore — Chinese, Indian, and Malay — are explicitly presented. The term âxenophobicâ also further highlights the long-running issue of foreigners in Singapore. Multiple terms refer to the Singaporean government, such as âgov,â âgovt,â âgovernment,â âgahmen,â and many acronyms of government-related persons or agencies, like âoykâ which stands for the Minister of Health, Mr. Ong Ye Kung. These terms are expected due to the strong focus on local news in r/singapore.
Toxicity triggers in r/singapore
An n-toxicity trigger is defined as a non-toxic comment that has at least n toxic replies, meaning that it initiates subsequent toxic discussions (Almerekhi et al. 2020). We fine-tune a pre-trained language model, Bidirectional Encoder Representations from Transformers (BERT), for predicting toxicity triggers (n=2). The prediction model of toxicity triggers achieves an F1-score of 0.79.
We then use Shapley Additive exPlanations (SHAP) to interpret the model. The main idea of SHAP is to find the average marginal contributions of each feature based on all permutations of the observations provided. We perform a qualitative analysis of the comments that contain the top 500 trigger words with the highest SHAP values.
As a result, we identify eight primary categories of toxicity triggers in r/singapore: 1) COVID-19 related news, regulations, and experiences, 2) Racism and xenophobia, 3) Meritocracy and elitism in the local education system, 4) Mental health and stress, 5) Organization screw-ups, 6) Outrageous but trivial acts, 7) Advertisements gone wrong, and 8) ExposĂŠs of scams. Additionally, two kinds of people are most frequently involved in the triggers: (1) local political figures and policymakers, and (2) local social media influencers.
Toxicity triggers in r/nyc
The trigger prediction model for r/nyc obtains an accuracy of 0.76 and an F1-score of 0.77, which is comparable to r/singapore.
We qualitatively analyze comments that contain the top 500 trigger words and identify five main topics, as we did for r/singapore. They are: 1) COVID-19 related news, regulations and experiences, 2) Protests or strikes against big companies, 3) Elections and campaigns, 4) Memes about politics or multinational corporation, and 5) Investments.
Comparison of toxicity triggers between r/singapore and r/nyc
In r/nyc, the triggers are more about rights, power, and money, such as protests and elections, while in r/singapore, the focus is more on social or public issues, like school and mental health.
News about local politicians and COVID-19 are prominent in both communities. However, the names of individual politicians seem to be more common in r/nyc, such as âcuomo,â âeric adams,â and âandrew yang,â while this is less common in r/singapore. It can also be observed that more focus is placed on what an individual politician, such as Governor Cuomo, said or did in the NYC community, while in Singapore, more focus is placed on the governmentsâ actions collectively.
In r/nyc, most government-related issues discussed are politics and economics-related bills, while in r/singapore, they are about pandemic management.
Between the Lines
This study has a strong contextual focus, where the online Singaporean community is studied using computational tools. Machine learning models and their interpretations deepen understanding of controversial issues in Singapore, which helps to learn what Singaporeans uniquely value.
Particularly, our study reveals stark differences in the top triggers between r/singapore and r/nyc. Among the top 200 triggers from each subreddit, we found that only 5% of them are the same words. Even if we look at the top 1,000 triggers from each, only 30% are overlapped. It demonstrates unique triggers in Singapore (and New York City) and calls for follow-up studies of more diverse countries to understand toxicity and its triggers in each corresponding context.
References
Almerekhi, H.; Kwak, H.; Salminen, J.; and Jansen, B. J. 2020. Are these comments triggering? predicting triggers of toxicity in online discussions. In Proceedings of The Web Conference 2020, 3033â3040.
An, J.; Kwak, H.; Lee, C. S.; Jun, B.; and Ahn, Y.-Y. 2021. Predicting Anti-Asian Hateful Users on Twitter during COVID-19. In Findings of the Association for Computational Linguistics: EMNLP 2021, 4655â4666.
Duggan, M. 2017. Online harassment 2017. https://www.pewresearch.org/internet/2017/07/11/onlineharassment-2017/. Accessed: 2022-01-05.