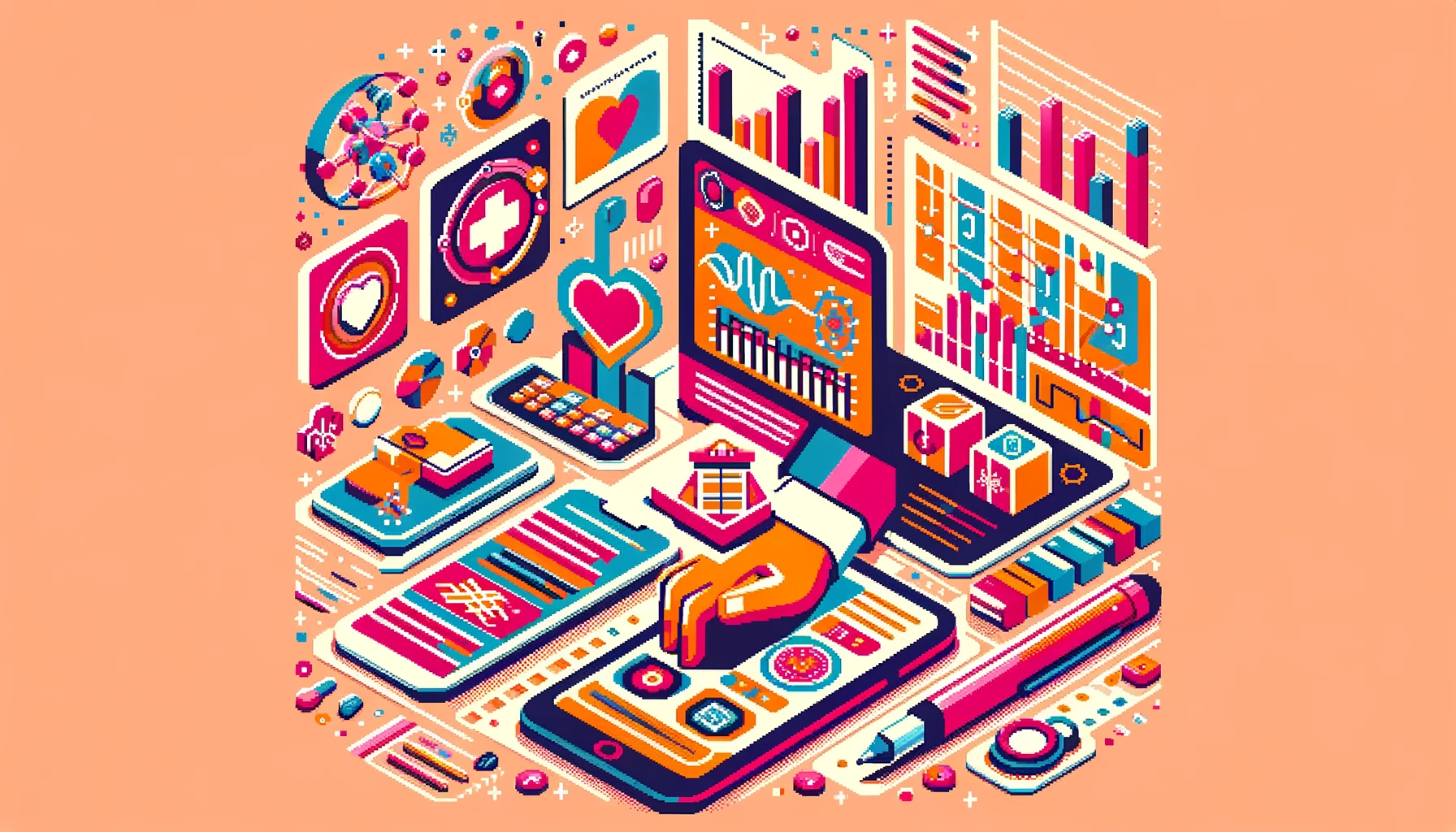
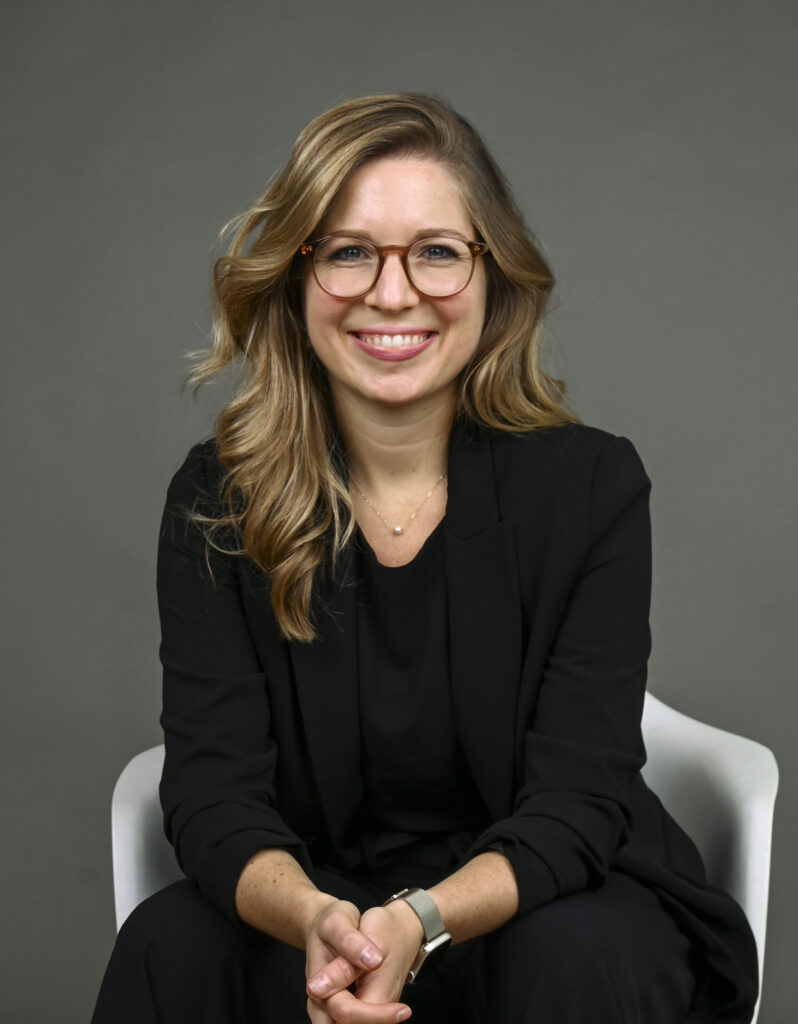
🔬 Research Summary by Michaela Benk, a PhD candidate at the Mobiliar Lab for Analytics at ETH Zurich, researching trust in the context of explainable, interpretable, and transparent AI.
[Original paper by Michaela Benk, Sophie Kerstan, Florian von Wangenheim, and Andrea Ferrario]
Overview: From healthcare to our smartphones, artificial intelligence (AI) is reshaping the world, and our trust in it is considered essential for its successful integration. Amidst a surge of research on ‘trust in AI,’ which research patterns help or hinder advancements in cultivating trust in AI-human interactions? This paper presents findings from a comprehensive bibliometric analysis of two decades of empirical research on trust in AI, offering insights into the field’s productivity, publication patterns, and citation trends, and proposes a research agenda to facilitate more targeted research efforts.
Introduction
Would you trust AI to pick your next vacation spot, give you health advice, or drive your car? Researchers have been exploring questions like these to better understand how or to what extent people trust different AI tools. To contribute to a better understanding of the research landscape and determine which research trends hinder or foster progress in our understanding of this complex concept, the authors conducted a comprehensive bibliometric analysis of two decades of empirical research on trust in AI across various disciplines, uncovering publication patterns, and describing the underlying knowledge structure of the field. They highlight and discuss several trends concerning (a) the rapidly evolving and increasingly heterogeneous research dynamic and the main research themes, (b) the foundational works and research domains that empirical research leverages, and (c) the predominant exploratory nature of empirical research on trust in AI. In light of these trends, the authors outline a research agenda facilitating a shift from exploration toward developing contextualized theoretical frameworks. They argue that such a shift is crucial to cultivate an in-depth understanding of trust in AI, one that can serve as a foundation for practitioners and inform the design of safe and trusted AI.
Key Insights
Dynamic and diverse
A pronounced surge in empirical research on trust AI, particularly over the past three years, underscores the growing interest in the topic. Western countries primarily drive this interest, reflecting a broader trend where only a handful of nations lead the way in AI development (known as the “AI Divide”). Furthermore, while technology-driven fields like computer science are at the forefront of the scientific output and influence in terms of citation counts, research is expanding beyond only tech-focused areas. The research considered in the analysis covers over 150 publication outlets, indicating that experts from various domains and application areas, such as psychology, management, or transportation, are interested in the topic.
Highly contextual, but drawing from selected disciplines
Research approaches trust as a nuanced, multifaceted concept, sometimes considering it as a feeling (e.g., asking people whether they would trust the AI) and other times as a behavior (e.g., observing whether people accept the AI’s recommendations). The context often determines what trust-fostering elements researchers focus on. In the context of consumer research, where products or services are evaluated based on user experience, a design that is easy and intuitive for users can be key. For example, if an AI-powered app is user-friendly, people may be more inclined to trust its recommendations because they feel at ease with the interface and the user experience. However, when we shift our focus to situations where AI systems are making decisions based on complex algorithms, the criteria change. In these scenarios, it’s less about the design and more about understanding how the AI arrives at its decisions to ensure the AI’s decision-making process is both understandable and consistent in its accuracy (or “reliability”). If users can understand the AI’s reasoning and believe that it will consistently make good decisions, they may be more likely to trust it.
These contexts have diversified and differ from traditional automated tools that simply replicate human tasks. Rather, they move toward more interactive formats aimed at augmenting humans (e.g., trusting self-driving cars or writing tools). Interestingly, although studies approach trust from various angles, there is a notable intersection in their foundational theoretical references, mainly from three fields: human factors and ergonomics, social robotics, and management, technology, and economics. However, these do not reflect the diversity in application areas nor the changing dynamics between humans and AI.
Mostly exploratory
A significant gap could be found between foundational theoretical frameworks on trust in AI and their application in empirical studies. Despite the frequent citation of these foundational works, most articles included in the qualitative analysis do not develop a theoretical model or hypothesis on trust in AI, and a large portion does not include any trust model. Moreover, the discussion on trust is typically brief, leaving readers without a comprehensive understanding of the concept or its implications. This indicates that current research predominantly prioritizes exploration and discovery over establishing robust theoretical frameworks. Such frameworks are vital for practitioners, as they can guide the design of AI systems that are both safe and trusted.
Moving Forward
Despite the increasing volume of research output, the dominant approach to trust in AI remains largely exploratory. This method lacks the rigor and reproducibility of other research methodologies, making it less suitable to guide practitioners effectively. The authors advocate for a transition towards more comprehensive studies that can inform the development of theoretical frameworks. To this end, the authors propose a more contextualized approach to studying trust in AI, which involves developing a taxonomy of human-AI interactions and clarifying the applicability of existing foundational works to different AI systems and interactions.
As developing a more robust, theoretically grounded approach may take time, the authors offer a few suggestions for ongoing empirical research on trust. First, researchers may reassess the weight given to trust in their investigations by focusing on more descriptive markers of trusting beliefs. When using quantitative measures, using behavioral manifestations of trust, such as reliance, may offer more robust results. Lastly, transparently disclosing when researchers are trying to explore or discover new insights rather than contribute to a theoretical understanding of trust could help build knowledge more effectively. Following these suggestions prevents basing the design and deployment of AI systems on unconfirmed or non-applicable results.
Between the lines
While the importance of ‘trust in AI’ is frequently mentioned, is research truly developing an in-depth understanding of this pivotal concept or merely scratching the surface? To design safe and reliable AI that people may trust, particularly in high-risk sectors such as healthcare, it’s imperative that practitioners can rely on empirical insights anchored in solid theoretical frameworks. As new AI systems, such as Large Language Models (LLMs), emerge and interaction formats increasingly deviate from the traditional automated systems that have informed early foundational works, a contextualized approach is necessary to further our understanding of trust in diverse application areas. This research highlights the pressing need to transition from mere exploration to an in-depth, contextualized understanding of trust in AI.