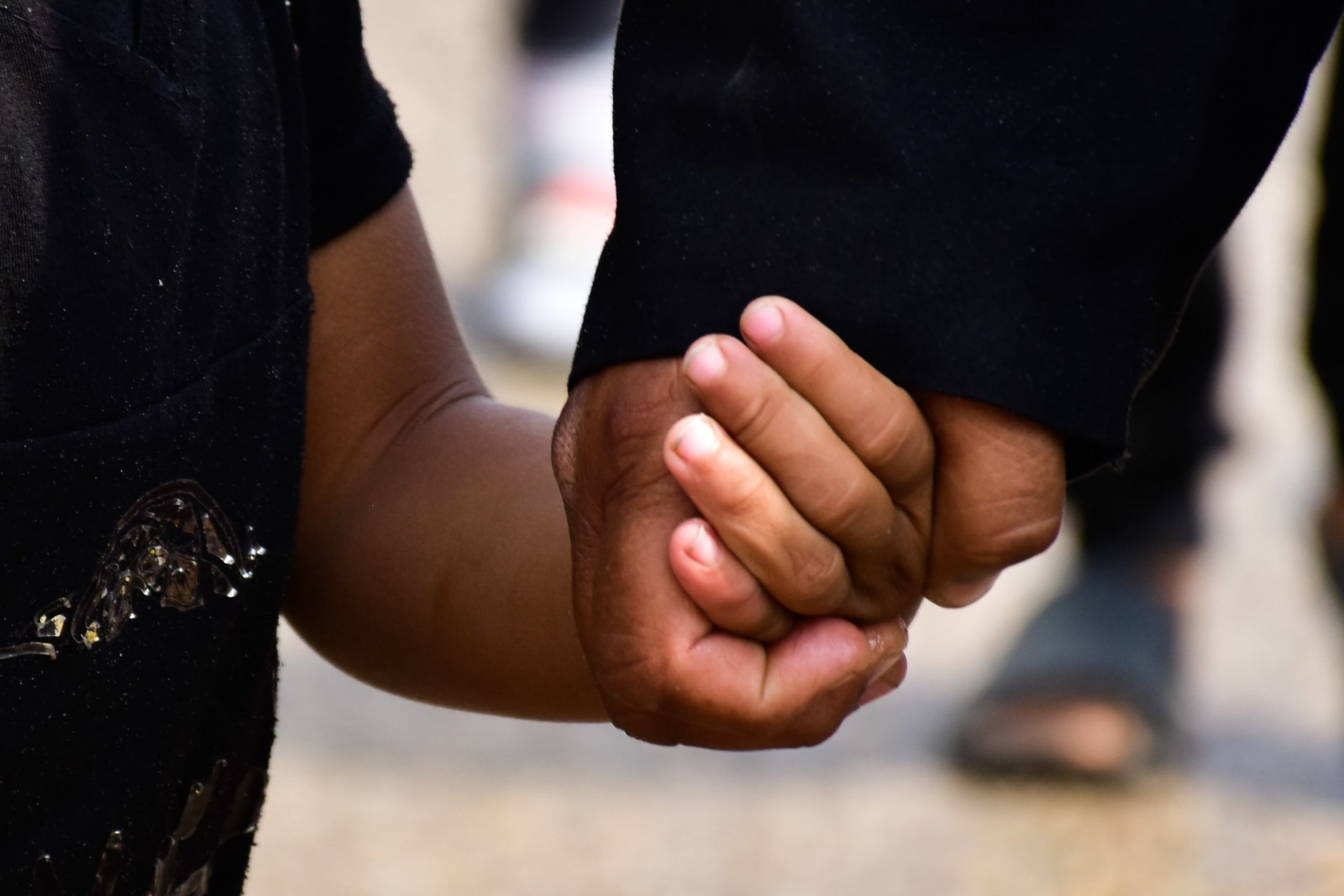
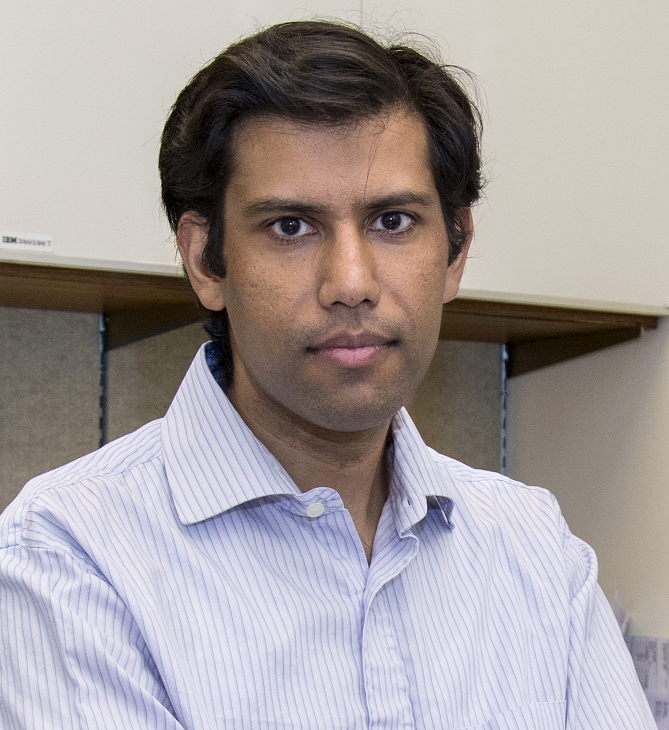
š¬ Original article by Kush R. Varshney, a distinguished research staff member at IBM Research and author of the book Trustworthy Machine Learning.
The dramatis personae of the 2013 play āThe (curious case of the) Watson Intelligenceā features four Watsons:
- Dr. John H. Watson, the assistant of fictional detective Sherlock Holmes,
- Thomas A. Watson, the assistant of telephone inventor Alexander Graham Bell,
- Watson, the IBM computer system that won the television quiz show Jeopardy! and was later touted as an assistant for various professions, and
- a present-day computer repairman.
The members of this āmanelā (man-only panel) are all intelligent advisors. They can help figure out cures to various ills, whether in machines, people, or mysterious circumstances. But according to a fifth Watsonā the famous nursing theorist Jean Watsonācuring alone is not enough. Caring is the key to unlocking health. Her theory of human caring emphasizes carative factors of kindness and equanimity (in contrast to technical curative factors) that begin with treating all patients as they are and respecting their values, even if they are different from your own. And such caring is what I believe is lacking in how we approach the development and application of machine learning systems and artificial intelligence (AI) more broadly today.
In his 1941 paper āIndustrial Mathematics,ā Thornton Fry describes how industrial mathematicians must act as consultants on different projects and lays out five qualities that they should possess.
- They should be able to straddle the theoretical and the practical.
- They must be gregarious and sympathetic.
- They must be cooperative and unselfish.
- They must be versatile enough to use the right tool for the problem at hand.
- They must have outstanding ability.
These qualities are broader than those required to simply be a research scientist or engineer. They imply a great amount of caring.
I began my professional career in 2010 as an industrial mathematician at IBM Research. Except for the fifth quality, which is debatable, I think I espoused all of Fryās qualities in my first eight years of work applying existing methods from statistics, data mining, machine learning, signal processing, and optimization and inventing new ones in engagements with clients focused on human capital management, health systems, and sustainable development. And it was care that revealed ethical aspects of the problems we were working on. In developing a model for predicting IBM employees at risk of voluntarily resigning so that we may proactively offer them retention incentives, interpretability was much more important than using a fancy new-fangled (and opaque) machine learning algorithm. In the projectās wake, we developed a new principled general approach for learning Boolean classification rules. In working with a large American health insurance company to develop a model for predicting health cost profiles in new markets opened up by the Obamacare laws, privacy and distributional robustness were key concerns. In the aftermath, we came up with a new broadly-applicable algorithm for distribution-preserving k-anonymity. When consulting with Simpa Networks, a provider of pay-as-you-go solar power systems for homes in rural Indian villages, developing a repayment prediction model brought up issues of unwanted caste- and religion-based discrimination, which later led to a general bias mitigation algorithm for fairness.
But this caring was lost during the Great AI War of 2018 when I was tabbed as an AI scientist. Our group underwent a reorg and change in mission that put a singular focus on publishing papers in top AI conferences. We continued to pursue topics in fairness, explainability and robustness (which became āhot topicsā around that time). However, conforming to the values of other AI researchers, we presented our work through the lenses of novelty and building on previous work. Our truly grounded motivation was but a remnant from the engagements we conducted previously with care. Our papers were filled with reams of comparison tables on toy datasets and an epistemology that did not fathom any other way of evaluating research besides reporting numbers on the Adult dataset and its ilk. Improving lives, livelihoods, and liberty was only a sentence to write in the introduction of a paper.
I was based at the IBM Research lab in Kenya for a few months in late 2019 working on a project sponsored by the Bill and Melinda Gates Foundation analyzing maternal and newborn health in sub-Saharan Africa using explainable AI. Despite being embedded in the affected community, our approach was not one of care. And that was mostly because of the way the Gates Foundation is organized. As our contact Nosa Orobaton explained, Gates usually maintains a clear separation between pure science often conducted in Seattle and translation/scaling efforts conducted by country offices in the developing world. The sponsored research could only be imagined as basic science. The results, although insightful, have not been impactful.
Based on these experiences, I argue that simply developing ānovelā methods in algorithmic fairness or other areas of trustworthy machine learning, despite being curative, does not fully constitute ethical AI because it is not a carative approach. Ethical AI must be carative AI with moral accompaniment: doing whatever it takes to journey with the marginalized until justice is achieved. That means starting with the real-world problem as experienced by the most vulnerable people: listening to them and understanding their values using the gregariousness and sympathy of the nurse, industrial mathematician, and consultant. That means meeting them where they are and working toward a solution to their problem all the way to the end, even if it involves a lot of grunt work and doesnāt lead to a flashy NeurIPS paper. That means conducting a qualitative assessment of the entire solution by interviewing the affected communities.But an ethical AI researcher can and should be more than a nurse or consultant. The nursing process is composed of four main steps: (1) assessment/diagnosis, (2) planning, (3) implementation, and (4) evaluation. A researcher should pursue one more step: (5) identifying general findings that are applicable to future real-world problems. A five-step cycle that includes the four nursing steps and the fifth learning step is the central premise of action research, shown in the figure from Susman and Evered (1978) below. Action research traces its beginnings to a 1946 paper by Kurt Lewin entitled āAction Research and Minority Problems.ā It centers the values of members of marginalized groups and includes them as co-creators. The generalization and abstraction at the end is what excites a lot of AI scientists and is certainly an utmost goal. However, it is hazardous to take a shortcut and jump straight to it without accompanying affected communities from the very beginning of their journeys. And that is what Iām afraid is happening too much today, my research included.
At various points in my own journey, I maintained a separation between AI for social impact and ethical/trustworthy AI. But it is in fact the care taken in well-done AI-for-social-good projects that makes for ethical AI. To conclude, I call on the ethical AI research community to rally around care, action research, and the problems of the most vulnerable to be the be-all and end-all of ethical AI. Of all the Watsons we could be inspired by, let us be inspired by Jean Watson.
This article is dedicated to the amazing nursing profession that was broken during the Covid-19 pandemic and whose care society cannot survive without.