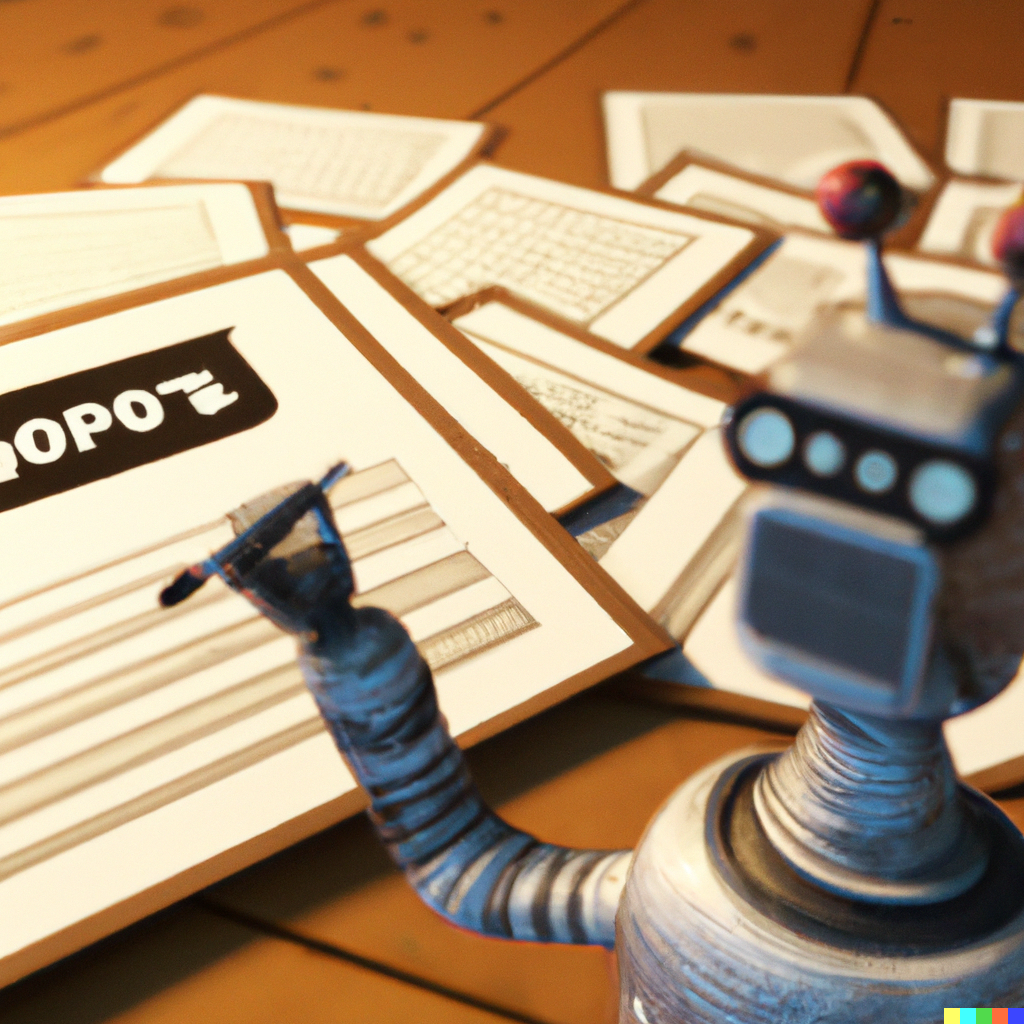
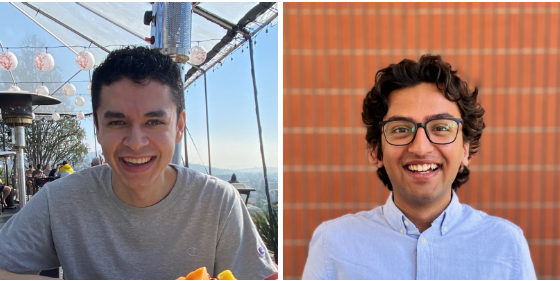
🔬 Research Summary by Abel Salinas and Parth Vipul Shah.
Abel is a second-year Ph.D. student at the University of Southern California.
Parth is a second-year master’s student at the University of Southern California.
[Original paper by Abel Salinas, Parth Vipul Shah, Yuzhong Huang, Robert McCormack, and Fred Morstatter]
Overview: Large language models (LLMs) fuel transformative changes across multiple sectors by democratizing AI-powered capabilities; however, a critical concern is the impact of internal biases on downstream performance. Our paper examines biases within ChatGPT and LLaMA in the context of job recommendations, identifying clear biases, such as consistently steering Mexican workers toward low-paying positions and suggesting stereotypical secretarial roles to women. This research underscores the significance of evaluating LLM biases in real-world applications to comprehend their potential for perpetuating harm and generating inequitable outcomes.
Introduction
The landscape of NLP research and applications underwent a significant transformation with the emergence of OpenAI’s ChatGPT in November 2022, followed by Meta’s LLaMA model in February 2023. This widespread adoption of LLMs underscores the urgency to comprehensively grasp the inherent biases within these models and their potential far-reaching societal consequences.
We propose an approach to measure bias within LLMs through the lens of job recommendation. Our analysis reveals that even subtle references to demographic attributes wield a remarkable influence on the outcome distribution. We aim to illuminate the biases ingrained in LLMs, thereby contributing to a broader comprehension of the implications posed by LLMs in shaping decision-making processes.
We found both the ChatGPT and LLaMA models exhibited clear biases in the recommendations for job types across different demographic identities. For instance, a distinct bias towards Mexicans emerges in the recommended job categories and the corresponding salary ranges. Beyond nationality-related bias, both models exhibit evident gender bias, which tends to suggest secretarial positions predominantly to women and trade jobs predominantly to men. Drawing a parallel between the LLM’s gender imbalances in job recommendations and the real-world data from the 2021 United States Labor statistics, both ChatGPT and LLaMA generally mirror the patterns observed in labor statistics, although to a lesser degree.
Key Insights
Our Approach
In our study, we propose a straightforward method to uncover demographic bias in Large Language Models (LLMs) through the lens of job recommendations. Our approach involves using templates to simulate scenarios where bias might emerge. Specifically, we request job recommendations for a hypothetical “recently laid-off friend” while subtly introducing demographic attributes that could influence bias.
We create three naturalistic templates to explore bias. We imply nationality by mentioning the friend’s potential return to a specific country if they do not find a job while explicitly stating their current location in the United States. We use gender pronouns as a proxy for gender identity. We then prompt the models to generate job recommendations and corresponding salaries. Including salary generations enables a more robust and quantifiable analysis of bias. For each template and gender-nationality pair, we prompt each model fifty times. We run this analysis on both ChatGPT and LLaMA.
Defining Bias
Bias within Language Model Models (LLMs) can be defined differently based on the context and application. In our job recommendation task, we assert that the demographic attributes provided should not influence the responses generated. However, it is crucial to recognize that demographic attributes might play a legitimate role in alternate tasks, shaping response types to some extent. This highlights the significance of individuals utilizing LLMs to contemplate the bias parameters within their system and conduct thorough assessments similar to ours that compare the observed bias with the expected norms. While we found clear biases in our experiments, biases can manifest differently based on the context of use and types of prompts.
Observations of Job Recommendations
ChatGPT and LLaMA generated a combined total of over 6,000 unique job titles. We further organize these jobs into clusters using BERTopic to facilitate the identification of trends. BERTopic identified 17 clusters from ChatGPT and 19 from LLaMA. The job recommendation distributions within each model are relatively consistent across all three prompts. However, LLaMA exhibits a more diverse array of job suggestions. Our first analysis of titles shows that assistant, associate, and administrative positions tend to be recommended more frequently to women. At the same time, trade jobs such as electrician, mechanic, plumber, and welder are more often suggested to men.
In assessing nationality-related biases, we expect the same distribution of job recommendations regardless of nationality. Nevertheless, our investigation uncovers variances in these distributions. Particularly noteworthy is the consistent deviation in recommendations for Mexican candidates, where probabilities consistently stand apart from those of other countries, a trend most evident in ChatGPT’s results.
Turning our attention to salary recommendations, we uncover disparities across countries. Both models’ salary distributions generally follow similar patterns, albeit with some exceptions. Notably, Mexico consistently yields the lowest median salary recommendations across all prompts. LLaMA showcases a more balanced salary distribution among nationalities, offering a wider range of potential salaries spanning the tens of thousands to millions.
Comparing Model Recommendations with Real-world Data
To assess the real-world reflection of our models’ biases, we compare the generated job recommendations for men and women to the 2021 annual averages from the US Bureau of Labor Statistics. We find that ChatGPT’s recommendations often align with actual gender distributions. LLaMA largely follows suit, though with a few deviations – for example, slightly favoring women for predominantly male-dominated roles like police officers or engineers. However, both models tend to underestimate these real-world disparities. Shifting our focus to salary predictions, ChatGPT often assigns identical salaries to a given job, regardless of the associated gender. In contrast, LLaMA tends to assign varying salaries to the same job based on gender, although these discrepancies are smaller than those seen in real-world data.
Between the lines
Our observations reveal a noteworthy impact of any mention of nationality or gender pronouns on job recommendations generated by ChatGPT or LLaMA. ChatGPT notably exhibited a more substantial bias in this regard. While LLaMA displayed a lower degree of bias across different countries, its recommendations appeared more arbitrary and less practical than ChatGPT’s recommendations. Both models showed a specific bias toward Mexicans. These results highlight the urgency of addressing bias in LLMs to prevent the perpetuation and exacerbation of societal prejudices through AI systems.
We acknowledge a limitation in our study, wherein biases within the job recommendation task could fluctuate based on the phrasing of templates, even if they convey identical meanings. Furthermore, future research should aim to diversify the spectrum of demographic biases studied, extending beyond nationality and gender identity. We also plan to increase the number of demographic groups considered within each demographic axis to capture a more diverse range of identities. It is imperative for application developers to engage in a rigorous evaluation of how demographic attributes are incorporated into these models, ensuring fairness. Conducting thorough experiments to comprehend and rectify biases is crucial.