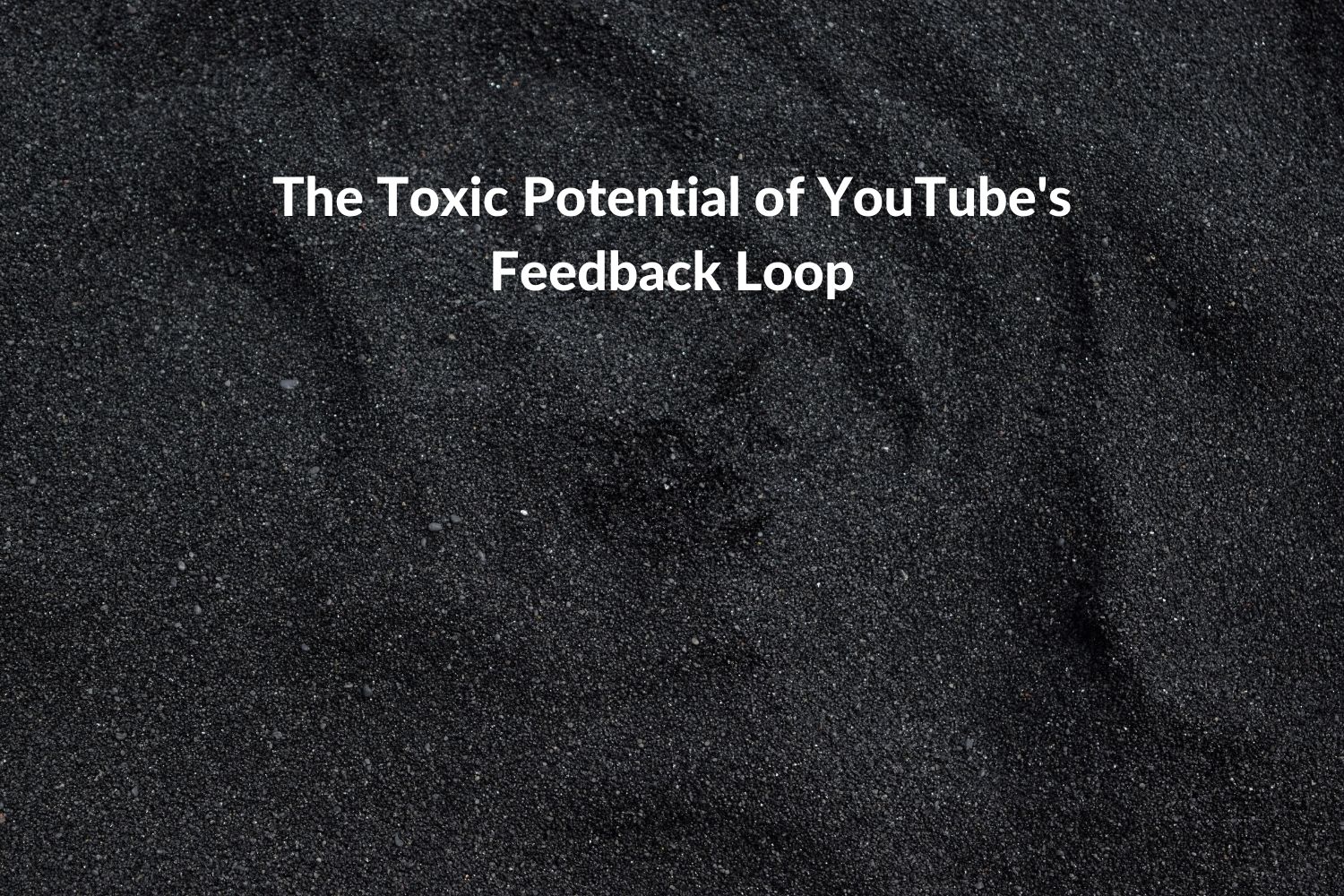
This summary is based on a talk from the CADE Tech Policy Workshop: New Challenges for Regulation in late 2019. The speaker, Guillaume Chaslot, previously worked at YouTube and had first hand experience with the design of the algorithms driving the platform and its unintended negative consequences. In the talk he explores the incentive misalignment, the rise of extreme content, and some potential solutions.
On YouTube everyday, more than a billion hours of video are watched everyday where approximately 70% of those are watched by automated systems that then provide recommendations on what videos to watch next for human users in the column on the side. There are more than 2 billion users on the YouTube platform so this has a significant impact on what the world watches. Guillaume had started to notice a pattern in the recommended videos which tended towards radicalizing, extreme and polarizing content which were underlying the upward trend of watch times on the platform. On raising these concerns with the team, at first there were very few incentives for anyone to address issues of ethics and bias as it related to promoting this type of content because they feared that it would drive down watch time, the key business metric that was being optimized for by the team. So maximizing engagement stood in contrast to the quality of time that was spent on the platform.
The vicious feedback loop that it triggered was that as such divisive content performed better, the AI systems promoted this to optimize for engagement and subsequently content creators who saw this kind of content doing better created more of such content in the hopes of doing well on the platform. The proliferation of conspiracy theories, extreme and divisive content thus fed its own demand because of a misguided business metric that ignored social externalities. Flat earthers, anti-vaxxers and other such content creators perform well because the people behind this content are a very active community that spend a lot of effort in creating these videos, thus meeting high quality standards and further feeding the toxic loop. Content from people like Alex Jones and Trump tended to perform well because of the above reasons as well.
Guillaume’s project AlgoTransparency essentially clicks through video recommendations on YouTube to figure out if there are feedback loops. He started this with the hopes of highlighting latent problems in the platforms that continue to persist despite policy changes, for example with YouTube attempting to automate the removal of reported and offensive videos. He suggests that the current separation of the policy and engagement algorithm leads to problems like gaming of the platform algorithm by motivated state actors that seek to disrupt democratic processes of a foreign nation. The platforms on the other hand have very few incentives to make changes because the type of content emerging from such activity leads to higher engagement which ultimately boosts their bottom line. He recommends having a combined system that can jointly optimize for both thus helping to minimize problems like the above. A lot of the problems are those of algorithmic amplification rather than content curation. Many metrics like number of views, shares, and likes don’t capture what needs to be captured. For example, the types of comments, reports filed, and granularity of why those reports are filed. That would allow for a smarter way to combat the spread of such content. However, the use of such explicit signals compared to the more implicit ones like number of views comes at the cost of breaking the seamlessness of the user experience. Again we run into the issue of a lack of motivation on part of the companies to do things that might drive down engagement and hurt revenue streams.
The talk gives a few more examples of how people figured out ways to circumvent checks around the reporting and automated take-down mechanisms by disabling comments on the videos which could previously be used to identify suspicious content. An overarching recommendation made by Guillaume in better managing a more advanced AI system is to understand the underlying metrics that the system is optimizing for and then envision scenarios of what would happen if the system had access to unlimited data.
Thinking of self-driving cars, an ideal scenario would be to have full conversion of the traffic ecosystem to one that is autonomous leading to fewer deaths but during the transition phase, having the right incentives is key to making a system that will work in favor of social welfare. If one were to imagine a self-driving car that shows ads while the passenger is in the car, it would want to have a longer drive time and would presumably favor longer routes and traffic jams thus creating a sub-optimal scenario overall for the traffic ecosystem. On the other hand, a system that has the goal of getting from A to B as quickly and safely as possible wouldn’t fall into such a trap. Ultimately, we need to design AI systems such that they help humans flourish overall rather than optimize for monetary incentives which might run counter to the welfare of people at large.