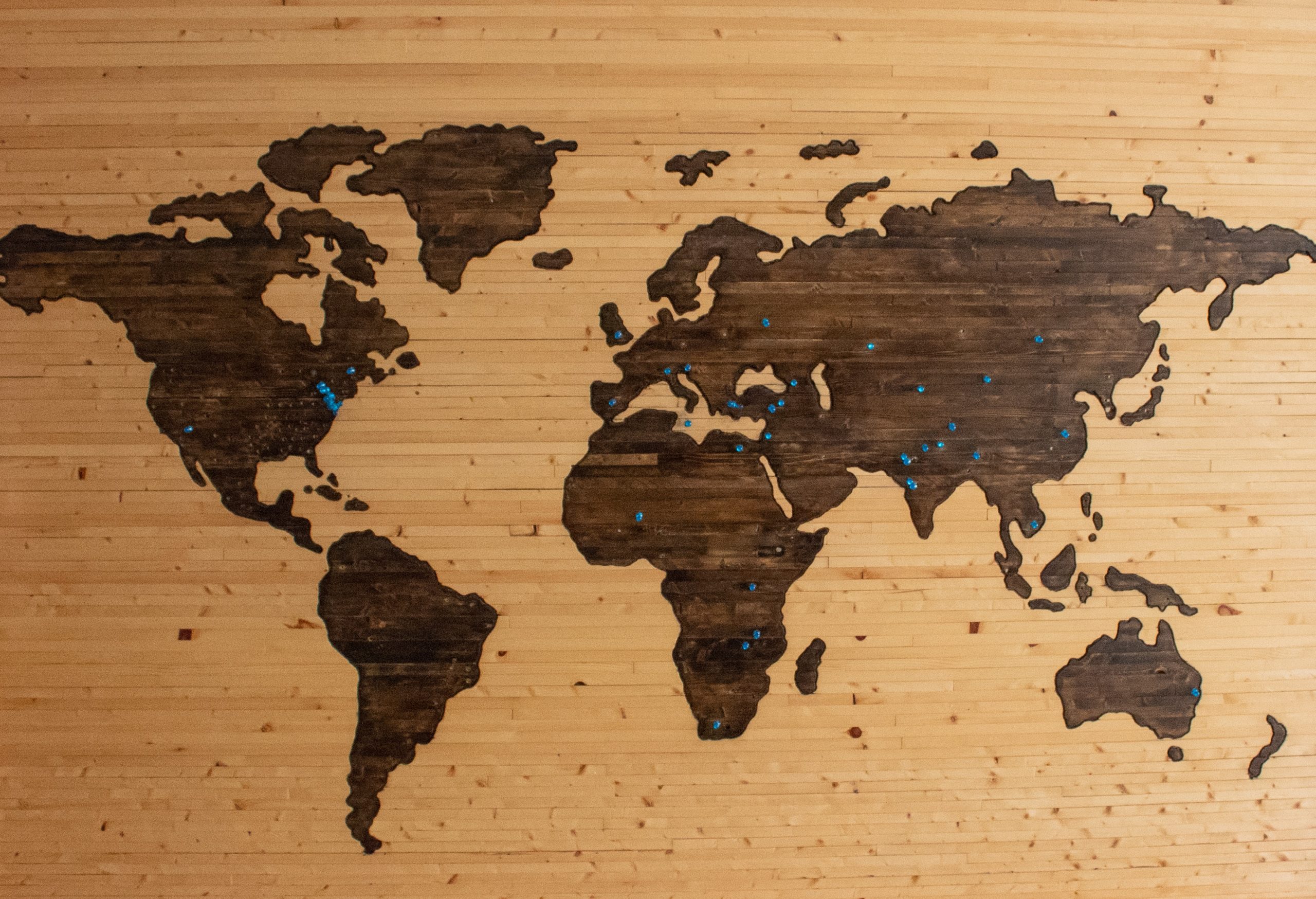
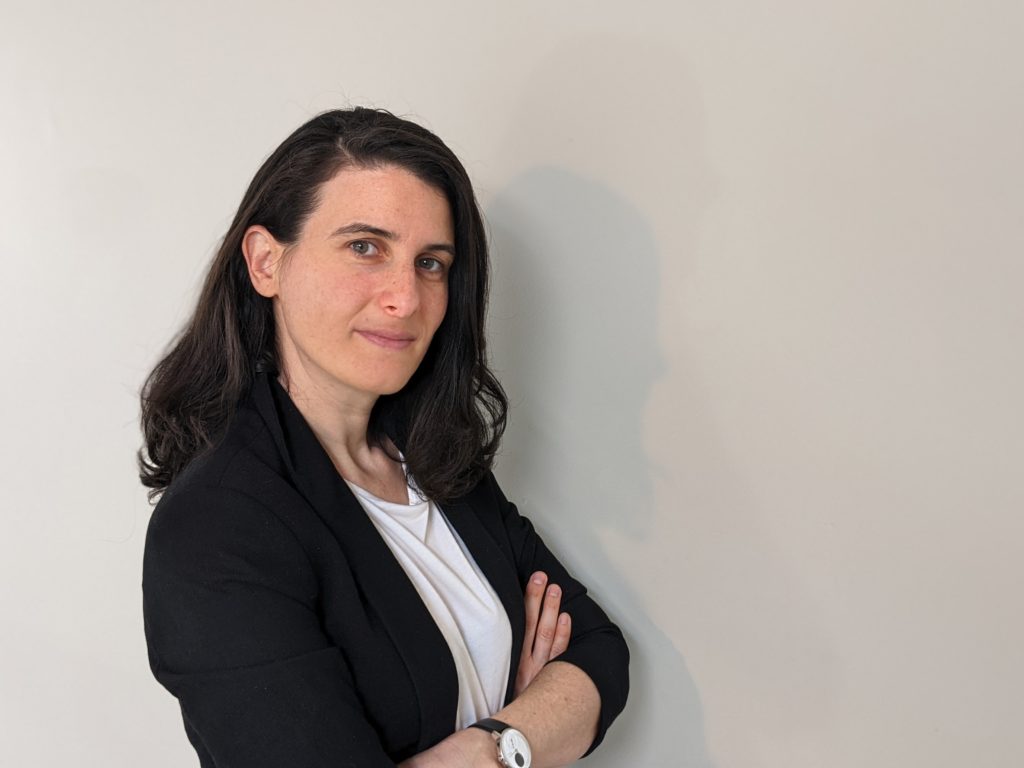
🔬 Original article by Ravit Dotan, who does research in AI ethics, impact investing, philosophy of science, feminist philosophy, and their intersections. She is currently a postdoc at the Center for the Philosophy of Science at The University of Pittsburgh, and she got her Ph.D. in philosophy from UC Berkeley..
With the rise of AI and the recognition of its impacts on people and the environment, more and more organizations formulate principles for the development of ethical AI systems. There are now dozens of documents containing hundreds of principles, written by governments, corporations, non-profits, and academics. This proliferation of principles presents challenges. For example, should organizations continue to produce new principles, or should they endorse existing ones? If organizations are to endorse existing principles, which ones? And which of the principles should inform regulation?
In the face of the proliferation of AI ethics principles, it is natural to seek a core set of principles or unifying themes. The hope might be that the core set of principles would save organizations from reinventing the wheel, prevent them from cherry-picking principles, be used for regulation, etc. In the last few years, several teams of researchers have set out to articulate such a set of core AI ethics principles.
These overviews of AI ethics principles illuminate the landscape. In addition, they highlight the limitations of the search for unifying themes. They help us see that it is unlikely that a unique set of core principles will be found. And that, even if it is found, universally applying it runs the risk of exacerbating power imbalances.
Five overviews of AI ethics principles
Let’s start with reviewing five studies that overview the landscape of AI ethics principles. What is their methodology? And what unifying themes do they identify?
1. The Global Landscape of AI Ethics Guidelines, by Anna Jobin, Marcello Lenca, and Effy Vayena (2019, read the paper here)
Jobin et al. conducted an extensive search and identified 84 papers producing AI ethics principles. The inclusion criteria were as follows: (i) The paper is written in English, German, French, Italian, or Greek. (ii) The paper was issued by an institutional entity. (iii) The paper refers to AI ancillary notions explicitly in its title or description. And (iv) the paper expresses a moral preference for a defined course of action.
The team used manual coding to identify unifying themes and came up with 11 of them: transparency (appeared in 87% of the documents), justice and Fairness (81%), non-maleficence (71%), responsibility (71%), Privacy (56%), beneficence (49%), freedom and autonomy (40%), Trust (33%), sustainability (17%), dignity (15%), and solidarity (7%).
While there is convergence on principles, Jobin et al. point out that there is divergence in how the principles are interpreted, why they are deemed important, and how they should be implemented.
2. A Unified Framework of Five Principles for AI in Society, by Luciano Floridi and Josh Cowls (2019, read the paper here)
Floridi and Cowl identify six high-profile and expert-driven AI ethics documents. The selection criteria were as follows: (i) The document was published no more than three years before the study. (ii) The document is highly relevant to AI and its impact on society as a whole. And (iii) the document is highly reputable, published by an authoritative and multi-stakeholder organization with at least national scope. In searching for unifying themes in AI ethics principles, the authors draw from the four ethical principles commonly used in bioethics: beneficence, non-maleficence, autonomy, and justice. They identify these same themes as unifying themes for AI ethics principles, and they add a fifth one: explicability.
3. Linking Artificial Intelligence Principles, by Yi Zeng, Enmeng Lu, and Cunqing Huangfu (2019, read the paper here)
Zeng et al. collected 27 proposals of AI ethics principles and grouped them by background: (i) Academia, non-profits, and non-governmental organizations, (ii) government, and (iii) industry. The authors extracted principles from each text and tracked common themes using a keyword search. They started by choosing ten keywords as core terms: humanity, collaboration, share, fairness, transparency, privacy, security, safety, accountability, and AGI (artificial general intelligence). After identifying these core terms, Zeng et al. computationally expanded them, creating lists of related words and expressions. For example, the “accountability” theme was expanded to include “responsibility.” Zeng et al. then performed keyword searches for all the words on the lists, thereby discovering the frequency of appearance of each theme.
The team found that the prominence of each theme depends on the background of the document:
- Corporations: The top three themes are humanity, collaboration, fairness, transparency, safety. They mention privacy and security much less than the other institutions and mention AGI and collaboration much more.
- Governments: The top themes are privacy, security, humanity. They mention accountability much less than the other kinds of institutions.
- Academia, non-profits, and non-government: The top categories are humanity, privacy, accountability. They mention humanity much more than the other kinds of institutions.
4. Principled Artificial Intelligence: Mapping Consensus in Ethical and Rights-based Approaches to Principles for AI, by Jessica Fjeld, Nele Achten, Hannah Hilligoss, Adam Christopher Nagy, and Madhulika Srikumar (2020, read the paper here)
Fjeld et al. analyzed 36 documents. The selection criteria were as follows: (i) The document represents the views of an organization or institution. (ii) The document was authored by relatively senior staff. (iii) In multi-stakeholder documents, a breadth of experts were involved. (iv) The document was officially published. And (v) the document was written in English, Chinese, French, German, or Spanish.
The authors extracted ethical themes from these documents by manual coding, resulting in eight themes: fairness and non-discrimination (appeared in 100% of documents), privacy (97%), accountability (97%), transparency and explainability (94%), safety and security (81%), professional responsibility (78%), human control of technology (69%), and promotion of human values (69%).
The paper recognizes that other teams of researchers may identify different themes. It also points out that, while there is a convergence on the themes, the principles are implemented differently in different documents.
5. The Ethics of AI Ethics: An Evaluation of Guidelines, by Thilo Hagendorff (2020, read the paper here)
Hagendorff analyzed 22 major ethical guidelines. The selection criteria were as follows: (i) The document was published no more than three years before the study. (ii) The document refers to more than a national context or has significant international influence. (iii) The document addresses AI ethics generally, not on specific aspects of AI. (iv) The principles are not corporate policies unless they have become well-known through media coverage.
Hagendorff identified eight themes: privacy protection (appeared in 82% of documents), fairness, non-discrimination, justice (82%), accountability (77%), transparency/openness (73%), safety, cyber-security (73%), common good, sustainability, well-being (73%), human oversight, control, auditing (54%), and solidarity, inclusion, social cohesion (50%).
Hagendorff also identified that most of the authors of the documents were men and that only one document included notes on the technical application of the principles, and even those were few and limited.
Limitations of the search for unifying themes in AI ethics principles
1. Is it likely to identify a unique set of core AI ethics principles?
As you can see, the different overviews resulted in different sets of unifying themes. Such differences are expected since the overviews differ on their choice of documents, methodology, and application of methodology.
What shall we do with the resulting multiplicity of unifying themes? One approach is to seek unifying themes in the proposed unifying themes. The hope might be to identify the “core” of the core AI ethics principles. However, it seems unlikely that such efforts will yield a unique set. We will once again need to ask: Which sets of unifying themes should be included? Which methodology should be chosen? And how should it be applied? Just as different overviews of AI ethics principles produced different unifying themes, it is likely that overviews of the overviews will produce different sets of “unifying unifying themes.”
Therefore, finding a unique set of core AI ethics principles seems unlikely.
2. Suppose that a core set of principles were to be found, should it be universally adopted?
Even if a core set of AI ethics principles were to be found in the existing AI ethics principles, universally adopting it is problematic because of the lack of diversity in the perspectives that generated the principles.
To start, the vast majority of the existing AI ethics documents were written in North America and Europe, as some of the overviews highlight.
Moreover, even within the global north, the perspectives that are represented in the existing AI ethics documents are limited. As Hagendorff identified, the documents were written by men for the most part. We do not have statistics on the participation of other relevant identity categories, such as race, religion, and sexual orientation. However, the authors of the AI ethics documents are probably relatively homogenous along these axes as well.
Further, the voice of those impacted by AI systems is likely to be underrepresented. Zeng et al. suggest that AI ethics documents might reflect the interests and needs of the institutions that authored them. For example, Zeng et al. show that corporations mention privacy and security less than other types of institutions. The reason might be that privacy and security are sensitive topics for them. Similarly, governments mention accountability less, and academia, non-profits, and non-governmental organizations mention collaboration less. The reason might be that these are sensitive topics for them. Which institutions represent the interests and needs of the broader, global public impacted by AI systems? How influential are they in the production of AI ethics principles?
Given the lack of diversity in the perspectives involved in generating AI ethics principles, they seem to represent the preferences and interests of a selected few. If a core set of principles were to be found among them, it would represent these selected few as well. Therefore, universally adopting unifying themes found in the existing AI ethics principles would run the risk of subjugating broad populations to principles that were formulated by a small elite, thereby exacerbating existing power imbalances.
What’s next?
Overviews of existing AI ethics principles help us see that it is unlikely that a core set of principles will be found and that, even if it were to be found, universally adopting runs the risk of exacerbating power imbalances. That brings us back to the questions with which we started. How do we navigate the proliferation of AI ethics principles? What should we use for regulation, for example? Should we seek to create new AI ethics principles which incorporate more perspectives? What if it doesn’t result in a unique set of principles, only increasing the multiplicity of principles? Is it possible to develop approaches for AI ethics governance that don’t rely on general AI ethics principles?