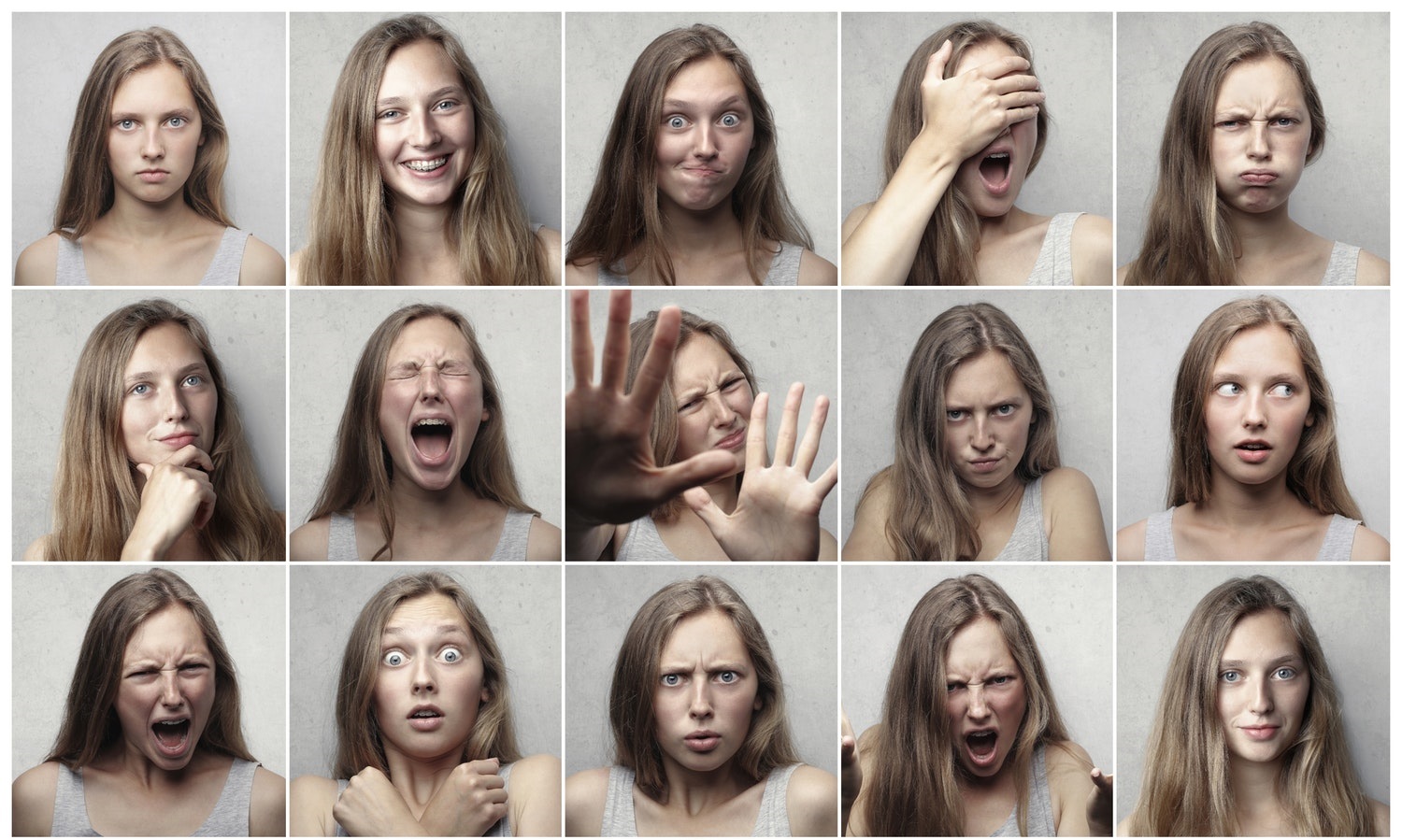
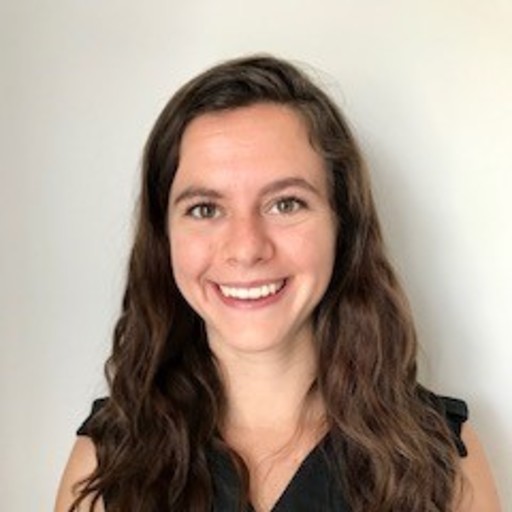
Summary contributed by our researcher Alexandrine Royer, who works at The Foundation for Genocide Education.
*Link to original paper + authors at the bottom.
Overview: In this summary paper, Luke Stark and Jesse Hoey, by drawing on the dominant theories of emotion in philosophy and psychology, provide an overview of the current emotion models and the range of proxy variables used to design AI-powered emotion recognition technology. The disjuncture between the complexity of human emotion and the limits of technical computation raises serious social, political, and ethical considerations that merit further discussion in AI ethics.
From benevolent Wall-E to femme fatal Ex-Machina, the emotional lives of intelligent machines have long been a subject of cinematic fascination. Even as early as Mary Wollstonecraft Shelley’s 1818 Frankstein, human imagination foresaw the advent of sentient and humanoid machines. With increasing machine learning sophistication that can drastically err from its makers’ original intentions (we’re looking at you Tay.Ai), Shelley’s 200-year-old metaphor of human genius leading to monstrous results still rings true. While we are still far from being menaced by emotionally intelligent humanoid robots, such fictional characters raise crucial questions concerning the range of human emotions, how we conceptualize them, and how they can be measured and input into intelligent systems. The next Frankenstein, rather than a terrifying green mechanical monster, will likely be an invisible AI system that recognizes and feeds off your emotions.
Indeed, AI technologies that predict and model human emotions to signal interpersonal interactions and personal preference are increasingly being used by social media platforms, customer service, national security, and more. In this paper, Stark and Hoey present the range of models and proxy data used for emotional expression and how these are combined in artificial intelligence systems. They argue that the “ethics of affect/emotion recognition, and more broadly so-called digital phenotyping ought to play a larger role in current debates around the political, ethical and social dimensions of AI systems.”
The conversation begins with choosing between the different theories of emotion and the range of phenomenon that the term can encapsulate, from the physiological, expressive, behavioural, mental, etc. When describing the difficulties of emotion modelling, the authors cite philosopher Jesse Prinz’s “problem of parts” – selecting the essential components of emotion- and “problem of plenty”- determining how these components function together. In psychology, emotion is distinguished from affect, described by psychologist Jerome Kagan as a “change in brain activity” and by affect theorist Deborah Gould as “nonconscious and unnamed, but … registered experiences of bodily energy response to a stimulus”. Affect is considered an immediate bodily response, while emotion is a much more complex cognitive process that responds to changes in affect.
Stark and Hoey list the different camps in the conceptual modelling of emotion, choosing to define emotions as:
- Experienced feeling states (Experiential theories). The feeling tradition emphasizes the “feeling” component and embodied sensations of emotions and defines them as distinctive conscious experiences. By concentrating on the felt dimension of emotions, their perception and detection are derived from physiological processes.
- Evaluative signals connected to other forms of human perception of social cues (Evaluative theories). Evaluative theories, emerging out of the 1960s cognitive turn, view emotions primarily as cognitive phenomena and ascribe to them a certain degree of intentionality connected to human judgment (i.e. humans direct our emotions towards objects).Â
- As intrinsically motivating desires/”internal cause of behaviours aimed at satisfying a goal” (Affective science). This perspective of emotion as “motivating states” draws from American psychologist Silvan Tomkins, who argued that nine innate affects are hardwired into humans. Paul Eckman, a student of Tomkins, pushed this argument further in the 1970s by developing the Basic Emotion Theory (BET) which posits that are universally identifiable bodily signals, such as facial expression, for each basic emotion. BET has received sustained criticism for its reductionist view of human emotional states.Â
As the authors point out, the boundaries between these three camps are not always so clear-cut, with several philosophical and psychological theorists engaging in what the authors term “hybrid approaches”, whereby human emotions involve a mix of physiological, affective, and social signals. In contrast, in computer science, affect and emotion are often treated interchangeably due to technical constraints. Computer scientists detect what they consider to be evidence of human emotions by tracking biophysical signals such as facial expression, gait, heart rate, or blood conductivity, hence conflating affect with emotion. In addition to a lack of consensus around what emotions are, there is a lack of agreement over the empirical evidence used- in this case, proxy data- to identify human emotions. Depending on the emotional model favoured by the computer scientists, the proxies for emotional expression may include what is referred to as physiological data (e.g. facial expressions), haptic and proprioceptive data (e.g. blood flow), audio data (e.g. tone of voice), behavioural data gathered over time and semantic signifiers (e.g. emojis).
Stark and Hoey explain that the popularity in computer science of correlating bodily changes with emotional changes is partly due to Rosalind Picard’s famous publication Affective Computing which argued for treating human emotional expressions as digitizable information. Picard’s work was criticized for ignoring the dynamism of human social and emotional relationships, with emotions often being culturally constituted. Nonetheless, the majority of “emotional AI” technologies on the market are built on Basic Emotion Theory, which, as stated above, emphasizes a traceable and universal view of emotions that are readily legible through bio-signals such as facial expressions, heart rate, voice recognition, etc. Even though it has a shaky scientific grounding, BET is more amenable to adoption in AI systems. Emotion recognition based on facial expressions in facial recognition technology continues to attract strong commercial interest. It has also enabled the creation of digital phenotyping, where large-scale computation extracts any manifestation of human emotion, whether in keyboard interaction or speech, and turns these into patterns of behaviour that reveal inner motivations. Not all emotional recognition system attempt to grasp our internal affective states, and instead are built to observe social displays of emotion, with Facebook’s “reactions” icons being a case in point.
Despite BET’s predominance, Stark and Hoey note that other emotional models are present in AI systems. One notable example is the Bayesian Affect Control Theory (BayesAct), a quantitative method designed by sociologists and computer scientists to capture through external observation and map out the range of interactions between a user and fellow actors, behaviours and settings. BayesAct combines sociological theory on cultural behavioural norms with the Bayesian probabilistic decision theory to predict what emotions are appropriate in a given situation and help guide users’ actions towards socially normative behaviours. Such systems are being used in cognitive assistance technologies to assist individuals who have dementia, for example. In terms of evaluative theories of emotion, other algorithmic learning models include the Computational Belief and Desire Theory of Emotions (CBDTE) and the Emotion-Augmented Machine Learning (E-A ML).
With much of the emotional AI (EAI) field being left unsupervised by regulators, the authors refer to actors such as Andrew Shay and Pamila Pavlisack, who have issued a set of guidelines for the ethical use of EAI. They warn against current EAI assumptions by stressing that “physical display of emotion is only one facet of emotion,” that there are “stereotypes and assumptions about emotions that materially affect a person or a group” and that “past expression does not always predict future feeling.” Not only may EAI systems be unwanted and overly invasive, but they also raise broader concerns around the use of data for tracking, profiling, and behavioural nudging. Such considerations go against the narrower definitional discourse of emotion in computer science and run contrary to social media giants’ business philosophy, whose profit model rests on manipulating users human emotion to get users to engage and interact on the platform. When touching on the divergence of opinion on emotions, the authors underline that recognizing this diversity of thought in the abstract is “unhelpful without considering how those differences might implicate AI design and deployment decisions, with their attendant ethical valences and social effects.”
There will always be a gap between the emotions modelled and the experience of EAI systems. Addressing this gap also implies recognizing the implicit norms and values integrated into these systems in ways that cannot always be foreseen by the original designers. With EAI, it is not just a matter of deciding between the right emotional models and proxy variables, but what the responses collected signify in terms of human beings’ inner feelings, judgments, and future actions. As Stark and Hoey warn, the AI systems used to analyze human faces, bodies, and gaits are dangerous digital revivals of the widely-discredited 19th and 20th pseudoscience of physiognomy, where a person’s character can be based solely on their outward appearance. From my own anthropological background, BET-based systems run counter to decades of ethnographic research that affirm the cultural specificity of human emotions and the danger of imposing a heterogeneous view on the human experience. Another serious ethical concern is the fact that individual users, often unknowingly, are the subject of human emotion subject research. There is also a self-realizing dimension to EAI systems, as individuals may, over time, modulate their emotional responses to fit the norms produced by these systems.
To conclude, as stated by the authors, “human emotion is a complex topic, and analysis of its effect and impacts in AI/ML benefit from interdisciplinary collaboration.” As a social scientist, I cannot overemphasize the critical need for cross-disciplinary discussion on EAI and the contexts in which it is ethically permissible to develop and deploy these systems. As it is currently developing, the reductionist view of computing emotions in AI systems may create terrifying monsters indeed.
Original paper by Luke Stark, Jesse Hoey: https://osf.io/9ad4u