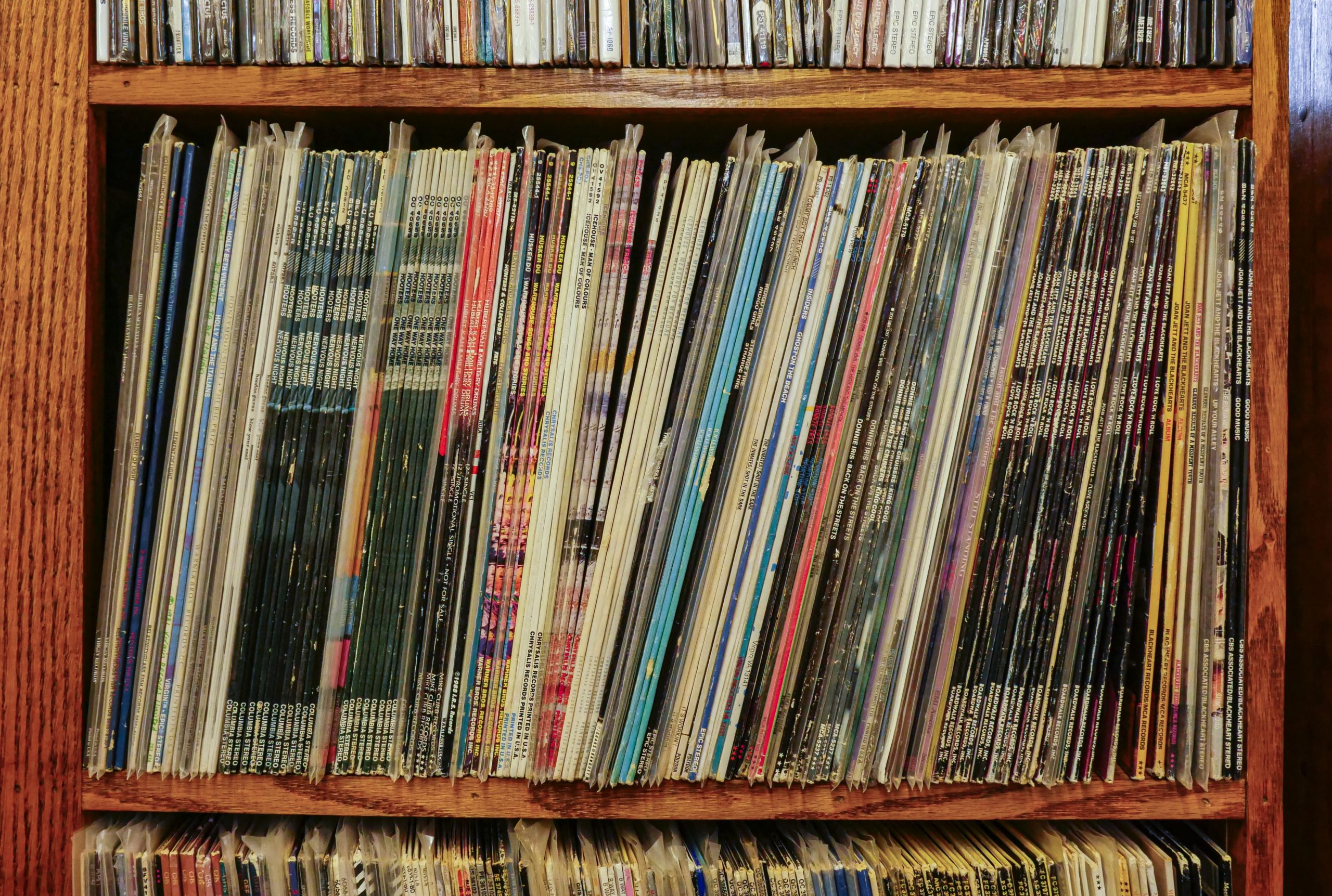
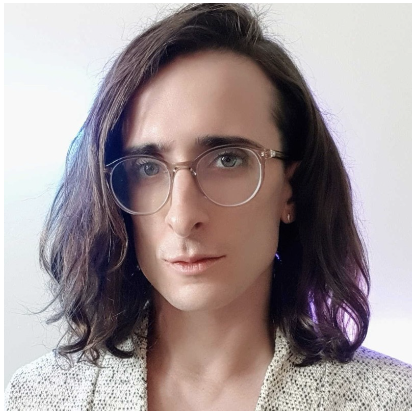
🔬 Research Summary by Blair Attard-Frost, a PhD Candidate at the University of Toronto’s Faculty of Information studying the governance and ethics of AI value chains.
[Original paper by Blair Attard-Frost, AndrĂ©s De los RĂos, Deneille R. Walters]
Overview: AI ethics guidelines tend to focus on issues of algorithmic decision-making rather the ethics of business decision-making and business practices involved in developing and using AI systems. This paper presents the results of a review and thematic analysis of 47 AI ethics guidelines. We argue that in order for ethical AI to live up to its promise, future guidelines must better account for harmful AI business practices, such as overly speculative and competitive decision-making, ethics washing, and corporate secrecy.
Introduction
AI ethics guidelines are vital tools in prescribing principles and practices to mitigate the potential harms of AI systems. However, current guidelines focus predominantly on the technical components involved in automated decision-making, such as a system’s data inputs, classification categories, and model characteristics. Recent controversies in the AI industry such as the firing of AI researchers Timnit Gebru and Margaret Mitchell from Google’s ethical AI team indicate that without greater attention to the business practices surrounding AI—such as the resourcing of AI systems, the recruitment and management of technical staff, marketing, sales, and investment—AI ethics guidelines will be unable to genuinely guarantee that an AI system is operating ethically.
To provide a better account of the ethical issues associated with AI business practices, this paper reviews and conducts a thematic analysis of 47 AI ethics guidelines published by governments, intergovernmental organizations, industry, civil society, and multistakeholder groups. In discussing our findings, we highlight four harmful AI business practices that we observed most often in the reviewed guidelines: (1) unempirical business decision-making based on speculative socio-economic benefits of AI, (2) ethics washing for competitive purposes, (3) unsustainable value extraction, and (4) selective corporate transparency and communication.
Key Insights
The Business Practices of AI
As AI ethics has continued to emerge as a field of research and practice in recent years, researchers and practitioners have increasingly noted that AI ethics guidelines tend to overlook ethical issues related to the political economies and business contexts in which AI systems are developed and used. Observing this shift in AI ethics beyond the technical context of algorithmic decision-making, we comment that “algorithms do not operate in a petri dish—they are always socially and economically situated.” We demonstrate this point by backgrounding our study against a variety of perspectives on the political economy of AI ethics, including discussions of the capitalist economic logics of AI-based business models as well as critiques of the exploitative and extractive practices that AI systems enable.
We unify all of these diverse, emerging perspectives on the political economy of AI ethics under the conceptual banner of “AI business practices,” which we define as “the iterative political and economic behaviors involved in the organized resourcing, design, development, deployment, and use” of an AI system. Noting that the ethics of AI business practices are consistently seen as a significant gap in AI ethics by many other researchers, we set out to more closely observe “how AI business practices, their political and economic effects, and ethical dimensions of that political economy are treated in a selection of prominent AI ethics guidelines.”
The Ethics of AI Business Practices: Key Themes
We structure our analysis by flipping the script on four principles that are commonly discussed in the AI ethics: fairness, accountability, sustainability, and transparency. We then analyze the degree to which the 47 guidelines account for those principles in issues of AI business practices rather than issues of automated decision-making. We find 11 key themes emphasized throughout the reviewed guidelines that directly pertain to those four principles:
Fairness of AI Business Practices
1. Open Innovation: Guidelines that discuss this theme observe “the importance of creating a fair environment in which businesses and governments can share data and intellectual property in order to develop new AI products and services.”
2. Market Fairness: Guidelines that discuss this theme “focus on the role of governments in reducing outsized power disparities between businesses and promoting fair competitive practices” in the marketplace.
3. Bias & Diversity in Professional Practices: Guidelines that discuss this theme center issues of fairness, bias, diversity, and inclusivity in professional practices such as recruitment, business operations, management, marketing, software development, and product design.
Accountability of AI Business Practices
4. Public Perception of AI Business Practices: Guidelines that discuss this theme “express concern that without appropriate accountability mechanisms, the public may feel anxious about or lack trust in the business context” of AI development and use.
5. Internal Oversight of AI Business Practices: Guidelines that discuss this theme attend to “the methods through which organizations can account for and assure ethical conduct” in developing and using AI systems, such as internally mandated social impact assessments and audits, internal review boards and ethics bodies, and worker involvement in managerial decision-making.
6. External Oversight of AI Business Practices: Guidelines that discuss this theme attend to “the methods through which the general public and public governments can bring organizations to account for their conduct,” such as legal and regulatory frameworks for AI, government intervention and incentivization in AI values chains and markets, or creation of standard-setting initiatives.
Sustainability of AI Business Practices
7. Sustainable Development: Guidelines that discuss this theme show interest in “principles and practices for developing and maintaining multistakeholder systems for adaptive AI governance and AI innovation policy,” such as implementing education, training, and business development programs in order to support the short-, medium-, and long-term knowledge and governance needs of the AI industry.
8. Management & Distribution of Benefits and Harms: Guidelines that discuss this theme show interest in responsibly managing business practices so as to produce greater benefit and lesser harm in the short-, medium-, and long-term through methods such as more directly involving vulnerable stakeholder groups in business decision-making.
Transparency of AI Business Practices
9. Scope of Decision-making Explanation: Guidelines that discuss this theme extend concepts such as transparency and explainability beyond the scope of algorithmic decision-making, highlighting the importance of some degree of transparency in also explaining the business processes and rationales involved in developing and using AI systems.
10. Transparent Business Practices & Cultures: Guidelines that discuss this theme encourage the cultivation of an industry-wide culture of transparency beyond algorithmic decision-making, such as transparency in research findings, risk assessments, community consultations, and procurement processes. 11. Documentation, Disclosure, & Selective Transparency: Guidelines that discuss this theme show interest in the specific conditions under which businesses may choose or may be obligated to disclose sensitive business information, such as harmful social impacts of their AI systems, intellectual property, or other specific aspects of business models, processes, or decisions that may arguably constitute “trade secrets.”
Between the lines
In light of our findings, we ultimately advocate for an expansion of the scope of AI ethics. We suggest that future AI ethics guidelines ought to go beyond the predominantly technical scope of current guidelines to “include a broader ontology of business practices, organizational systems, and political-economic considerations.” In practice, this could be achieved by adapting existing design and evaluation approaches to AI business practices, such as broadening algorithmic impact assessments to assess the social and economic impacts of business decisions, applying principles and processes from algorithmic auditing frameworks to auditing AI-based business models, or involving a broader group of stakeholders and communities in making decisions about how AI systems ought to be resourced and managed.
Expanding the scope of future AI ethics guidelines also necessitates expanding the disciplinary scope of AI ethics. Politics, economics, management, culture, and ecology are all at issue in the development and use of AI. To meaningfully describe an AI system as “ethical,” AI ethics guidelines must account for the ethics of AI systems through all of those disciplinary lenses, in addition to the technical lens that so often dominates discussions of ethical AI. Social, political, and economic realities matter. Business context matters. AI systems are always inextricably embedded in economic logics and business practices—AI ethics ought to be, too.