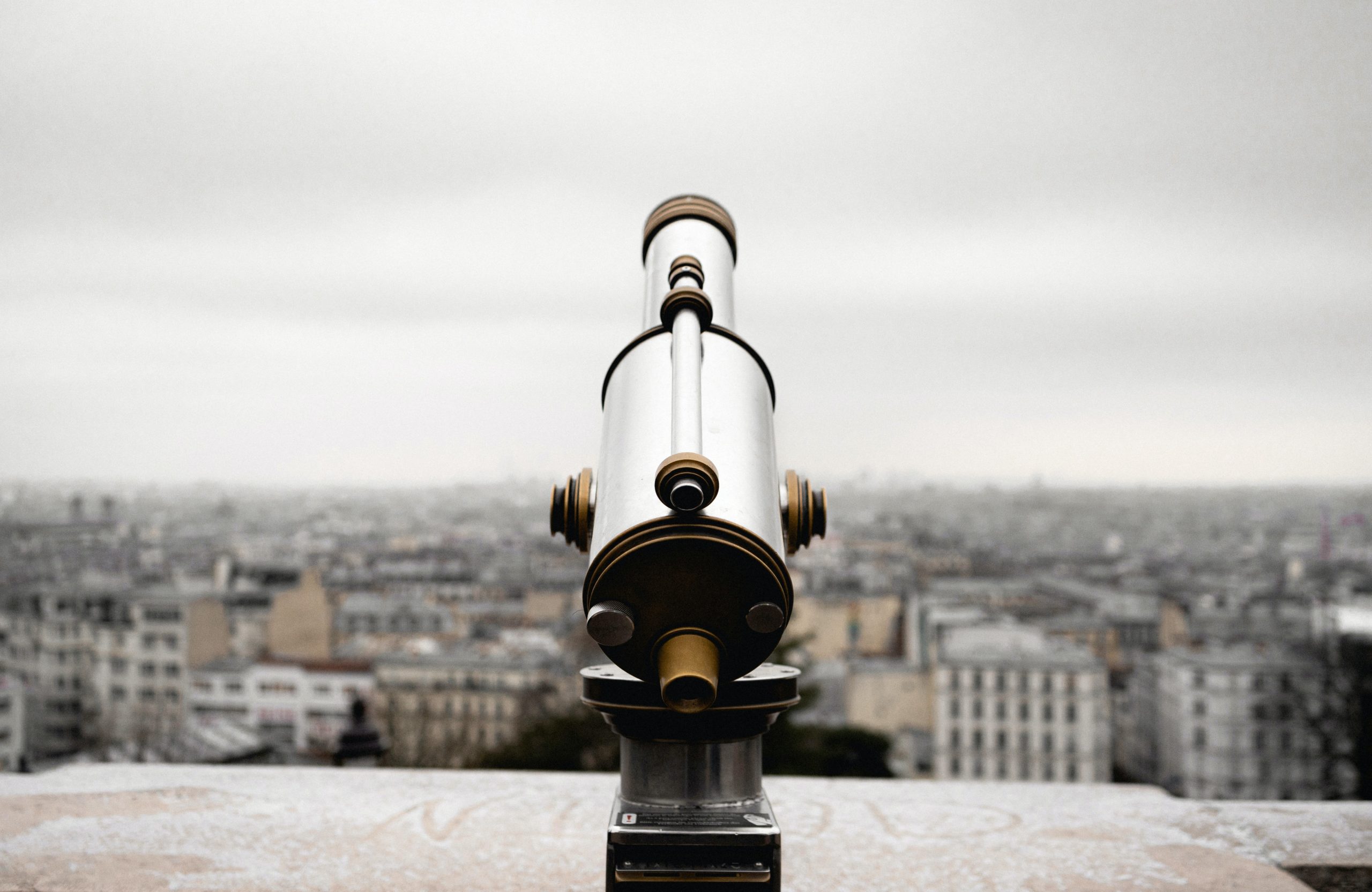
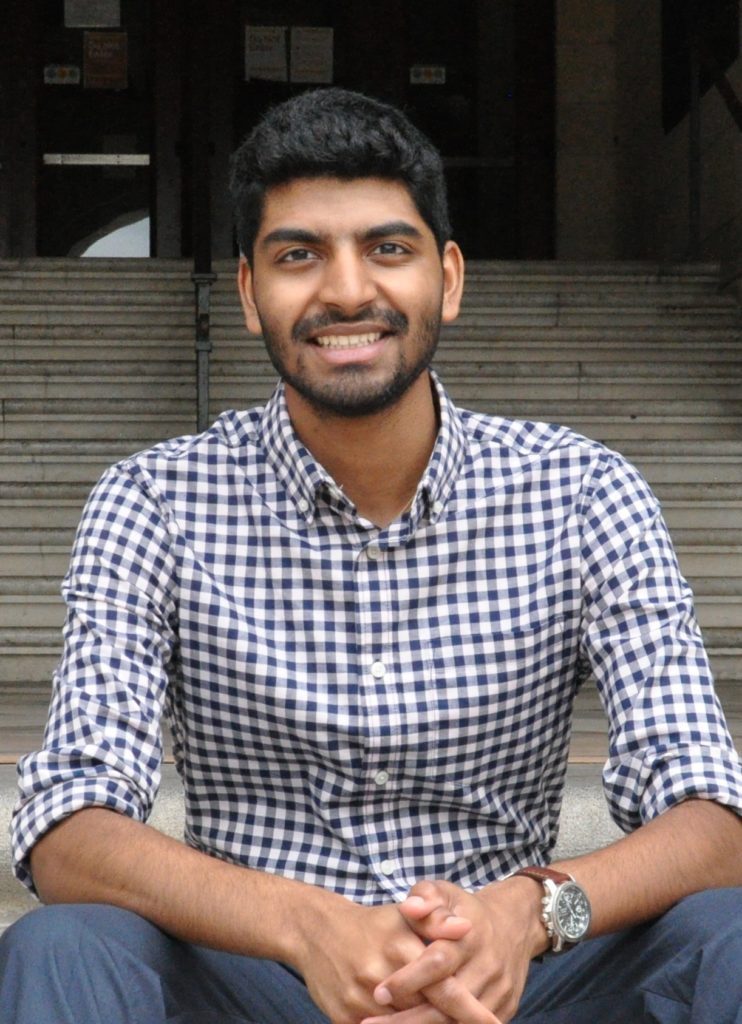
✍️ Original article by Abhinav Raghunathan, the creator of EAIDB who publishes content related to ethical ML / AI from both theoretical and practical perspectives.
This article is a part of our Ethical AI Startups series that focuses on the landscape of companies attempting to solve various aspects of building ethical, safe, and inclusive AI systems.
In our last issue, we talked about the idea that data is a liability and how an entire industry has evolved to help organizations deal with it. Now, we follow the path of data as it continues to the next stage in the AI lifecycle — the model. The governing principle of machine learning models is “garbage in, garbage out.” This phrase was coined in the 1950s to describe the idea that outputs are no more than the quality of their inputs but this maxim is still critically relevant today in the machine learning and AI realm.
However, it is not just data that carries biases with it. It has been shown that models have bias too and contribute to a phenomenon known as “bias amplification,” in which the model amplifies unfairness in the data so that the output is even less fair than the input. This leads to the mission-critical question of how do we monitor and observe our models? How can we mitigate these risks and ensure a level of safety and responsibility when the models we use are (ironically) so unpredictable?
Enter the ModelOps, Monitoring, and Observability category in the Ethical AI Database (EAIDB). Startups within this designation have functions across the board — they monitor models, detect bias and unfairness, mitigate risks, and communicate between multiple teams. Some companies in this subcategory are Fiddler, Arthur, ETIQ, and KOSA. Others provide model governance and access to all stakeholders to bridge the gap between legal, business, and data science teams. Apres and 2021.AI are good examples.
Growth in the number of companies in EAIDB’s “MLOps, Monitoring, and Observability” space.
TRENDS
Finance and healthcare are launchpads for promising ethical MLOps companies.
Unlike most other fields, finance and healthcare have a rigorous set of standards already in play. In the United States and Europe, there are very well-defined regulations that dictate what institutions can and cannot do in a way that does not exist in other fields (like hiring, for example). Startups are using these better-defined areas to grow their potential, almost like an incubation phase. “There’s just more demand in these areas,” Rasmus Hauch, CTO of 2021.AI says, “and from an AI perspective, use cases are generally considered ‘riskier,’ which make them more critical to solve.” Selected clients from some MLOps, Monitoring, and Observability startups featured on EAIDB.
Ethical MLOps companies are expanding horizontally to meet demand.
“Pure MLOps” is quickly becoming a concept of the past. Even the incumbents in DataRobot, Amazon AWS, etc. are modifying their products to appeal to those who care about features with bias detection and monitoring. However, the approaches taken by these companies are rarely special — they are more of post hoc add-ons to products that have already dominated their niche.
Because this is a very fragmented space and there are many, many players for what is, quite honestly, a limited demand, modern startups like those in EAIDB are pivoting. 2021.AI has moved towards a slightly more GRC-related angle. Netherlands-based KOSA AI has functionality that covers everything from data bias and observability to post-model governance. These companies can be dubbed “MLOps+” because their core function is still within the scope of MLOps, Monitoring, and Observability, but their product and service lines are much more multidimensional. This response is a method of product differentiation — a sound strategy in such a nascent and competitive landscape.
Established companies that have added bias mitigation and detection features within the last five years.
“Here in the EU,” Layla Li, CEO of Netherlands-based KOSA AI says, “there is next-to-no demand for ML monitoring alone.” KOSA markets its compliance angle to sell its product, but companies often come back for other features like their data observability.
Client investment will shift from solving specific ethical AI issues to having the entire ethical AI pipeline.
In the current climate, investing in the whole nine yards of ethical AI (from data sourcing to model deployment to governance and beyond) is somewhat of a tall order for companies across the AI landscape, especially when the need for such products is still not widely understood.
Hauch says, “clients want solutions to very specific issues right now, not necessarily the entire ethical pipeline.” 2021.AI provides an end-to-end approach to MLOps and GRC from data creation and ingestion to operational aspects and even termination. As time passes and the need for specific solutions across the entire pipeline becomes more pressing, unified solutions like 2021.AI or KOSA AI may become the norm.
EAIDB’s “Auditing, Governance, Risk, and Compliance” category (discussed in our next article) features a host of consulting firms that are experts at solving these “specific issues,” but are not currently built to provide entire pipelines. However, these firms are also human-driven, which makes them very nimble in what is a changing geopolitical landscape. How the demand for both consulting firms and automated platforms changes into the future as demand increases and policy is reshaped will be a fascinating topic to watch.
BARRIERS
Drastic differences in global AI market maturity.
There is a very large disparity between the “AI powers” of today’s world. In the United States, AI is a mature market. Most organizations employ AI to some degree and the pain points of AI are well-known. However, the United States is historically very slow to react on the regulatory side, which is one of the main drivers of ethical AI stack adoption.
Contrastingly, the EU’s AI market is very immature — banks and other institutions are just now exploring what AI has to offer. As Li puts it, “pain points in the EU are lesser known because the same demand for solutions does not exist in Europe quite yet.” Even so, the EU is currently a leader in AI policy and this regulation will create its own demand. “Regulation will be a driver,” Li maintains, “companies will flock to the ethical AI scene when Europe’s AI Act and similar policy forces their hand. A similar turn of events happened when GDPR was introduced.”
There are difficulties in both markets from different sides of the equation. In the United States, customers are hard-won because there’s no regulatory pressure moving them. In the EU, there is pressure, but clients are less familiar with AI and its governance. Some companies, like Apres, operate in both environments and are therefore in constant contact with these odds.
Countries ranked by key components of AI-readiness, research by McKinsey & Company.
The communications gap between data science and business / legal teams has never been wider.
Communicating model risk is a task that requires a legal framework, a business context, and data science technicality. Unifying these seamlessly is almost impossible, but is critical to place models in context. How does a data scientist communicate with a lawyer to ensure that a company’s models are compliant? How does a CEO communicate business risks to a data science team? The lack of shared vocabulary throughout the levels of corporate hierarchy hurts all sides in understanding how to create safer and more responsible AI. This problem is complicated to solve for most ethical MLOps+ companies, but also presents an opportunity for the very best.
“This is a problem that flows directly into GRC,” Hauch says, “as a company, our approach is to take legal text and create controls and rules, then translate those into metrics that a data science team can understand.”
Matt Waite, CEO of San Francisco-based Apres, says, “products cannot just work for data scientists. We at Apres strive to create an operating system that connects people of all backgrounds with the model at hand.”
OUTLOOK
We have a fundamental problem in how we approach models under today’s schema. Our data science teams might understand some portions of the business context, but most likely have no knowledge of the legal framework. Lawyers and businesspeople have no insight into how the models work. Stakeholders are left in the dark and the barrier to bring them in is the communication of context via a shared language, which doesn’t currently exist.
More worryingly, the voices working to improve AI are primarily sourced from three regions: the US, the EU, and Canada. Global representation and diverse cultural perspectives are simply non-existent. Add into this equation the idea that over time more and more of society’s predictions will be impacted by AI and the result is a very frightening reality. A reality in which only a handful of people in Western countries with data science backgrounds manage decisions for the rest of the world.
Geographic distribution of startups in EAIDB’s MLOps, Monitoring, and Observability category. North America (NA), Europe + Middle East + Africa (EMEA), Asia Pacific (APAC).
To counter this, startups like 2021.AI are creating a shared language by contextualizing and translating existing legal structures into model metrics, controls, and rules in addition to providing model monitoring services. Apres is providing an operating system that enables all hands across organizations and perspectives to touch the model.
“What’s important to realize,” Waite says, “is that every data science team has some notion of governance already. What’s lacking is the ability to have strong checks and balances and context-conscious solutions.”
In the next issue of this series, we address the “Audits, Governance, Risk, and Compliance” subcategory, in which startups and consulting firms act after models have been applied to manage, detect, and mitigate risk and compliance issues.EAIDB is the first publicly-available database of AI startups either providing ethical services or addressing areas of society historically riddled with bias. Learn more about the mission of EAIDB at https://eaidb.org.