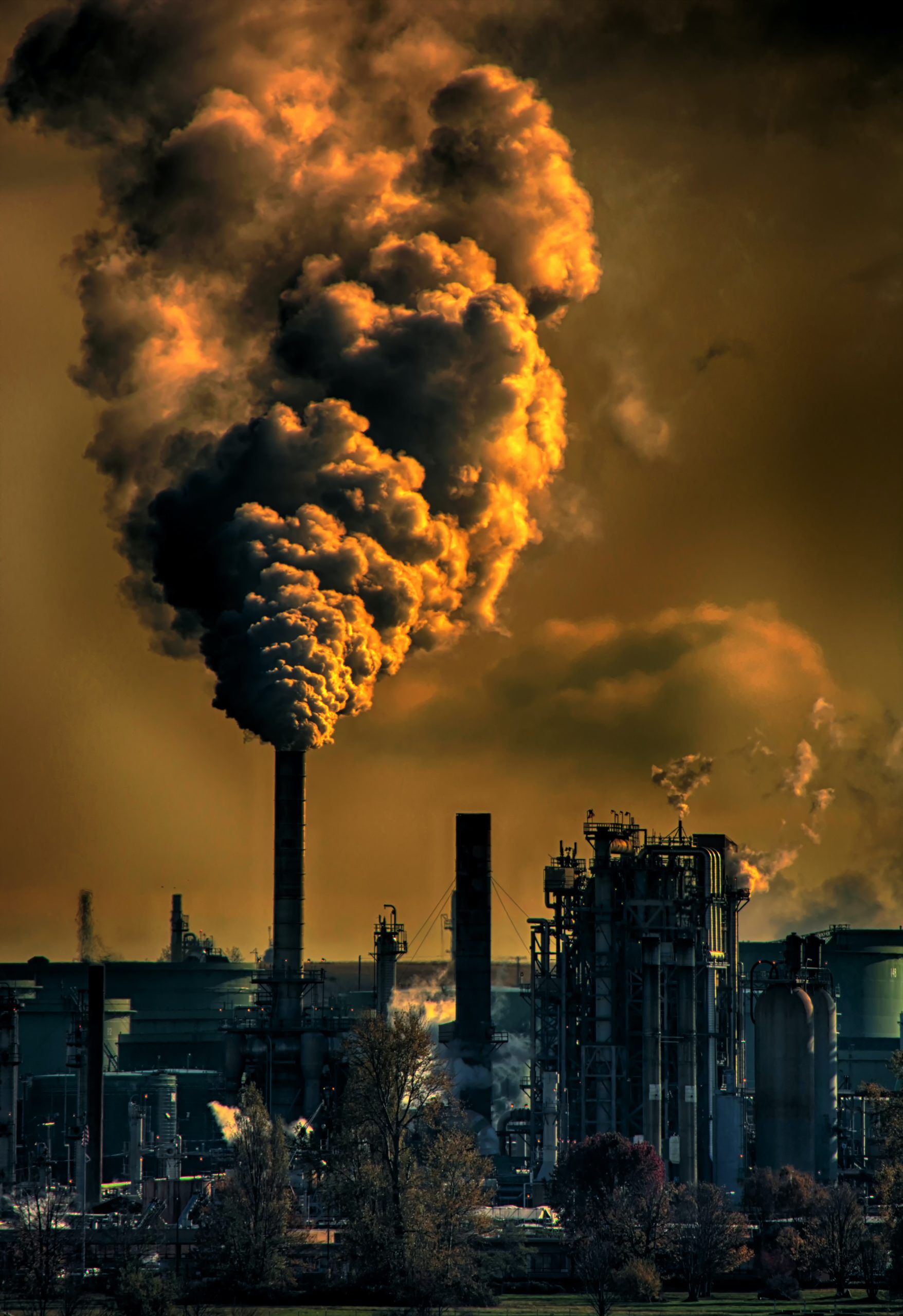
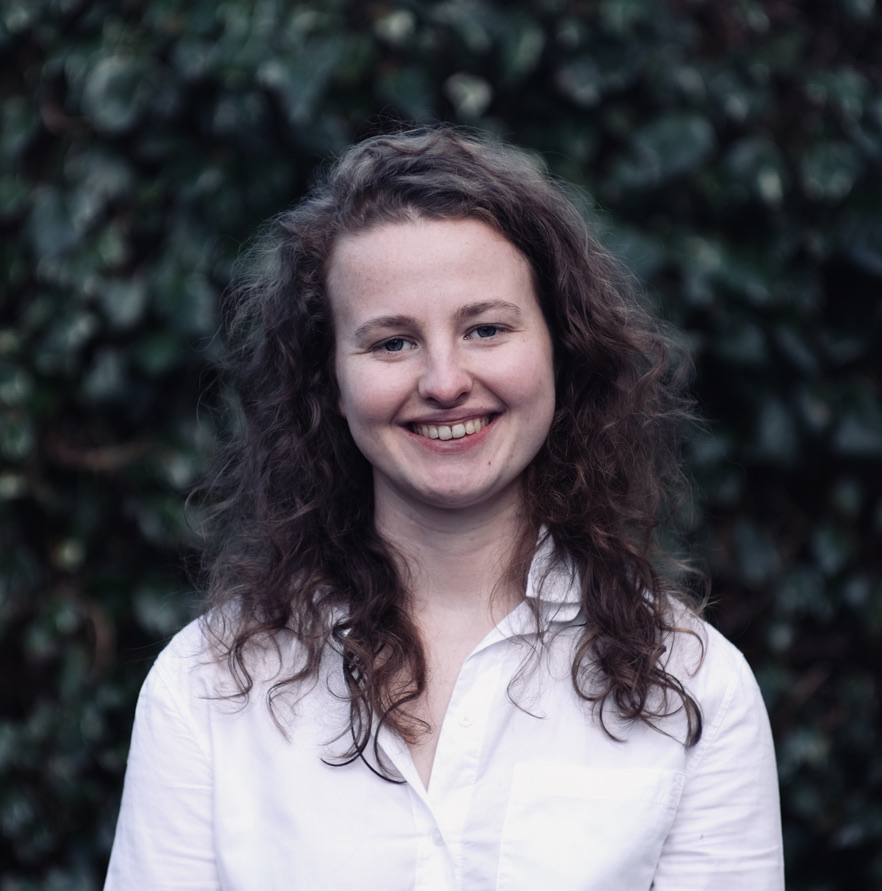
🔬 Research Summary by Eryn Rigley, a PhD research student at University of Southampton, specialising in the intersection of environmental and AI ethics, as well as defence & security AI ethics.
[Original paper by Guglielmo Tamburrini]
Overview:  Tamburrini approaches the critical, yet underrepresented, problem of AI science impact on the environment. He argues that a shift in the metrics by which AI research and development (R&D) success is measured to encompass environmental impact might well provide a means of distributed responsibility for our planet’s wellbeing.
Introduction
The training of an DNN based NLP Transformer model is estimated to emit as much greenhouse gasses (GHG) as five cars over their entire lifecycle. Training a BERT Large NLP model produces as much GHG as a flight from San Francisco to New York. There are ways in which we are taxed or charged to neutralize the carbon footprint of our travel; but what about AI research which clearly has just as significant an impact? Distributed responsibility has been explored significantly in terms of the outputs of AI systems, particularly in ML systems where the often unpredictable output of an AI system can directly impact humans. However, there are other responsibilities AI scientists should consider. In this paper, Tamburrini picks apart the responsibility of AI scientists to environmental protection.
Key Insights
The paper examines the global environmental impact of training machine learning (ML) systems in depth, specifically it’s carbon footprint. Tamburrini outlines the complexities of the global problem of GHG emission in AI R&D, and offers an appropriate and measured solution.
The problem
Tamburrini argues the environmental impact of AI R&D is global. No matter your geographical periphery to a particular AI system, you will be impacted by the carbon emissions that training AI systems generate. However, there are many elements to an AI system’s impact on the environment which makes defining an AI researcher’s responsibility to reducing GHG emissions a complex problem. An interesting point Tamburrini notes is that much of AI in use today is intangible, yet has very tangible effects on the environment. We cannot see or touch a neural network or ML algorithm. Yet we live and breathe the GHG emissions which the training of those systems will produce. As such, the environmental impact of ML systems can be a difficult problem to motivate.
Moreover, the problem of many hands arises. Because up to hundreds of people are involved in the various stages of AI development and deployment, assuming someone else is to blame can be an easy way of shucking one’s personal responsibility within a complex organization. This problem becomes further exasperated when considering the various, sometimes disconnected and discordant, stages of an AI system’s life cycle; from conception, design, research, development, training, deployment, to reflection.
The aim of this paper is to approach this complex web of people involved in the development of AI, to fairly distribute the responsibility of environmental protection within AI R&D.
The solution
Currently, the metrics to measure the success of an AI system exclude any reference to environmental impact. In a random sample of 100 relevant research papers, a resounding zero percent mentioned the carbon footprint of an AI system. Instead, metrics include accuracy and computational resources used (without reference to the environmental impact of these). Alternatively, metrics measure the impact of a ML system on humans, including fairness and bias. Environmental resources used or carbon footprint is not considered when we measure the efficacy of AI.
This paper suggests herein lies the problem and solution. Looking at the different impacts from different stages of R&D process can help distribute responsibility. That is, how different parties (energy suppliers, hardware builders, data scientists) play unique roles in the stages of AI R&D. More specifically, we can examine the environmental impact of each player within AI R&D. So rather than just computational efficiency in terms of outputs, we can consider the range of influence on carbon footprint such as electricity supply,, hardware employed, and even time of day for training, so that each player has their own recognized role and responsibility to find a more environmentally considerate process. This, Tamburrini arges, should distribute responsibility fairly.
His proposed means to encourage such responsibility is AI research competitions, which can focus on the carbon footprint of an AI system’s development as well as the accuracy of outputs. His solution seems feasible, as winning esteem and standing in the community will no doubt motivate a shift in priorities for AI scientists.
Between the lines
Tamburrini approaches the very real, yet poorly recognised, challenge of mitigating the environmental consequences of AI R&D. There is an opportunity to push this idea further. Tamburrini rightly acknowledges that GHG from AI is a global issue, but does not consider the unequal distribution of climate change affects across the globe. GHG emissions impact communities and geographical locations differently. Some countries are more negatively affected than others, already experiencing drought, flooding, and melting glaciers, and marginalized or disadvantaged groups face a changing climate more intensely than those who are privileged or living in parts of the world less drastically affected. Tamburrini should examine the object of our responsibility in more detail; who or what do we owe responsibility to?Â