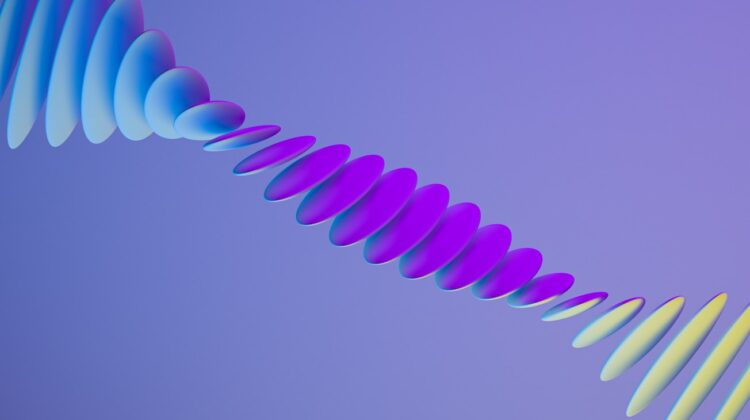
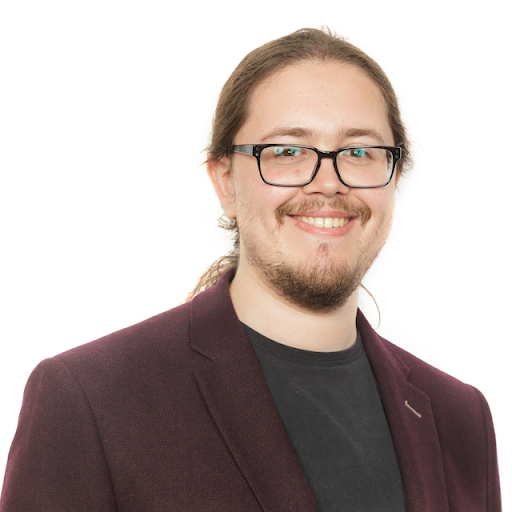
🔬 Research Summary by Ismael Kherroubi Garcia. Ismael is trained in business management and philosophy of the social sciences. He is the founder and CEO of Kairoi, the AI Ethics and Research Governance Consultancy; founder and co-lead of the Responsible AI Network (RAIN) at the RSA; and co-chair of the Certification Working Group at the Open Modeling Foundation (OMF).
[Original paper by Ismael Kherroubi Garcia (Kairoi), Christopher Erdmann (SciLifeLab), Sandra Gesing (US-RSE), Michael Barton (Arizona State University), Lauren Cadwallader (PLOS), Geerten Hengeveld (Netherlands Institute of Ecology), Christine R. Kirkpatrick (San Diego Supercomputer Centre), Kathryn Knight (Oak Ridge National Laboratory), Carsten Lemmen (Helmholtz-Zentrum Hereon), Rebecca Ringuette (Heliophysics Digital Resource Library), Qing Zhan (Royal Netherlands Institute for Sea Research), Melissa Harrison (EMBL-EBI), Feilim Mac Gabhann (Johns Hopkins University), Natalie Meyers (University of Notre Dame), Cailean Osborne (University of Oxford), Charlotte Till (Arizona State University), Paul Brenner (University of Notre Dame), Matt Buys (Datacite), Min Chen (Nanjing Normal University), Allen Lee (Arizona State University), Jason Papin (University of Virginia) and Yuhan Rao (North Carolina State University)]
Overview: Computational models are complex scientific constructs that have become essential for us to better understand the world. Many models are valuable for peers within and beyond disciplinary boundaries. This paper suggests 10 simple rules for you to both (1) ensure you share models in a way that is at least “good enough,” and (2) enable others to lead the change towards better model-sharing practices.
Introduction
The open science movement advocates for greater transparency across scientific domains for the purpose of more robust scientific work. Computational modeling, in turn, refers to a range of critical methods for studying diverse social and natural phenomena. At the intersection of open science and modeling, the Open Modeling Foundation (OMF) has a vision for “a common suite of ethics, standards, protocols, and best practices that enable modeling scientists to share knowledge and build on one another’s research.”
To this end, the OMF’s certification working group —co-chaired by Christopher Erdmann, Sandra Gesing and Ismael Kherroubi Garcia— set out to explore how open science practices can translate into computational modeling. Through a series of online workshops conducted in early 2024, the certification working group convened 14 experts and built a community of over 80 individuals to collate insights for good model-sharing practices.Â
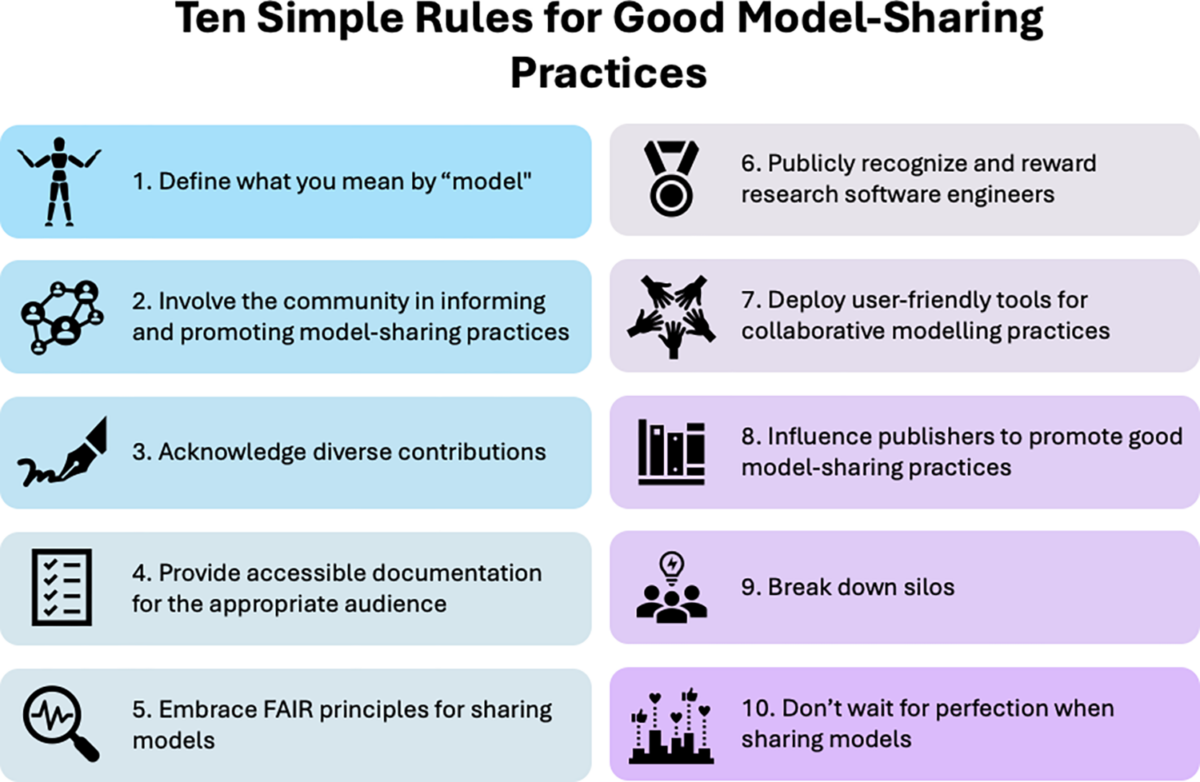
Key Insights
The following 10 simple rules are designed to enable and promote good model-sharing practices that are tenable and flexible —this is why they are “good” and not “best” practices. We also note that we do not use “good” in its moral sense and that ethical considerations involved when sharing models and their diverse elements are beyond this paper’s scope. Incorporating some or all of the below practices into your model-sharing can significantly increase your work’s impact on the community, often resulting in increased citations, collaborations, opportunities, and funding.
1. Define what you mean by “model”
Scientists and organizations can only understand one another and collaborate effectively when they use terms in similar ways. A collision of terminology is highly likely since models can be very diverse in nature. Therefore, when sharing your models—or even just speaking about models—clearly articulate what “model” means to yourself, your team, and your community.
2. Involve the community in informing and promoting model-sharing practices
Community building is a key element in promoting good model-sharing practices. Individual model developers are embedded in larger communities, and their behaviors are guided by community norms. You may shape those norms by involving your communities when sharing models.
3. Acknowledge diverse contributions
Computational modeling is usually a multidisciplinary endeavor. Contributions may be of very different types, involving model developers, domain experts, archivists, and policy-makers. With this, you must be ready to acknowledge such a great variety of contributors, and the Contributor Roles Taxonomy (CRediT) is one approach toward this goal.
4. Provide accessible documentation for the appropriate audience
Make your model reusable and more impactful by providing documentation alongside it. By “documentation,” we mean a collection of documents and additional written material that describe a computational model across its entire life cycle and along with its underlying assumptions and scientific bases. This helps communicate to diverse stakeholders why a model is worth using.
5. Embrace FAIR principles for sharing models
The FAIR principles—findability, accessibility, interoperability and reusability— have gained significant traction throughout open science initiatives. It is important that you don’t try to reinvent the wheel when it comes to good model-sharing practices. With this, you can draw on the FAIR principles when sharing information about your model in its metadata.
6. Publicly recognise and reward research software engineers
Research software engineers (RSEs) can play important roles in modeling projects but have only garnered attention in recent years. With RSEs’ varied contributions to modeling, it is important to specifically recognize and reward their work.
7. Deploy user-friendly tools for collaborative modeling practices
User-friendly tools—such as interfaces where users can request a model execution—are essential for models to be more seamlessly adopted by domain experts, including those who may lack specialized computer science training. Models often rely on complex cyberinfrastructure, and science gateways offer a solution for making modeling more accessible and straightforward.
8. Influence publishers to promote good model-sharing practices
As part of the research community, you have the power to influence what model-sharing practices are valued and adopted. Whichever your role in modeling, you should guide your peers and future scholars towards good model-sharing practices, and seek opportunities to inform the policies that publishers implement.
9. Break down silos
Working within silos is detrimental to scientific progress. With modeling being inherently multidisciplinary, you should value the role of collaborating across disciplines and organizations as central to all good model-sharing practices. After all, computational models are shared by people for scientific and policy advancement via platforms.
10. Don’t wait for perfection when sharing models
As the adage goes, perfection is the enemy of progress. We cannot be paralyzed by the desire to enact every good model-sharing practice each and every time. Rather, we must do the best we can, given the resources we have access to and the policies our institutions implement.
Between the lines
Ten Simple Rules for Good Model-sharing Practices is the result of a huge, multidisciplinary, international collaboration. The paper advocates for just that in the context of computational modeling. To make the most of models and the efforts and resources that go into them, we must seek collaboration with others, promote good practices, and enable their reuse.
In a world where modeling has proven to be such a valuable method for scientific enquiry and discovery—in biology, sociology, philosophy, astrophysics and so on—it is crucial that we are transparent about the practices that work, making it easier for others to also follow good model-sharing practices.