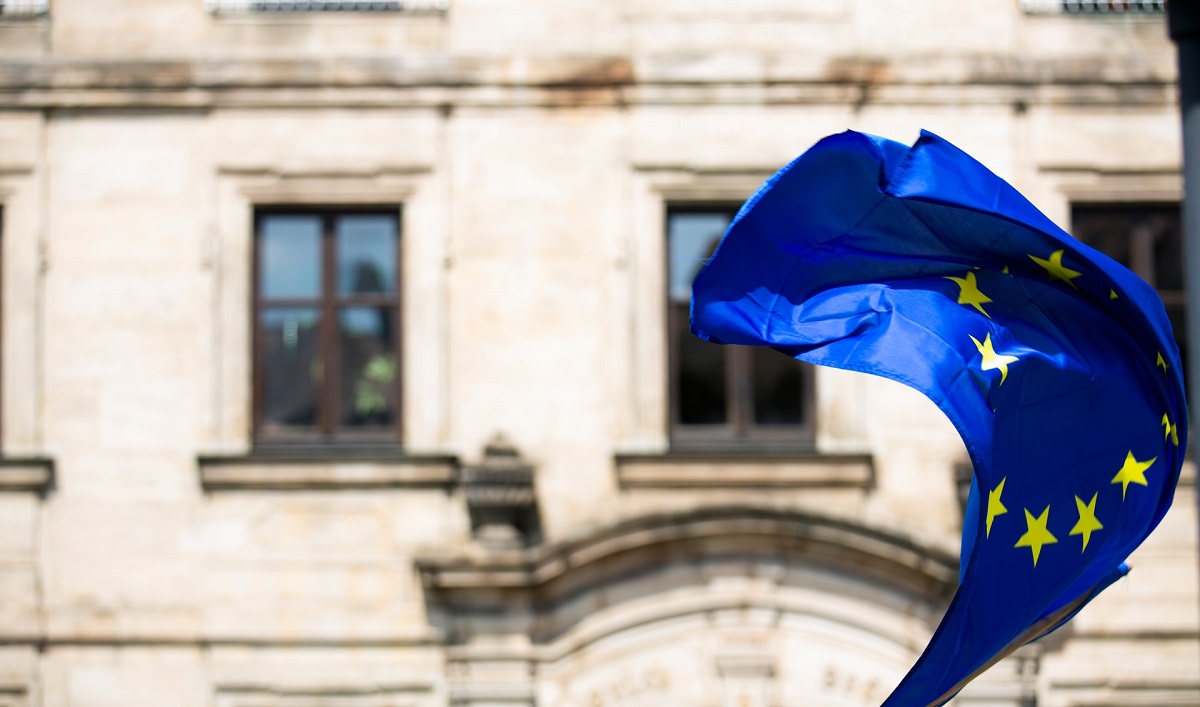
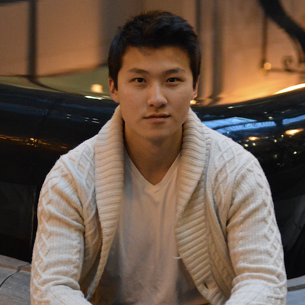
🔬 Research summary by Jimmy Huang, Financial Technology Researcher.
[Original paper by Jimmy Huang, Abhishek Gupta, Monica Youn]
Overview: This paper evaluates the efficacy of current EU ethical guidelines for commercial AI—specifically the “European framework on ethical aspects of artificial intelligence, robotics and related technologies”, and provides a regulatory recommendation where there are gaps. We delve into 3 use-cases in the financial services space to highlight the spectrum of ethical risks that arise from each implementation.
Introduction
AI ethics and data privacy issues have been increasingly occupying public consciousness as hot button topics. By evaluating the effectiveness of EU standards against three varied, real-world cases in the financial sector, we are able to pinpoint practical pitfalls in the current framework for future improvement. The three cases are taken from the financial sector as firms within this industry, on average, spend the most in percentage of total revenue on IT projects and are generally opaque in the sense that the goal of these private IT projects are not usually visible or known to the public.
Each practical application and business use-case employing AI to automate tasks come with different levels of ethical risks. The issue of subjectivity in the initial ethical risk assessment further complicates matters especially when it is an AI-enabled system with far-reaching effects that should be evaluated. The paper serves to highlight both the range of ethical risks as well as to provide a novel perspective on what may be improved in future standards.
Discrimination in Mortgage Applications
AI-enabled systems seeking to automate the mortgage application process face the risk of using inappropriate metadata from training sets such as race or gender features in filtering out or determining the creditworthiness of applications.
Data that may be used in a discriminatory manner should be properly controlled upstream at the data aggregation-level. The paper references previous research from Fuster et al. who have used historical U.S. administrative mortgage data as a training set in predicting creditworthiness and found that “…minority groups appear to lose, in terms of the distribution of predicted default propensities, and in our counterfactual evaluation, in terms of equilibrium rates…” (source). That being said, EU standards have been able to effectively tackle most data privacy issues and seek to identify discriminatory behaviour in data processing with rules such as the GDPR set of rules.
Unexplained Amounts in Trade Reconciliations
AI-enabled systems that automate the matching of different datasets for a trade reconciliation and reporting use-case face little to no ethical risk if implemented incorrectly. This is because any unexplained amounts will be caught in peer-auditing tasks and the harm caused from any faulty reconciliations would be limited to affecting the profits of the firm that produced the mistake in question.
Automated Trading Algorithms Causing Market Shocks
Out of the three use-cases, the most opaque AI implementations are the ones employed by sophisticated investment firms to automate order entries and trades. As these AI-enabled systems trade among each other and add complexity to a highly interlinked system, unforeseen market shocks could arise due to the unpredictable nature of their interactions, especially with research from Kirilenko et al. corroborating that “High Frequency Traders aggressively trade in the direction of price changes” (source).
Additionally, if market consequences do occur, it is extraordinarily difficult to determine the degree of harm produced by the systems and to track the systems accountable. It is evident that the current EU framework lacks a comprehensive mechanism to hold systems or agents accountable in use-cases such as this where harm arises from many sophisticated AI-enabled systems interacting with each other.
Between the lines
The current EU framework handles the mortgage application and trade reconciliation use-cases effectively with provisions that include comprehensive human-in-the-loop (HITL), risk assessment, data privacy, and ex-ante procedures. However, for the trading algorithm use-case, the paper includes an example novel solution to hold applications accountable such as with system registration and additional system tracking as an amendment to current trade reporting rules (such as with the MiFID II / MiFIR post-trade reporting requirements). This is not meant to be prescriptive, rather, it is meant to encourage and illustrate how regional regulatory bodies within the relevant industry can add additional rules covering specific business applications on top of existing federal or EU-wide rules. With knowledge of the industry, these bodies are well suited to understand the nuances of each business use-case, its implications on society, and how to effectively enforce controls.