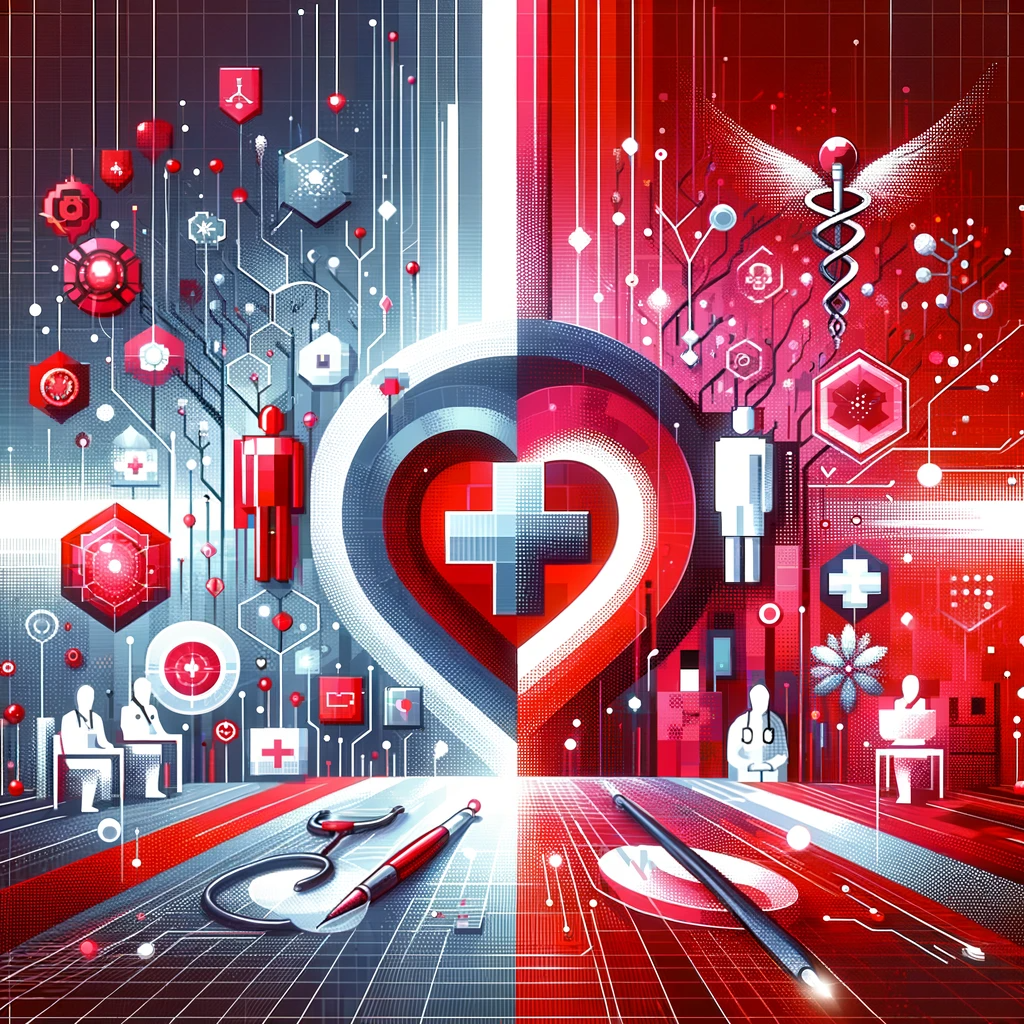
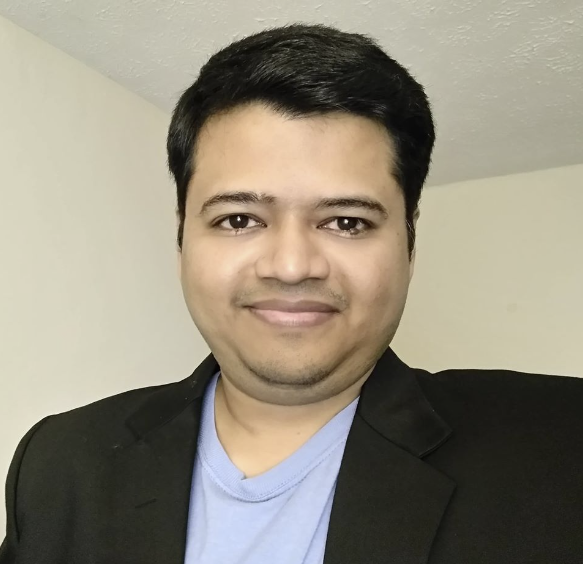
🔬 Research Summary by Prathamesh Muzumdar, Director of IT services and cloud computing with research focused on Ethical and Fair AI
[Original paper by Dr. Apoorva Muley, Prathamesh Muzumdar, George Kurian, and Ganga Prasad Basyal]
Overview:
AI and its applications have found their way into many industrial and day-to-day activities through advanced devices and consumer’s reliance on the technology. One such domain is the multibillion-dollar healthcare industry, which relies heavily on accurate diagnosis and precision-based treatment, ensuring that the patient is relieved from his illness in an efficient and timely manner. This paper explores the risks of AI in healthcare by meticulously exploring the current literature and developing a concise study framework to help industrial and academic researchers better understand the other side of AI.
Introduction
Some argue that the potential of AI in medicine has been overestimated, citing a lack of concrete data showing significant patient outcome improvements. This skepticism questions the widespread adoption and transformative impact of medical AI. Experts also express concerns about the potential negative consequences of medical AI, spanning clinical, technical, and socio-ethical risks. These issues emphasize the importance of carefully evaluating and regulating AI in healthcare to ensure patient safety and address unintended adverse effects.
While AI in healthcare shows promise, assessing its performance, benefits, and risks for informed integration into medical practice is crucial. Like any emerging technology, cautious and responsible implementation is vital to unlock its true potential while minimizing downsides. The literature identifies seven main risk categories for introducing AI into future healthcare: patient harm from AI errors, misuse of AI tools, bias and inequities, lack of transparency, privacy and security concerns, accountability gaps, and implementation challenges.
Addressing these risks necessitates collaboration among stakeholders such as healthcare professionals, AI developers, policymakers, and ethicists. Robust evaluation, regulation, and continuous monitoring of AI systems are essential to maximize benefits while minimizing potential negative impacts on patient care and healthcare delivery. A recent study reviewed 39 articles from 2018 to 2023, creating a comprehensive framework for understanding AI risks in healthcare. This framework aims to provide a clear guide for mitigating these risks.
Key Insights
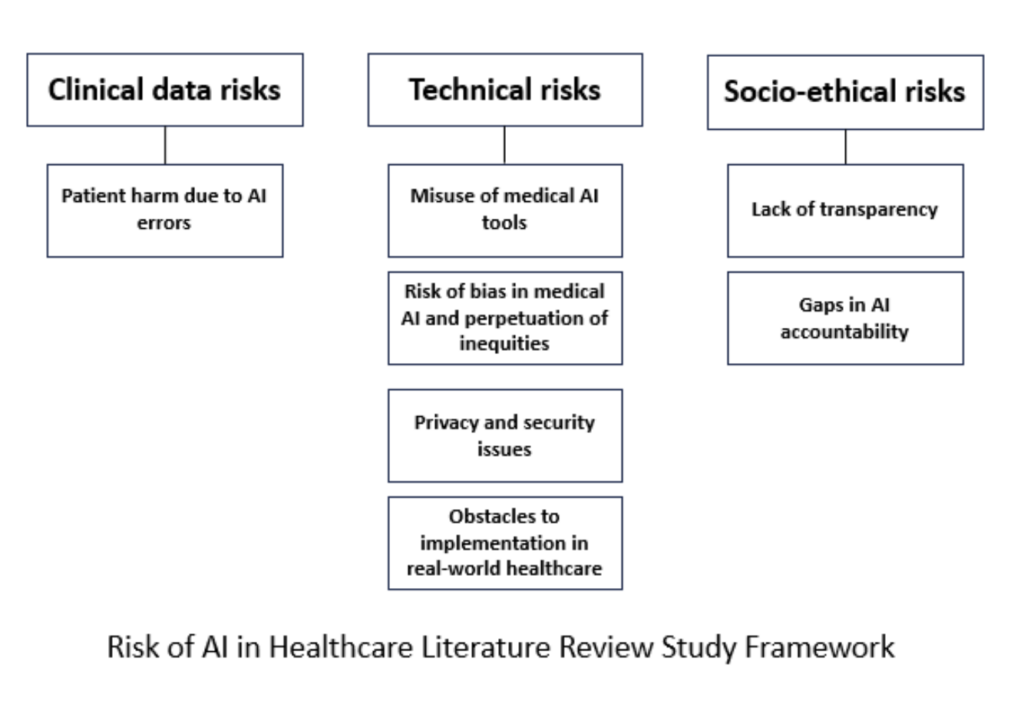
- Clinical Data Risks
- Clinical data risks encompass the potential for unauthorized access, disclosure, or manipulation of sensitive patient information, which could compromise privacy and confidentiality. Additionally, inaccuracies or biases within clinical data sets may lead to erroneous diagnoses or treatment recommendations, posing significant threats to patient safety and quality of care.
- Technical Risks
- Misuse of medical AI tools can occur when healthcare professionals rely too much on automated suggestions without critical evaluation, potentially leading to incorrect diagnoses or treatment decisions. Additionally, inappropriate deployment or customization of AI algorithms without proper training and understanding of their limitations can contribute to patient safety concerns and suboptimal healthcare outcomes.
- The risk of bias in medical AI arises from the potential for algorithms to be trained on historically biased data, leading to disparities in diagnosis and treatment recommendations across different demographic groups. If not carefully addressed, this bias can perpetuate existing inequities in healthcare, potentially resulting in unequal access to accurate diagnoses and appropriate care for marginalized communities.
- Privacy and security issues in healthcare AI revolve around safeguarding sensitive patient information from unauthorized access, breaches, or cyberattacks. Failing to protect this data adequately can lead to serious consequences, including identity theft, compromised medical records, and erosion of patient trust in healthcare systems.
- Implementing medical AI in real-world healthcare faces challenges such as regulatory hurdles and the need for standardized evaluation criteria. Additionally, ensuring seamless integration with existing healthcare workflows and systems and addressing concerns about liability and accountability present significant obstacles to widespread adoption.
- Socio-Ethical Risks
- The lack of transparency in AI applications within healthcare raises socio-ethical concerns, as it may hinder patients’ understanding of how decisions are made about their health. Without clear explanations of AI-driven recommendations, trust in the healthcare system and the medical profession could erode, potentially reducing patient compliance and engagement.
- Gaps in AI accountability in healthcare stem from challenges in attributing responsibility for decisions made by autonomous systems. Determining who is ultimately responsible in cases of errors or adverse outcomes involving AI-driven interventions remains a complex and evolving ethical and legal issue, potentially hindering the establishment of clear lines of accountability.
Between the Lines
This extensive literature review has carefully analyzed 39 articles that center on the risks associated with AI in healthcare. By thoroughly examining the literature, a strong framework has been established, illuminating three main categories of AI risks: clinical data risks, technical risks, and socio-ethical risks. The study goes deeper into each category, exploring various sub-categories and providing a nuanced grasp of the complex challenges surrounding the integration of AI in healthcare. By offering this detailed reference, the article equips researchers, policymakers, and healthcare professionals with valuable insights to support qualitative and quantitative research on AI risks in healthcare. This framework improves our understanding of the potential drawbacks linked with AI adoption and is a vital guide in formulating effective risk mitigation strategies.