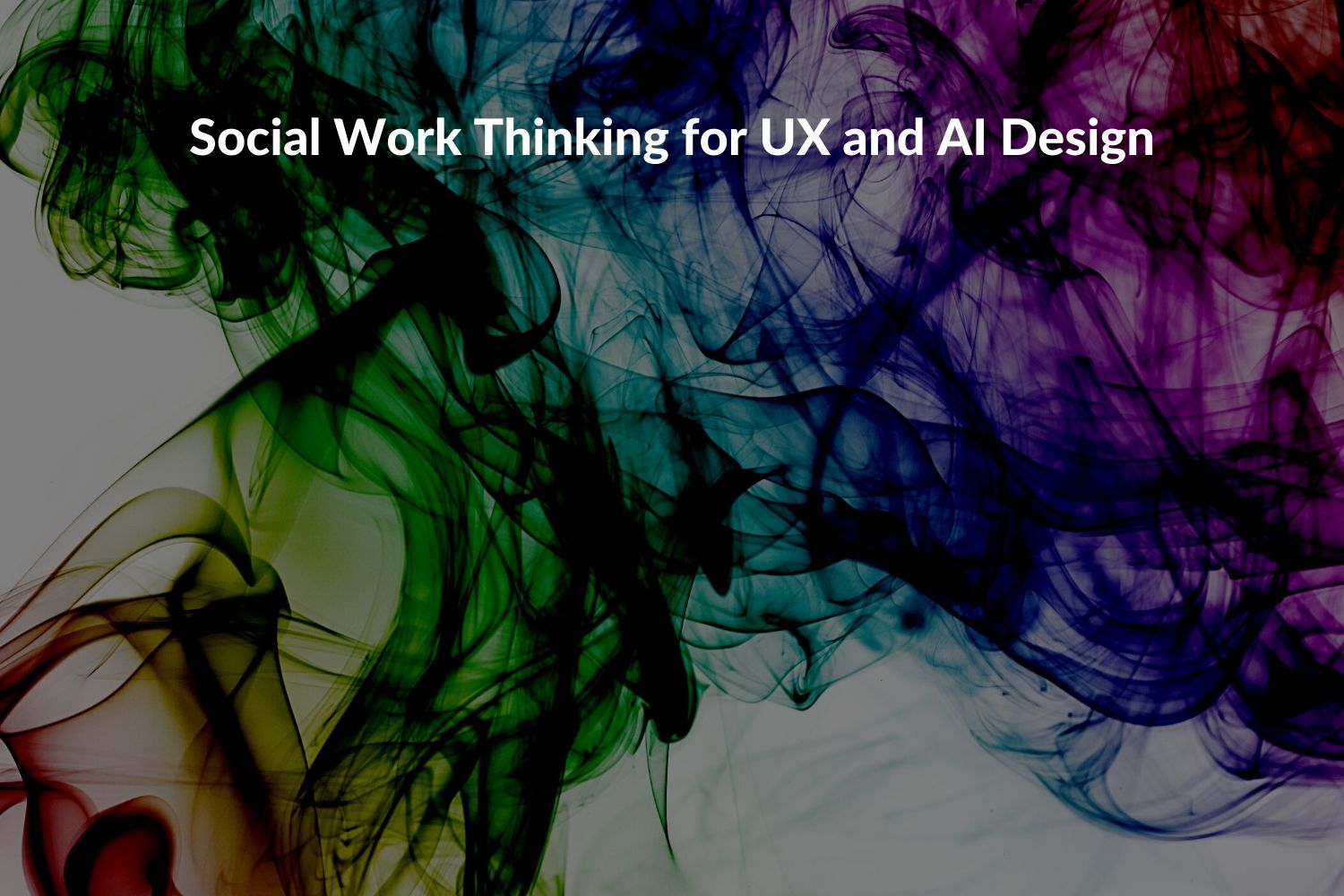
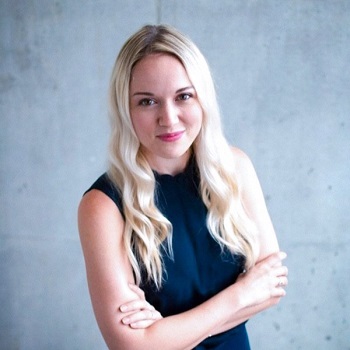
Summary contributed by Victoria Heath (@victoria_heath7), Communications Manager at Creative Commons
*Authors of full paper & link at the bottom
Mini-summary: What if tech companies dedicated as much energy and resources to hiring a Chief Social Work Officer as they did technical AI talent? If that was the case, argues Desmond Upton Patton (associate professor of social work, sociology, and data science at Columbia University, and director of SAFElab), they would more often ask: Who should be in the room when considering “why or if AI should be created or integrated into society?” By integrating “social work thinking” into the process of developing AI systems, these companies would be better equipped to anticipate how technological solutions would impact various communities.
The code of ethics that guides social workers, argues Patton, should be used to guide the development of AI systems—leading companies to create systems that actually help people in need, address social problems, and are informed by conversations with the communities most impacted by the system. In particular, before looking for a technical solution to a problem, the problem must be fully understood first, especially as it’s “defined by the community.” These communities should be given the power to influence, change, or veto a solution. To integrate this social work thinking into UX and AI design, we must value individuals “beyond academic and domain experts.” Essentially, we must center humanity and acknowledge that in the process of doing so, we may end up devaluing the power and role of the technology itself.
Full summary:
What if tech companies dedicated as much energy and resources to hiring a Chief Social Work Officer as they did technical AI talent (e.g. engineers, computer scientists, etc.)? If that was the case, argues Desmond Upton Patton (associate professor of social work, sociology, and data science at Columbia University, and director of SAFElab), they would more often ask: Who should be in the room when considering “why or if AI should be created or integrated into society?”Â
By integrating “social work thinking” into their process of developing AI systems and ethos, these companies would be better equipped to anticipate how technological solutions would impact various communities. To genuinely and effectively pursue “AI for good,” there are significant questions that need to be asked and contradictions that need to be examined, which social workers are generally trained to do. For example, Google recently hired individuals experiencing homelessness on a temporary basis to help collect facial scans to diversity Google’s dataset for developing facial recognition systems. Although on the surface this was touted as an act of “AI for good,” the company didn’t leverage their AI systems to actually help end homelessness. Instead, these efforts were for the sole purpose of creating AI systems for “capitalist gain.” It’s likely this contradiction would have been noticed and addressed if social work thinking was integrated from the very beginning.
It’s especially difficult to effectively pursue “AI for good” when the field itself (and tech more broadly) remains largely racially homogenous, male, and socioeconomically privileged; as well as restricted to those with “technical” expertise while other expertise is largely devalued. Patton asks, “How might AI impact society in more positive ways if these communities [e.g., social workers, counselors, nurses, outreach workers, etc.] were consulted often, paid, and recognized as integral to the development and integration of these technologies…?”
Patton argues that systems and tools can be used to both help a community, and hurt it. “I haven’t identified an ethical AI framework,” he wrote, “that wrestles with the complexities and realities of safety and security within an inherently unequal society.” Thus, an AI technology shouldn’t be deployed in a community unless a “more reflective framework” can be created that “privileges community input.” When developing these systems, it’s important to admit, as Patton does, that the technical solution may not be what’s needed to solve the problem.
Through his work at SAFElab, Patton has nurtured collaboration between natural language processing (NLP) and social work researchers to “study the role of social media in gun violence,” and create an AI system that predicts aggression and loss. Their approach was to first collect qualitative data by social workers trained in annotation who provided an analysis that then influenced the development of the “computational approach for analyzing social media content and automatically identifying relevant posts.” By working closely together, the social workers and the computer scientists were able to develop a more contextualized technical solution to a problem that was cognizant of the “real-world consequences of AI.”
In order to effectively ask the right questions and deal with the inherent complexities, problems, and contradictions with developing “AI for good,” we need to change who we view as “domain experts.” For the project at SAFElab, for example, they developed an “ethical annotation process” and hired youth from the communities they were researching in order to center “context and community voices in the preprocessing of training data.” They called this approach Contextual Analysis of Social Media (CASM). This approach includes collecting a baseline interpretation of a social media post from an annotator who provides a contextualized assessment; and then debriefing, evaluating, and reconciling disagreements on the labeled post with the community expert and the social work researcher. Once done, the labeled dataset is then given to the data science team to use in training the system. This approach eliminates the “cultural vacuum” that can exist in training datasets from the beginning and throughout the entire development process.
The code of ethics that guides social workers, argues Patton, should be used to guide the development of AI systems—leading companies to create systems that actually help people in need, address social problems, and are informed by conversations with the communities most impacted by the system. In particular, before looking for a technical solution to a problem, the problem must be fully understood first, especially as it’s “defined by the community.” These communities should be given the power to influence, change, or veto a solution. To integrate this social work thinking into UX and AI design, we must value individuals “beyond academic and domain experts.” Essentially, we must center humanity and acknowledge that in the process of doing so, we may end up devaluing the power and role of the technology itself.
Original paper by Desmond Upton Patton: https://dl.acm.org/doi/10.1145/3380535