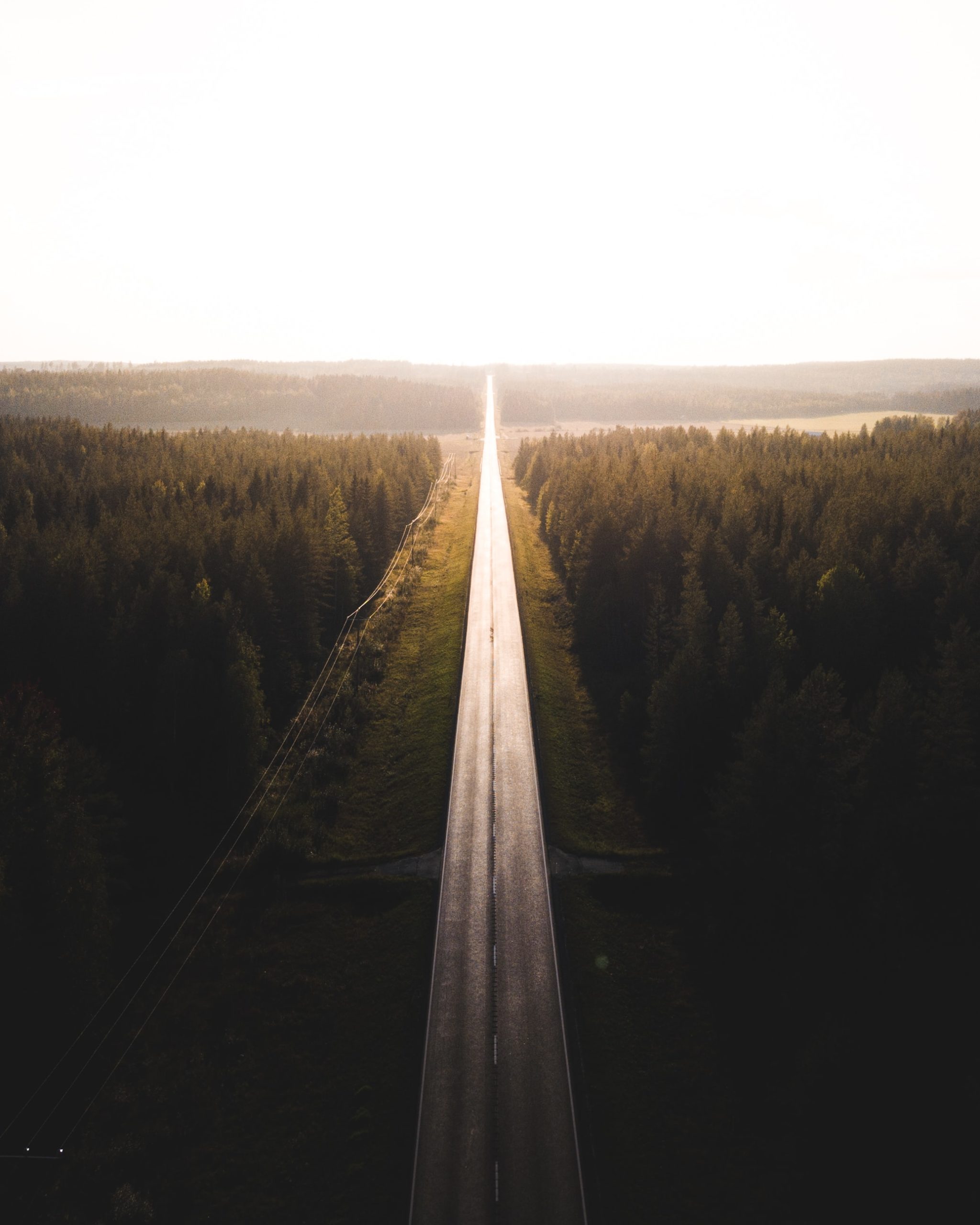
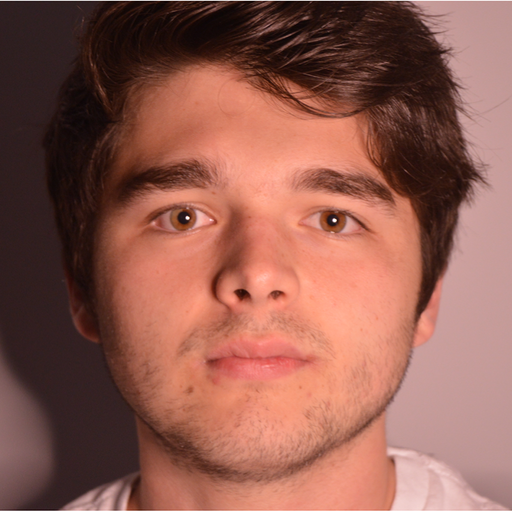
š¬ Research summary by Connor Wright, our Partnerships Manager.
[A podcast from the World Economic Forum: Radio Davos]
Overview: Will the rise of the machines solve our problems or prove detrimental to our existence? A robot uprising is not really on the cards, but there are equally scary prospects taking place today.
Introduction
The rise of artificial general intelligence (AGI) against humans has been a hot topic in the AI literature. Yet, in this podcast, Professor Russell says this is not on the cards. Instead, we have other issues to deal with first. To explain, Iāll go through a definition of AI before covering AI in three use cases: social media, facial recognition technology (FRT) and the economy. Iāll then observe how AGI has always been the goal of AI design. I then conclude how AI is not intrinsically good or evil, and weāre the ones whoāll decide which way it goes.
Key Insights
To begin with, AI is treated as a spectrum. Rather than advocating for an explanation along the lines of āan AI system is one which has X number of rulesā, it is set on a scale from extremely simple agents to extremely complex agents continuum. The highest complex agent is centred on a human.
Situated on the lower end are systems based in more rigid environments. Algorithms that turn on your house lights at 6 pm and turn them off at 11 pm are a good example. Yet, within the inbetween, we have an interesting plethora of algorithms, some of which are pivotal in influencing the social media landscape.
How AI is affecting social media
Algorithms want to maximise click-through. Initially, this goal was met by sending people the content they like. However, the way to fully encounter click-through is to supply content that molds the human into the ideal candidate, i.e. a person that spends time on the platform. Through these hundreds of little nudges a day, the algorithms begin to alter peopleās beliefs. Subsequently, those molded into ideal candidates start specialising in specific content streams, creating polarisation between those focussed on different subject areas.
As a result, Professor Russell calls for more visibility into how these algorithms work. He believes that this is a byproduct of algorithmic design, with researchers being given the benefit of the doubt about not intending to allow algorithms to have this effect. When an algorithm generates lots of money for the company, thereās added pressure not to change the algorithm. Hence, despite researchers being aware of this algorithmic effect, they may not be empowered to change it.
Hence, to attack this problem, we shouldnāt think about revenue. Rather, directing our energy towards what our users care about should be at the core of social media. This can be seen in the realm of FRT.
AI and FRT
Mistakes made once FRT is deployed are not so much because the data is āwrongā but more so attributable to a non-representative dataset. Given this reality, itās a hot topic whether there is a way to create a perfectly representative dataset. For example, whatās representative in Namibia will be different to Thailand. Hence, the question of how we deal with the dangers of this technology becomes socio-technical.
What matters is how we respond to the issues and how we adapt your system once it gets deployed. For example, it may become the case that people do not want to release their data to be included in the new FRT system. Consequently, they do not consent to the use of FRT and cannot enter the spaces in which itās established. There may even be scenarios where consent is not required, such as losing your job.
How AGI would affect the economy
The British economist John Maynard Keynes mentioned technological unemployment in his research, saying we would not need any workers, given the trajectory of technological innovation. What rings especially true is the impact that small nudges this innovation has on the economy. If we designed a robot to be able to pick up any object out of a bin, 3-4 million peopleās jobs would be put at risk. An automated taxi would be a quarter of the price of a regular taxi, affecting the job security of 25 million people.
Training everyone to be a data scientist or to have a job related to AI will not solve this problem and may not even be possible. Instead, asking questions about whether automation is necessary in the first place could be a promising initial step. Nevertheless, the march towards AGI goes on.
General-purpose AI has always been the goal
AGI systems are those which can carry out or learn any task that humans can do and do them better. The problem we find when creating these systems comes with how AI must know the full ins and outs of a task to carry out their work. For example, we canāt instruct an AI to solve climate change as we donāt know what this entirely involves. If we wanted an AI to solely construct electric vehicles, we donāt know what this future would look like.
Professor Russell notes how if we create systems where they are aware that we donāt know the entire outcome, they will ask permission. This gives us more control over their behaviour. Control comes through the machineās uncertainty over what the objective is.
The arrival date of AGI wonāt be overnight. While the end of the century could be promising to Professor Russell, there will be many other scenarios to follow up on first, especially regarding the economy.
Between the lines
Uncertainty is part of our world, which doesnāt suit AI very well. Hence, humans need to prioritise building adaptive and flexible systems to deal with the inevitable. It will be impossible to predict what scenarios could result from a system, especially if itās never been released before. While this is daunting, it can also be exciting. It means that AI is not intrinsically good or evil. Instead, itās up to us and, for good or worse, we are the ones making the decisions.