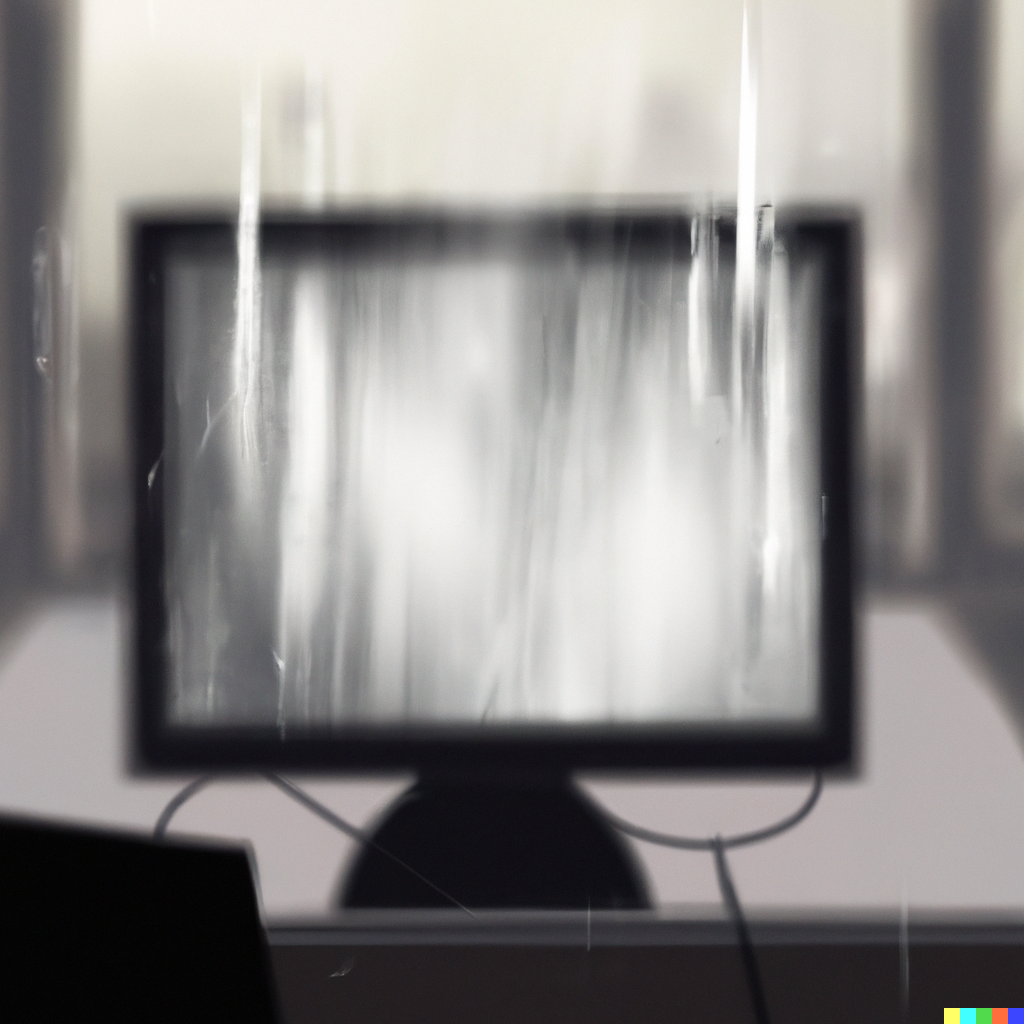
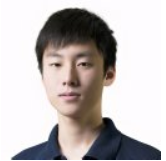
🔬 Research Summary by David Zhang, a PhD Candidate and Research Engineer at CSIRO’s Data61, and his research focuses on understanding the societal implications of AI technology.
[Original paper by Dawen Zhang, Pamela Finckenberg-Broman, Thong Hoang, Shidong Pan, Zhenchang Xing, Mark Staples, and Xiwei Xu]
Overview: The right to be forgotten (RTBF) is an integral aspect of the right to privacy and was established through a landmark case involving search engines. It is also a demonstration of the emergence of new rights as a result of technological advancements. This paper delves into the legal principles behind RTBF, highlighting that Large Language Models (LLMs), the emerging technology behind popular chatbots, are not exempt from this regulation, and in reality, their practical adherence to the law is highly challenging. The paper identified multiple issues of LLMs related to RTBF and offered potential directions for addressing the challenges.
Introduction
In March 2023, Italy temporarily banned ChatGPT, citing privacy concerns. OpenAI, the company behind this chatbot, also faces a class action in California against its use of data, which may violate internet users’ privacy. The widespread comparison between ChatGPT and search engines prompted us to delve into its adherence to the right to be forgotten – a right, as an aspect of the right to privacy, established along with the emergence of search engines.
Through the investigation, we identified the issues of LLMs in relation to the right to be forgotten (RTBF), including training data memorization and hallucination. We further summarized the societal and technological similarities and dissimilarities between search engines and LLMs, leading to the greater complexity of RTBF compliance in LLMs. RTBF may apply in two parts of data in LLMs, i.e., chat history and in-model data, and the mechanism of LLMs makes the relevant personal information significantly difficult to access, delete, or rectify. The problem of hallucination may even extend this difficulty further.
We compiled a set of potential solutions to these issues, encompassing machine unlearning, model editing, and prompting. We also provide our insights from the legal perspective, including the issues related to the definition of undue delay, the trade-offs between human rights and technological advancements, and the interpretation of legitimate interests outlined in GDPR.
Key Insights
Though having several similarities, LLMs are different from search engines. When understanding the Right to be Forgotten (RTBF), established through a case involving search engines, we cannot only look at the legal text itself but dive into the legal principles behind it. We picked out three points from the very first case:
Privacy: The ruling cites Articles 7 and 8 of the Charter of Fundamental Rights of the European Union, declaring that the processing of personal data should respect the privacy of data subjects. Interestingly, the ruling explicitly mentioned that the data subject’s personal information would not be ubiquitously available and interconnected without the existence of the internet and, specifically, search engines in modern society. This clearly suggested how RTBF emerged due to technological advancements interfering with the rights of people. The LLMs, seen as a disruptive technology by many, play a similar role subject to relevant regulations.
Legitimate interests: The ruling found that the operators of search engines are the controllers of their data, as their processing of data has different legitimate interests and consequences from the original publishers of information.
Balancing of interests: The ruling acknowledges that the processing of personal information is necessary if the processing is for legitimate interests but that such interests can be overridden by the interests of the data subject’s fundamental rights and freedoms. This also suggests that, even though LLM-powered applications are labeled as “research preview” or “experiment” to put themselves within the definition of legitimate interests, these legitimate use of data may still be overridden by the data subject’s right to privacy.
LLMs vs. search engines
RTBF in search engines has very mature technical solutions, while in comparison, LLMs face significant challenges, which are the result of the unique characteristics of LLMs. We summarized the similarities and dissimilarities between LLMs and search engines.
Similarities
Organizing internet data. LLMs and search engines have sourced data from the internet. Specifically, LLMs are deep neural networks trained on crawled data also scraped from web pages, with the data embedded into these LLMs as weights, while search engines use crawlers to scrape web pages and index this data.
Used to access information. Users often employ LLMs and search engines to access information. While LLMs are trained on a vast amount of online information and generate responses based on their internal representations, search engines are used to search through online information. This usage has led to a debate about whether LLMs can replace search engines.
Intertwined with each other. LLMs have been embedded into search engines, e.g., Microsoft’s Bing, while search engines are also now embedded into LLMs, e.g., Google’s Bard.
Dissimilarities
Predicting words vs. indexing information. LLMs are trained to predict the next word in the text, and the relationship between words does not necessarily reflect the actual information and reality. On the other hand, search engines are created to collect, index, and rank relevant web pages based on user queries.
Conversational chatbots vs. search box. LLMs aim to assist users by employing the interfaces of conversational chatbots, in which users refine inputs about their problems in the form of multi-round conversations with LLMs. In contrast, search engines provide services through a user interface with a search box that receives users’ queries and outputs a list of relevant web pages.
Challenges of Applying RTBF on LLMs
With regard to the format of data, the right to be forgotten (RTBF) can be applied to two types of data: the user chat history and the in-model data, which can be further categorized into memorized data and hallucinated information.
User chat history. As mentioned previously, these LLMs are built into chatbots that interact with users in an anthropomorphic and conversational manner, which could retrieve more personal information from users.
In-model data. The memorized data is learned from training data, which can be removed from methods such as re-training; however, the hallucination is hard to eliminate, but the removal or rectification is codified in law, i.e., right to erasure and right to rectification. Moreover, these in-model data, both memorized and hallucinated, are hard to access due to the mechanism of LLMs, making it difficult to practice the right of access.
Potential technical solutions
Techniques such as machine unlearning are solutions designed for dealing with RTBF, while other methods, though they may not have a focus on RTBF, have the potential to provide solutions for RTBF. We categorized the solutions into two types. One type is fixing the original model, including Exact Machine Unlearning and Approximate Machine Unlearning. The other type is band-aid approaches, which include Model Editing and Prompting. These methods are still far from mature enough to serve as real-world AI systems solutions and require further research.
Legal perspectives
Legal issues also require further discussion, including the definition of “undue delay” and the interpretation of “legitimate interests” for data usage. It is imperative to acknowledge that as new technology becomes more aggressive and data-hungry, the balancing of power and the trade-offs between human rights and technological advancements need careful consideration by all stakeholders involved in legal matters to ensure the responsible and ethical use of technology while safeguarding individual rights in modern society.
Between the lines
Google recently proposed the Machine Unlearning Challenge at NeurIPS. One aim of Machine Unlearning methods is to address the RTBF. Machine unlearning methods have been there for several years but have not been put into industry practice, which reflects the immaturity of this stream of methods, while also showing that the researchers lack understanding of the actual complexity of the problem.
In terms of the issue related to RTBF, we believe there are gaps between the following:
- Law vs. the understanding and awareness of AI practitioners
- Law vs. technical reality and potential technical solutions
Through this paper, we want to fill these gaps and provide a comprehensive view of the complexity of the problem.