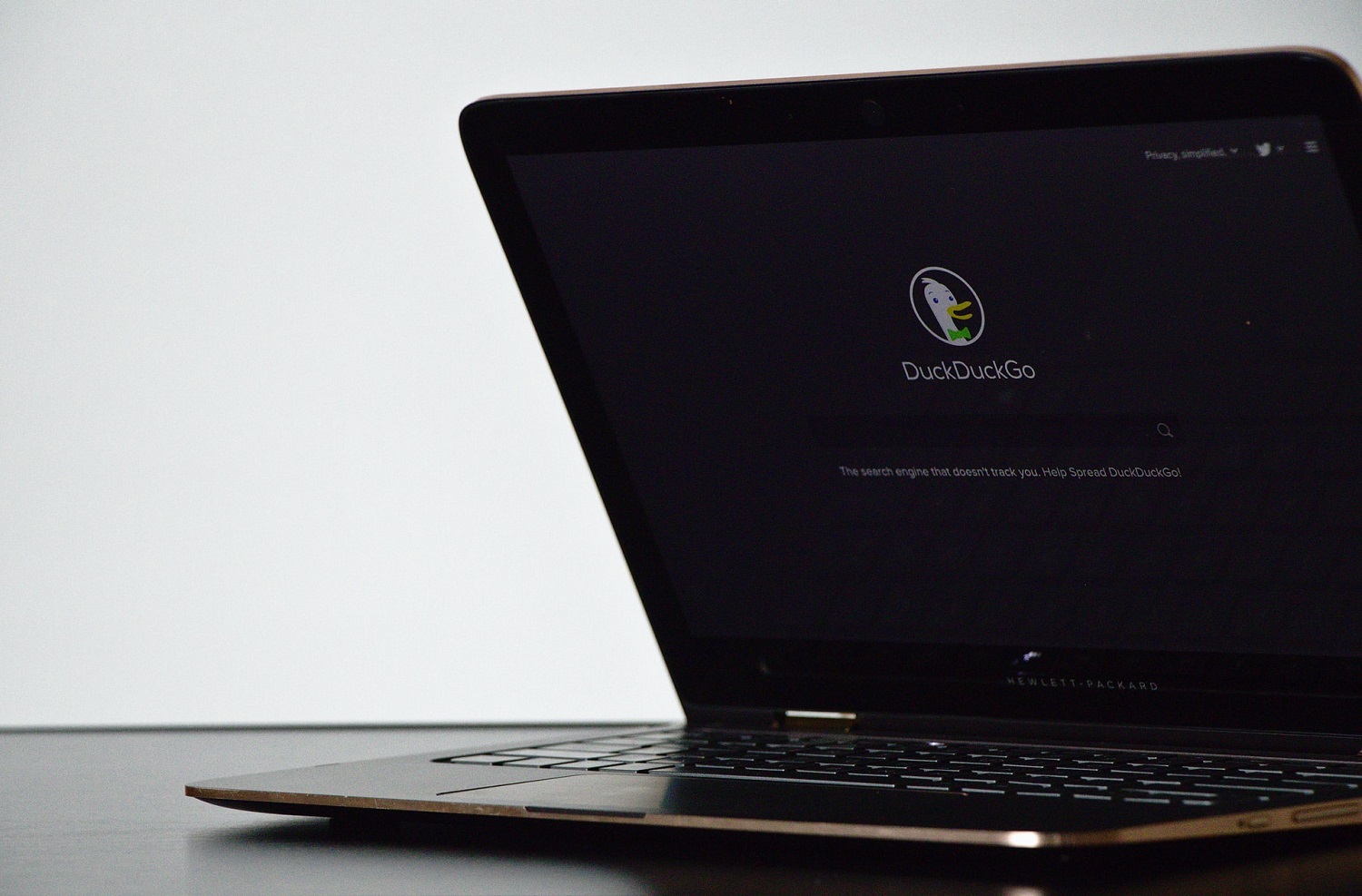
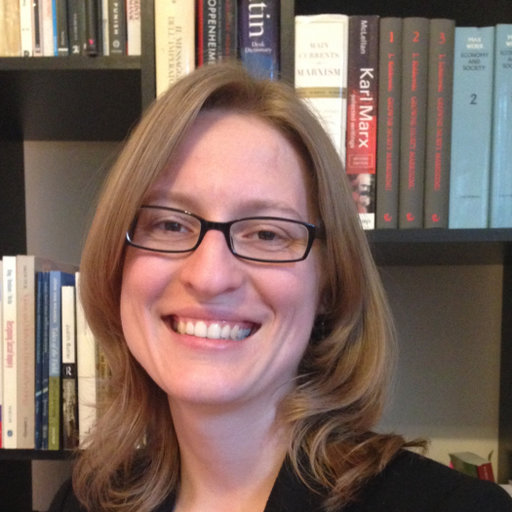
🔬 Research summary contributed by Dr. Iga Kozlowska (@kozlowska_iga), a sociologist working on Microsoft’s Ethics & Society team where she’s tasked with guiding responsible AI innovation.
✍️ This is part 3 of the ongoing Sociology of AI Ethics series; read previous entries here.
[Link to original paper + authors at the bottom]
Overview: Through ethnographic research, Ziewitz examines the “ethical work” of search engine optimization (SEO) consultants in the UK. Search engine operators, like Google, have guidelines on good and bad optimization techniques to dissuade users from “gaming the system” to keep their platform fair and profitable. Ziewitz concludes that when dealing with algorithmic systems that score and rank, users often find themselves in sites of moral ambiguity, navigating in the grey space between “good” and “bad” behavior. Ziewitz argues that designers, engineers, and policymakers would do well to move away from the simplistic idea of gaming the system, which assumes good and bad users, and focus instead on the ethical work that AI systems require of their users as an “integral feature” of interacting with AI-powered evaluative tools.Â
Remember JC Penny? In 2011, they were shamed in the pages of The New York Times for “gaming” Google’s search engine algorithm to boost their website’s ranking. They did this by having a bunch of irrelevant pages link to theirs so that the algorithm would read that as an indicator of the page’s relevancy and bump it up in the “organic” search results, right before the holiday shopping season. When Google found out, they “punished” JC Penny’s “bad” behavior by substantially lowering their ranking thereby reducing traffic to their website.
Ziewitz uses this case study to interrogate what we mean when we accuse algorithmic technology users of “gaming the system.” As machine learning models that rank, score, classify, and predict proliferate across AI applications in fields ranging from healthcare and journalism to credit scores and criminal justice, there is widespread concern around how to design and govern AI systems to prevent abuse and manipulation. Maintaining the integrity of AI systems is of paramount importance to most stakeholders, but it is a hard nut to crack.
From “Gaming the System” to “Ethical Work”
As with anything in the social world, what is “ethical” behavior is hard to pin down. People often straddle the line between good and bad behavior, constantly negotiating what is acceptable and what isn’t in any given situation. It may seem like we live in a world with hard and fast rules like “lying is bad” and “speaking the truth is good.” Instead, when we take a closer look, we see that we operate in a morally ambiguous world where “white lies” or coloring or downplaying the truth to protect others’ feelings, for example, may be acceptable. Intentions, identities, and context matter. By remembering that AI systems are in fact sociotechnical systems, we can expect people to engage with AI systems just like they do with other people i.e. in complex ways and from within existing (though everchanging) cultural norms, simultaneously reproducing and resisting them.
Because people alter their behavior as they negotiate the cultural rules of interacting back and forth with algorithms, ranking and scoring algorithms don’t just measure “objective” reality. Through interaction with people and instructions, algorithms co-create reality. It is through that “ethical work,” as Ziewitz calls it, that we collectively produce more or less ethical outcomes.
What does it mean then to design and build “ethical AI”? It requires us to take into consideration the ethical work that will be done through the building, deployment, maintenance, and use of the AI system. Below are some questions that ML developer teams can explore to move away from the binary thinking associated with “gaming the system” to a more nuanced approach that tries to understand the ambiguities, uncertainties, and context dependencies of algorithm-human interaction.
Applying Ziewitz’s Ideas to Machine Learning Development
Extending Ziewitz’s sociological research into engineering practice, we can extract a few thought-provoking questions for ML developers to consider when building ML models and AI systems.
- Recognize that AI systems don’t just take in human behavior as input, but they also actively elicit some behaviors versus others. In other words, algorithms have the power to change and shape human behavior as users respond to the affordances or constraints of the system.
- How will your AI system potentially change human behavior or incentivize broader collective action?
- For example, could your product inadvertently create a new cottage industry, like SEO consultancies, to deal with the ambiguities of your product?
- Acknowledge that just as ethics is blurry, so is ethical AI. Moving away from binaries (AI is neither ethical nor unethical), we can instead build AI on a moral spectrum, that through its use, adaptation, and constant change in the deployment environment, creates more or less ethical behaviors, practices, and outcomes depending on the time and place of its use.
- In other words, in addition to considering “bad” or “good” user interactions with your system, what kind of behavior could be considered to fall in a morally ambiguous or grey area?
- Will that depend on the type of user or the time or place of their interaction with your product?
- In the SEO example, is trading links acceptable but paying for them is not? Is it ok if a struggling small business does it versus a big retailer like JC Penny?
- Consider the blurry lines between intended and unintended uses of an AI system during the design phase.Â
- How likely is it that your intended uses for the product align with those of your expected users?Â
- Broadening your view of relevant stakeholders, how might unexpected users of your product or types of users that don’t yet exist (e.g. SEO consultants) engage with your product?Â
- What mechanisms will you rely on to figure out if your system is being manipulated (e.g. New York Times exposés) and how will you impose fair penalties and mechanisms for appeal?
- Stepping back from the black and white thinking of “gaming the system,” consider the kind of “ethical work” that is expected of various stakeholders. Â
- How heavy is the burden of ethical work on some versus others?Â
- What kind of behaviors do you expect stakeholders to engage in to try to generate ethical clarity out of ethical ambiguity when interacting with your product?Â
- What is every stakeholder’s position of power(lessness) vis-Ă -vis that of the AI system, the company developing and maintaining the AI system, and the broader institutions within which the AI system will be deployed? Could some behaviors be a form of resistance to an existing unequal power relationship? If so, how can you distribute more agency to marginalized stakeholders to level the playing field?Â
- What systematic inequalities can your product potentially propagate within the ecosystem and how might your design compensate for that?Â
Conclusion
More and more, ML models are used to organize online information, rank and score human characteristics or behaviors, and police online interactions.
Despite being done by largely automated AI systems, this work, nonetheless, requires making value judgements about people and their behavior. And this means that there will always be moral grey areas that cannot be automated away with the next best ML model.
Ziewitz encourages us to think more deeply about how users will interact with our AI systems beyond the binary of good or bad behavior. He welcomes us to take the extra time to dwell in ambiguity and consider the ethical work that all humans will do as they interact with automated voice assistants, self-driving cars, child abuse prediction algorithms, or search engine ranking models.
There are no easy answers or one-size-fits-all solutions, but surely considering human behavior in all of its complexity will help us build better and more human-centered AI.
Original paper by Malte Ziewitz: https://doi.org/10.1177/0306312719865607