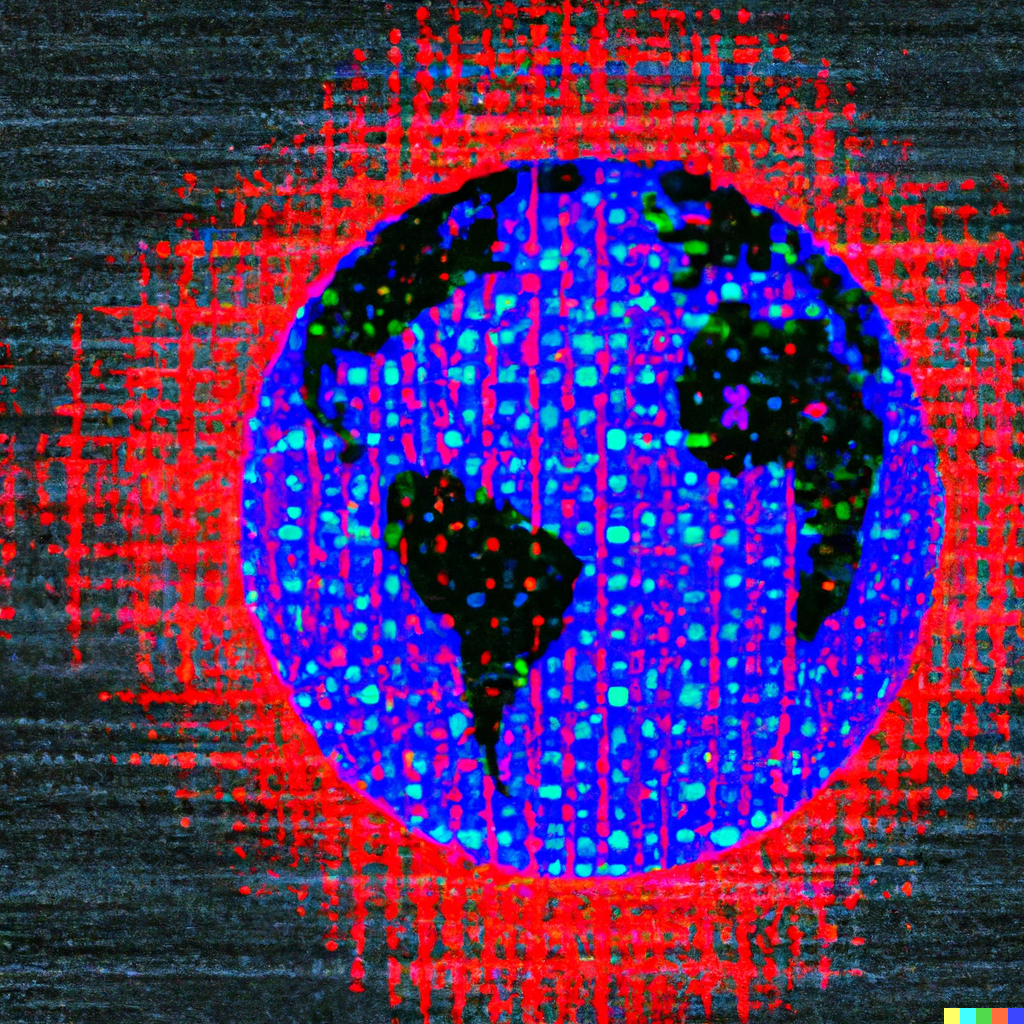
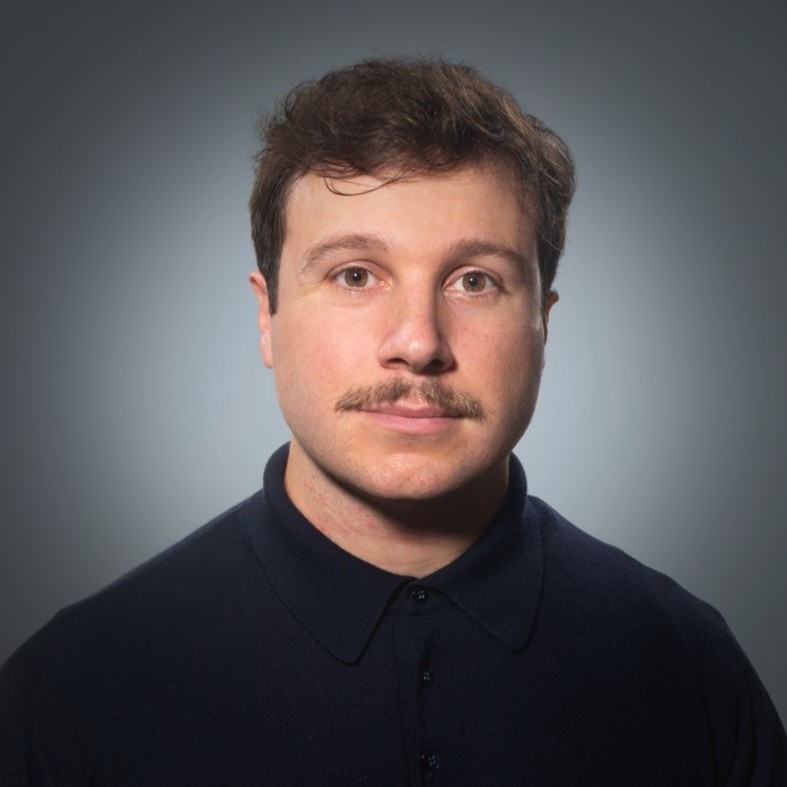
✍️ Column by Carlos Muñoz Ferrandis, RAIL Initiative, HuggingFace.
Acknowledgments to Yacine Jernite, Giada Pistilli, and Danish Contractor for their kind review and valuable feedback.
Overview: This article explores a new licensing paradigm in the AI space – Open & Responsible AI Licenses (Open RAIL) – from a social perspective as a social institution with the potential of setting future community norms for the respect and responsible sharing of AI artifacts.
The birth of a new licensing paradigm in the AI space
Contracts help us to be cooperative and trusting when we may otherwise be disobliging and distrusting.
Royal Swedish Academy of Science presenting the Nobel Prize for Oliver Hart’s and Bengt Holmström’s research on Contract Theory.
Imagine you develop a Machine Learning (ML) model, and you want to release it on an open basis. However, you feel concerned about what users could do with it, which could eventually lead to undesired and potentially harmful consequences due to the capabilities of your ML model.
Currently, the mainstream way to openly release your ML model is to pick an open-source license (for example, Apache, MIT, BSD, or GPL). Now think briefly: what message does an OSS license convey to the public who will inspect, access, and use your ML model?
“Do whatever you want with it, I don´t care?” Is this how you see the exchange of work and information between yourself and your users, or would you rather foster a sense of shared responsibility for what happens next?
While the above interpretation of the implications of an OSS license may be seen as caricatural, it is important to note that their context and general assumptions about how software is shared and used have shifted in the last decade – especially when the software relies on newer ML models. Open Source and Creative Commons licenses are icons of a modern licensing paradigm stemming from the knowledge-sharing economy. Both sets of licensing phenomena bring huge benefits to society at large. Notwithstanding the latter, none of these two licensing phenomena can be used in AI. They were not designed with ML models or datasets in mind. How can we extend this open paradigm to account for the specific properties of model weights and their differences from software code? And how do we answer recent calls to take more responsibility for these artifacts that can have both very positive and very negative impacts on people’s lives? There were almost no answers to this question a few months ago apart from closing access to the ML feature, potentially disincentivizing open innovation in the AI space.
BigScience brought one possible answer to these questions by creating the first embodiment of an OpenRAIL license designed for models and initially applied for the set of BLOOM models. OpenRAILs seek to balance open access and responsible use of AI artifacts. They enable free access, use, and distribution of derivatives of the AI artifact while requiring respect for a set of use-based restrictions both when using and distributing the AI artifact due to concerns from the licensor and their awareness of the technical capabilities and/or limitations of the artifact, informed by documentation such as model cards and/or data sheets.
Once the BLOOM RAIL license was released, we realized that recent major releases by Meta (namely OPT-175, SEER, BB3) also included licenses that could also be viewed as forms of Responsible AI Licenses. Consequently, we needed to set a naming convention that clearly distinguishes between RAIL and OpenRAIL and the specific AI artifact licenses: data; apps; model; source code. Once the basis was set, we released the BigScience OpenRAIL-M, generally applicable to any ML model for the AI community. In parallel, Stability.ai released Stable Diffusion with the CreativeML OpenRAIL, an adapted version from the BigScience BLOOM RAIL license.
Open licenses: the epitome of economic interactions among ICT market actors
Open software licenses can be conceived as social institutions settings the norms in specific communities and/or markets, see Widder et al. (2022). The license plays a core role. It carries the message from the licensor -e.g., an individual or a company – on how the licensed material can be used. Thus, the license is a carrier of norms to respect by the public when using the licensed material.
Over time, open software licenses, such as open-source licenses, have become a licensing standard among scientific communities and companies. These are nowadays massively adopted and have been standardized as social institutions governing the economic interactions between market actors. Each license represents a very specific set of economic interests transposed into a very specific set of clauses.
For instance, what is a licensor telling the public when releasing a software feature with a GPL2 license? The licensor wants the public to benefit from their innovation while requiring the public to share under the same terms their incremental innovation. In other words, the community gives you, and you give back to the community, a social tradeoff.
And what about an MIT license, which message is the licensor seeking to convey? The licensor is willing to share its innovation with the public to do whatever it wants with the licensed material. The only thing the licensor asks in return is to include a copyright notice and a copy of the license.
Licenses like GPL2 and MIT have become the de facto standard way of sharing software-related material in the Information and Communications Technologies (ICT) industry. Corollary to it, the messages conveyed by each license have transcended as community norms, as behavioral standards which, despite the specific legal terms present in the license, are widely understood and respected by most market actors. Consequently, it seems probable that when software developers choose a GPL license to release their code, they consider the GPL license as a set of values part of the software-sharing community that has to be respected. The developer chooses the license due to the message it conveys to the public as a community norm and value carrier.
OpenRAILs as informed value carriers
Open & Responsible AI licenses are also conceived and designed as value carriers. OpenRAILs were designed to include specific provisions enabling widespread adoption of the informed use restrictions embedded in the genesis license. These provisions require subsequent re-distributions of the licensed AI artifact or distributions of derivatives to include -at a minimum- the same use restrictions.
As a result, the set of informed restrictions, stemming from licensor’s concerns, are passed on from user to user, from license to license, all the way down the value chain. In the long run, this set of informed use restrictions will become a well-established community norm in the AI space, and users will know what values they have to respect when using an AI artifact licensed under a RAIL or OpenRAIL license. The aim is not to standardize values but rather how ethical concerns about the technical capabilities and limitations of AI artifacts can inform the open licensing of AI artifacts to foster new community norms around the respect of the licensed artifact employing use-based restrictions acting as informed value carriers.
OpenRAILs as an AI community institution enabling decentralized control of AI
OpenRAILs bring the ability to stand up for responsible use of the license not just to the original licensor but even more to all the subsequent users who will redistribute the licensed AI artifact or distribute a derivative version of it. Each of these distributions will have to include, at minimum, the exact use restrictions as the original license, and therefore each of these new licensors will be in a position to promote and enforce a responsible use of the AI artifacts, depending on their interest and capacity of enforcement.
Enforcement is not a duty. It is rather an ability that OpenRAILs provide to users in contrast with open source licenses through which the licensor will not be able to have any control whatsoever of the ML model they decide to release.
The decentralization of control of the use of AI artifacts calls for the AI community to collectively promote and enforce informed concerns carried out by OpenRAIL. OpenRAILs will not enable a maximal control capability of the subsequent uses of the AI artifact. However, they are a step towards optimizing control processes and action-driven efforts towards mitigating harms with the engagement of the AI community at large.