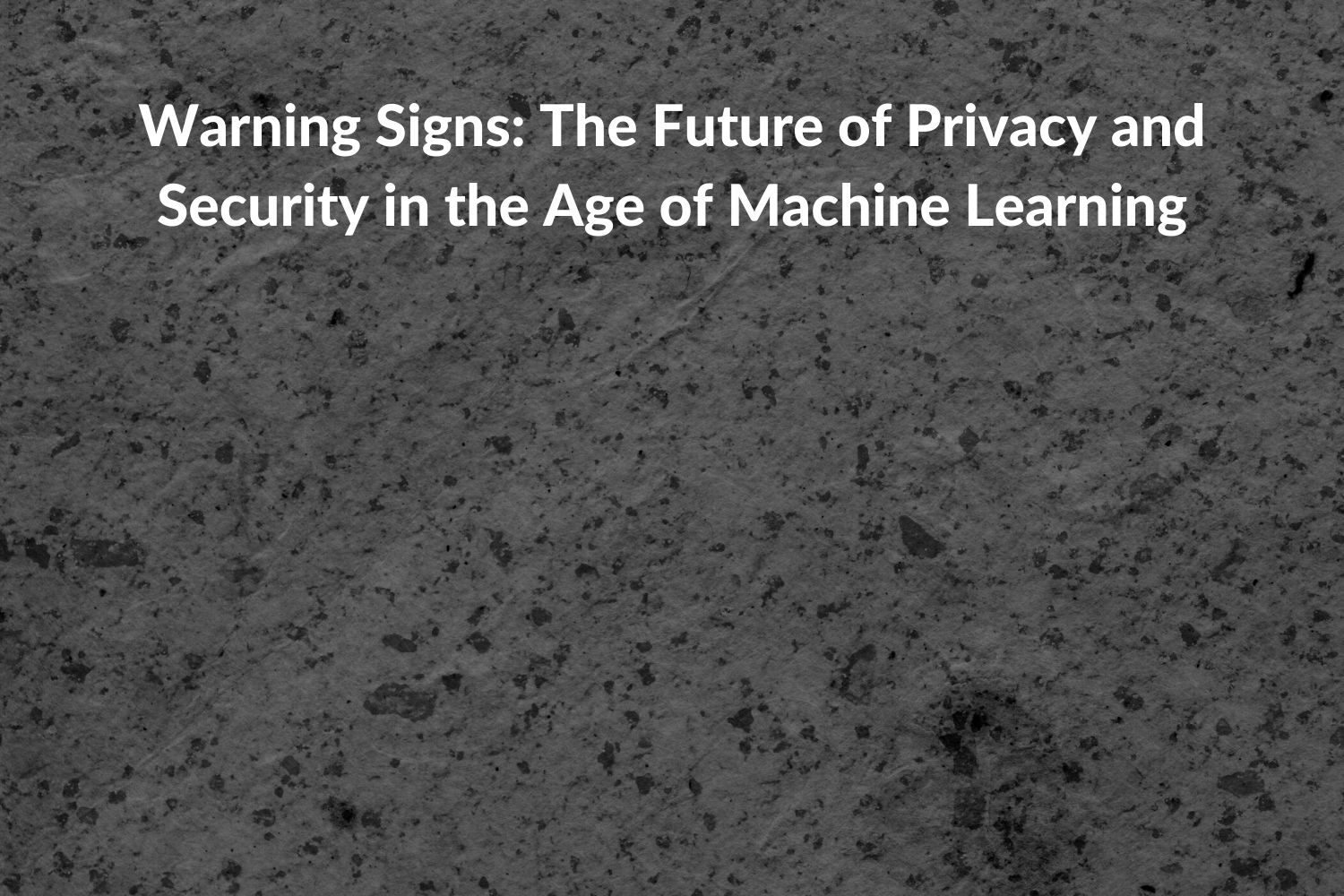
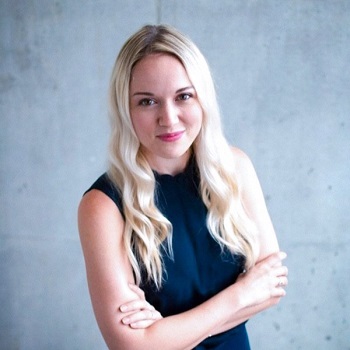
Summary contributed by Victoria Heath (@victoria_heath7), Communications Manager at Creative Commons
Authors of full paper: Sophie Stalla-Bourdillon, Brenda Leong, Patrick Hall, and Andrew Burt (link provided at the bottom)
There are no widely accepted best practices for mitigating security and privacy issues related to machine learning (ML) systems. Existing best practices for traditional software systems are insufficient because they’re largely based on the prevention and management of access to a system’s data and/or software, whereas ML systems have additional vulnerabilities and novel harms that need to be addressed. For example, one harm posed by ML systems is to individuals not included in the model’s training data but who may be negatively impacted by its inferences.
Harms from ML systems can be broadly categorized as informational harms and behavioral harms. Informational harms “relate to the unintended or unanticipated leakage of information.” The “attacks” that constitute informational harms are:
- Membership inference: Determining whether an individual’s data was utilized to train a model by examining a sample of the model’s output
- Model inversion: Recreating the data used to train the model by using a sample of its output
- Model extraction: Recreating the model itself by uses a sample of its output
Behavioral harms “relate to manipulating the behavior of the model itself, impacting the predictions or outcomes of the model.” The attacks that constitute behavioral harms are:
- Poisoning: Inserting malicious data into a model’s training data to change its behavior once deployed
- Evasion: Feeding data into a system to intentionally cause misclassification
Without a set of best practices, ML systems may not be widely and/or successfully adopted. Therefore, the authors of this white paper suggest a “layered approach” to mitigate the privacy and security issues facing ML systems. Approaches include noise injection, intermediaries, transparent ML mechanisms, access controls, model monitoring, model documentation, white hat or red team hacking, and open-source software privacy and security resources.
Finally, the authors note, it’s important to encourage “cross-functional communication” between data scientists, engineers, legal teams, business managers, etc. in order to identify and remediate privacy and security issues related to ML systems. This communication should be ongoing, transparent, and thorough.
Original paper by Sophie Stalla-Bourdillon, Brenda Leong, Patrick Hall, and Andrew Burt: https://fpf.org/wp-content/uploads/2019/09/FPF_WarningSigns_Report.pdf