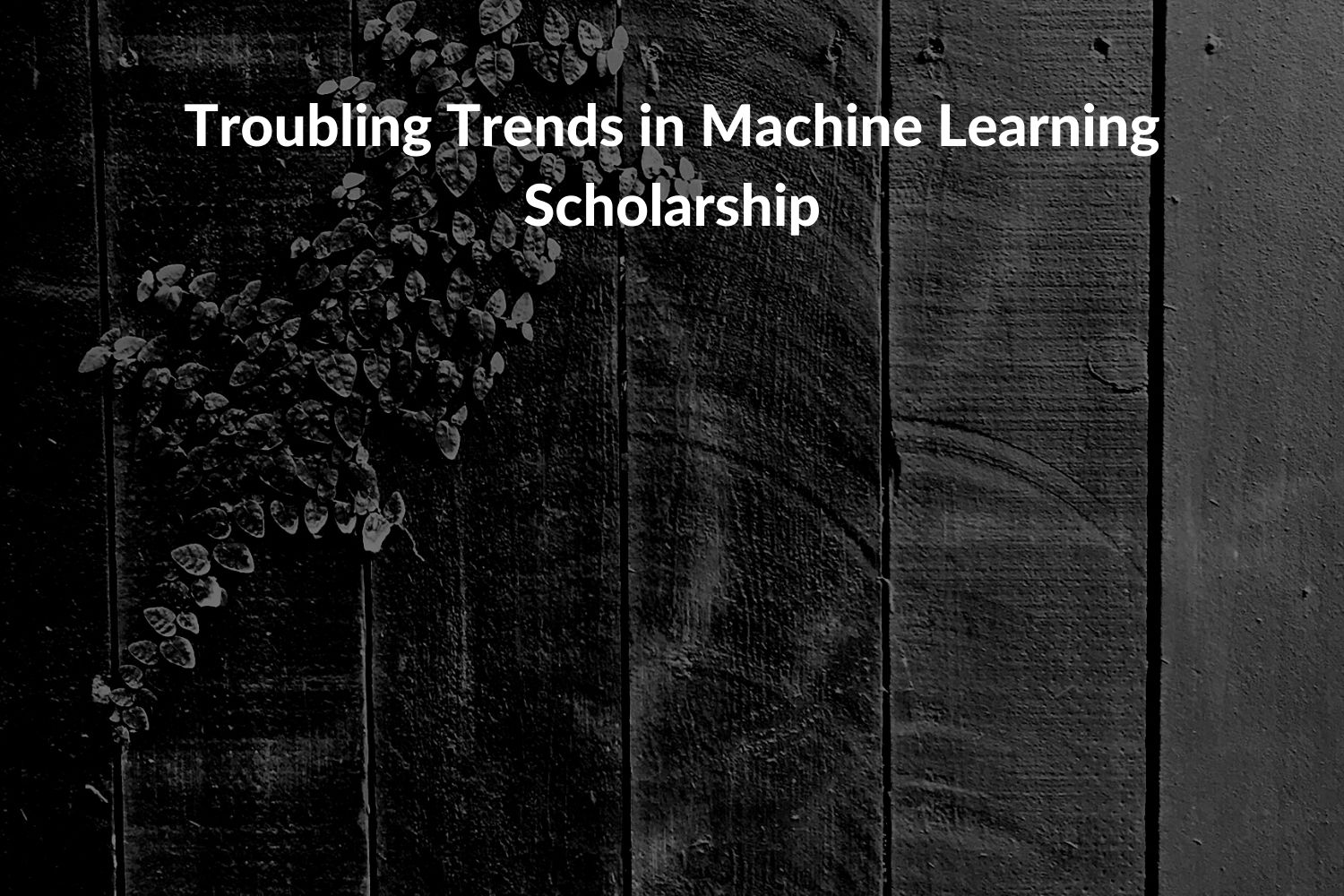
Mini summary (scroll down for full summary):
With the explosion of people working in the domain of ML and the prevalence of the use of preprint servers like arXiv, there are some troubling trends that have been identified by the authors of this paper when it comes to scholarship in ML. Specifically, the authors draw attention to some common issues that have become exacerbated in the field due to a thinning experienced reviewer pool who are increasingly burdened with larger numbers of papers to review and might need to default to checklist type of patterns in evaluating papers. A misalignment of incentives when it comes to communicating and expressing the results of the papers and the subject matter associated with them in terms that might draw the attention of investors and other entities who are not finely attuned to pick up flaws in scholarship. Lastly, a complacency in the face of progress whereby weak arguments are seen as acceptable in the face of strong quantitative and empirical evidence also makes this problem tough to handle.
The authors point to some common trends that they have observed such as the use of suitcase words that have multiple meanings and no consensus in how they are used which leads to researchers talking across each other. Conflation of terms and overloading existing technical definitions thus making results and the subject matter more impressive than it actually is also hampers the quality of scholarship. Using suggestive definitions where terms have other colloquial meanings and can be misconstrued to infer that the capabilities of the systems are far beyond what is actually presented is especially problematic when such research gets picked up by journalists and policymakers who use these to make decisions that are ill-informed.
Finally, the authors do provide recommendations on how this can be improved by sharing that authors should consider why they get certain results, what they mean more so focusing on just how they got to the results. Trying to avoid these anti-patterns in scholarship and practicing diligence and critical assessment prior to submitting work to be considered for publishing will also help counter the negative impacts. For those that are reviewing such work, the guidance is to cut through the jargon, unnecessary use of math, anthropomorphization to exaggerate results, and critically asking the question why the authors arrived at results and evaluating arguments for strength and cohesion rather than just looking at empirical findings that compete in producing better SOTA results will also help to alleviate the problem.
Given that the field has seen a resurgence in publication and that the impacts from this are widespread, the authors of this paper call on the community to aspire to higher quality of scholarship and do their part in minimizing the unintended consequences that occur when you have poor quality of scholarship circulating.
Full summary:
Authors of research papers aspire to achieving any of the following goals when writing papers: to theoretically characterize what is learnable, to obtain understanding through empirically rigorous experiments, or to build working systems that have high predictive accuracy. To communicate effectively with the readers, the authors must: provide intuitions to aid the readers’ understanding, describe empirical investigations that consider and rule out alternative hypotheses, make clear the relationship between theoretical analysis and empirical findings, and use clear language that doesn’t conflate concepts or mislead the reader.
The authors of this paper find that there are 4 areas where there are concerns when it comes to ML scholarship: failure to distinguish between speculation and explanation, failure to identify the source of empirical gains, the use of mathematics that obfuscates or impresses rather than clarifies, and misuse of language such that terms with other connotations are used or by overloading terms with existing technical definitions.
Flawed techniques and communication methods will lead to harm and wasted resources and efforts hindering the progress in ML and hence this paper provides some very practical guidance on how to do this better. When presenting speculations or opinions of authors that are exploratory and don’t yet have scientific grounding, having a separate section that quarantines the discussion and doesn’t bleed into the other sections that are grounded in theoretical and empirical research helps to guide the reader appropriately and prevents conflation of speculation and explanation. The authors provide the example of the paper on dropout regularization that made comparisons and links to sexual reproduction but limited that discussion to a “Motivation” section.
With a persistent pursuit for achieving SOTA results, there is a lot of tweaking that happens to realize gains in model performance and often there are many different techniques applied in tandem. From a reader’s perspective, elucidating clearly what the necessary sources of the realized gains are, disentangling it from other measures is essential. The authors highlight how a lot of the gains happen due to clever problem formulations, scientific experiments, applying existing techniques in a novel manner to new areas, optimization heuristics, extensive hyperparameter tuning, data preprocessing techniques and any number of other techniques. Absent proper ablation studies, sometimes research paper authors can obfuscate the real source of the gains. Sometimes careful studies that make use of ablation can highlight challenges in existing challenge datasets and benchmark datasets which can point the community towards more promising research directions.
Using mathematics in a manner where natural language and mathematical expositions are intermixed without a clear link between the two leads to weakness in the overall contribution. Specifically, when natural language is used to overcome weaknesses in the mathematical rigor and conversely, mathematics is used as a scaffolding to prop up weak arguments in the prose and give the impression of technical depth, it leads to poor scholarship and detracts from the scientific seriousness of the work and harms the readers. Additionally, invoking theorems with dubious pertinence to the actual content of the paper or in overly broad ways also takes away from the main contribution of a paper.
In terms of misuse of language, the authors of this paper provide a convenient ontology breaking it down into suggestive definitions, overloaded terminology, and suitcase words. In the suggestive definitions category, the authors coin a new technical term that has suggestive colloquial meanings and can slip through some implications without formal justification of the ideas in the paper. This can also lead to anthropomorphization that creates unrealistic expectations about the capabilities of the system. This is particularly problematic in the domain of fairness and other related domains where this can lead to conflation and inaccurate interpretation of terms that have well-established meanings in the domains of sociology and law for example. This can confound the initiatives taken up by both researchers and policymakers who might use this as a guide.
Overloading of technical terminology is another case where things can go wrong when terms that have historical meanings and they are used in a different sense. For example, the authors talk about deconvolutions which formally refers to the process of reversing a convolution but in recent literature has been used to refer to transpose convolutions that are used in auto-encoders and GANs. Once such usage takes hold, it is hard to undo the mixed usage as people start to cite prior literature in future works. Additionally, combined with the suggestive definitions, we run into the problem of concealing a lack of progress, such as the case with using language understanding and reading comprehension to now mean performance on specific datasets rather than the grand challenge in AI that it meant before.
Another case that leads to overestimation of the ability of these systems is in using suitcase words which pack in multiple meanings within them and there isn’t a single agreed upon definition. Interpretability and generalization are two such terms that have looser definitions and more formally defined ones, yet because papers use them in different ways, it leads to miscommunication and researchers talking across each other.
The authors identify that these problems might be occurring because of a few trends that they have seen in the ML research community. Specifically, complacency in the face of progress where there is an incentive to excuse weak arguments in the face of strong empirical results and the single-round review process at various conferences where the reviewers might not have much choice but to accept the paper given the strong empirical results. Even if the flaws are noticed, there isn’t any guarantee that they are fixed in a future review cycle at another conference.
As the ML community has experienced rapid growth, the problem of getting high-quality reviews has been exacerbated: in terms of the number of papers to be reviewed by each reviewer and the dwindling number of experienced reviewers in the pool. With the large number of papers, each reviewer has less time to analyze papers in depth and reviewers who are less experienced can fall easily into some of the traps that have been identified so far. Thus, there are two levers that are aggravating the problem. Additionally, there is the risk of even experienced researchers resorting to a checklist-like approach under duress which might discourage scientific diversity when it comes to papers that might take innovative or creative approaches to expressing their ideas.
A misalignment in incentives whereby lucrative deals in funding are offered to AI solutions that utilize anthropomorphic characterizations as a mechanism to overextend their claims and abilities though the authors recognize that the causal direction might be hard to judge.
The authors also provide suggestions for other authors on how to evade some of these pitfalls: asking the question of why something happened rather than just relying on how well a system performed will help to achieve the goal of providing insights into why something works rather than just relying on headline numbers from the results of the experiments. They also make a recommendation for insights to follow the lines of doing error analysis, ablation studies, and robustness checks and not just be limited to theory.
As a guideline for reviewers and journal editors, making sure to strip out extraneous explanations, exaggerated claims, changing anthropomorphic naming to more sober alternatives, standardizing notation, etc. should help to curb some of the problems. Encouraging retrospective analysis of papers is something that is underserved at the moment and there aren’t enough strong papers in this genre yet despite some avenues that have been advocating for this work.
Flawed scholarship as characterized by the points as highlighted here not only negatively impact the research community but also impact the policymaking process that can overshoot or undershoot the mark. An argument can be made that setting the bar too high will impede new ideas being developed and slow down the cycle of reviews and publication while consuming precious resources that could be deployed in creating new work. But, asking basic questions to guide us such as why something works, in which situations it does not work, and have the design decisions been justified will lead to a higher quality of scholarship in the field.
Original piece by Zachary Lipton and Jacob Steinhardt: https://arxiv.org/abs/1807.03341