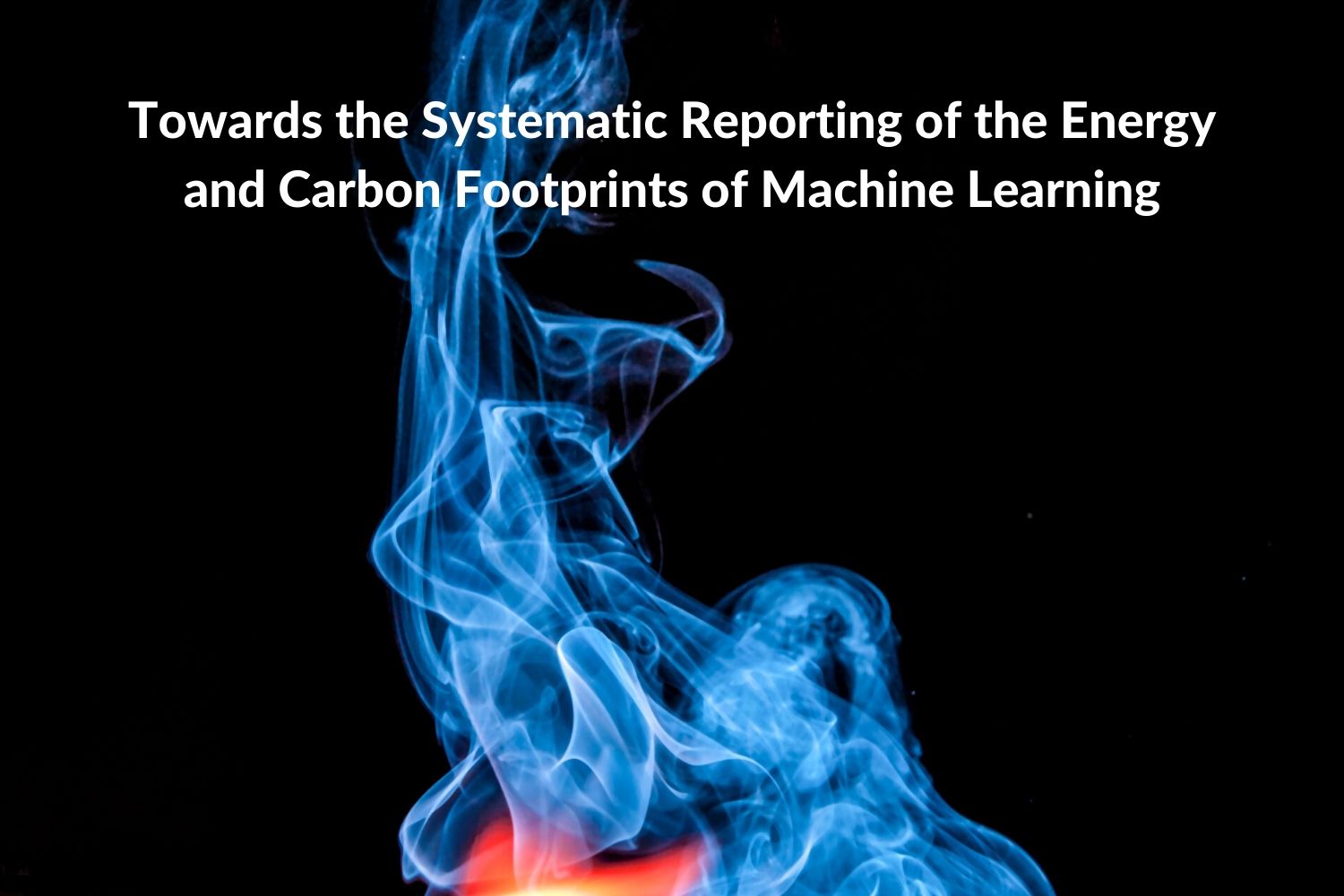
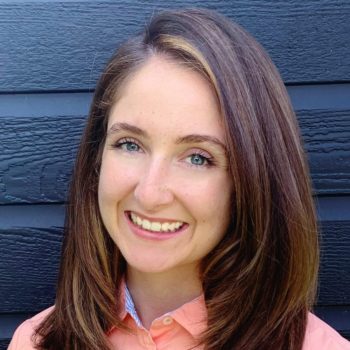
Summary contributed by Camylle Lanteigne (@CamLante), who’s currently pursuing a Master’s in Public Policy at Concordia University and whose work on social robots and empathy has been featured on Vox.
*Authors of original paper & link at the bottom
Climate change and environmental destruction are well-documented. Most people are aware that mitigating the risks caused by these is crucial and will be nothing less than a Herculean undertaking. On the bright side, AI can be of great use in this endeavour. For example, it can help us optimize resource use, or help us visualize the devastating effects of floods caused by climate change.
However, AI models can have excessively large carbon footprints. Henderson et al.’s paper details how the metrics needed to calculate environmental impact are severely underreported. To highlight this, the authors randomly sampled one-hundred NeuRIPS 2019 papers. They found that none reported carbon impacts, only one reported some energy use metrics, and seventeen reported at least some metrics related to compute-use. Close to half of the papers reported experiment run time and the type of hardware used. The authors suggest that the environmental impact of AI and relevant metrics are hardly reported by researchers because the necessary metrics can be difficult to collect, while subsequent calculations can be time-consuming.
Taking this challenge head-on, the authors make a significant contribution by performing a meta-analysis of the very few frameworks proposed to evaluate the carbon footprint of AI systems through compute- and energy-intensity. In light of this meta-analysis, the paper outlines a standardized framework called experiment-impact-tracker to measure carbon emissions. The authors use 13 metrics to quantify compute and energy use. These include when an experiment starts and ends, CPU and GPU power draw, and information on a specific energy grid’s efficiency.
The authors describe their motivations as threefold. First, experiment-impact-tracker is meant to spread awareness among AI researchers about how environmentally-harmful AI can be. They highlight that “[w]ithout consistent and accurate accounting, many researchers will simply be unaware of the impacts their models might have and will not pursue mitigating strategies”. Second, the framework could help align incentives. While it is clear that lowering one’s environmental impact is generally valued in society, this is not currently the case in the field of AI. Experiment-impact tracker, the authors believe, could help bridge this gap, and make energy efficiency and carbon-impact curtailment valuable objectives for researchers, along with model accuracy and complexity. Third, experiment-impact-tracker can help perform cost-benefit analyses on one’s AI model by comparing electricity cost and expected revenue, or the carbon emissions saved as opposed to those produced. This can partially inform decisions on whether training a model or improving its accuracy is worth the associated costs.
To help experiment-impact-tracker become widely used among researchers, the framework emphasizes usability. It aims to make it easy and quick to calculate the carbon impact of an AI model. Through a short modification of one’s code, experiment-impact-tracker collects information that allows it to determine the energy and compute required as well as, ultimately, the carbon impact of the AI model. Experiment-impact-tracker also addresses the interpretability of the results by including a dollar amount that represents the harm caused by the project. This may be more tangible for some than emissions expressed in the weight of greenhouse gases released or even in CO2 equivalent emissions (CO2eq). In addition, the authors strive to: allow other ML researchers to add to experiment-impact-tracker to suit their needs, increase reproducibility in the field by making metrics collection more thorough, and make the framework robust enough to withstand internal mistakes and subsequent corrections without compromising comparability.
Moreover, the paper includes further initiatives and recommendations to push AI researchers to curtail their energy use and environmental impact. For one, the authors take advantage of the already widespread use of leaderboards in the AI community. While existing leaderboards are largely targeted towards model accuracy, Henderson et al. instead put in place an energy efficiency leaderboard for deep reinforcement learning models. They assert that a leaderboard of this kind, that tracks performance in areas indicative of potential environmental impact, “can also help spread information about the most energy and climate-friendly combinations of hardware, software, and algorithms such that new work can be built on top of these systems instead of more energy-hungry configurations”.
The authors also suggest AI practitioners can take an immediate and significant step in lowering the carbon emissions of their work: run experiments on energy grids located in carbon-efficient cloud regions like Quebec, the least carbon-intensive cloud region. Especially when compared to very carbon-intensive cloud regions like Estonia, the difference in CO2eq emitted can be considerable: running an experiment in Estonia produces up to thirty times as much emissions as running the same experiment in Quebec. The important reduction in carbon emissions that follows from switching to energy-efficient cloud regions, according to Henderson et al., means there is no need to fully forego building compute-intensive AI as some believe.
In terms of systemic changes that accompany experiment-impact-tracker, the paper lists seven. The authors suggest the implementation of a “green default” for both software and hardware. This would make the default setting for researchers’ tools the most environmentally-friendly one. The authors also insist on weighing costs and benefits to using compute- and energy-hungry AI models. Small increases in accuracy, for instance, can come at a high environmental cost. They hope to see the AI community use efficient testing environments for their models, as well as standardized reporting of a model’s carbon impact with the help of experiment-impact-tracker.
Additionally, the authors ask those developing AI models to be conscious of the environmental costs of reproducing their work, and act as to minimize unnecessary reproduction. While being able to reproduce other researchers’ work is crucial in maintaining sound scientific discourse, it is merely wasteful for two departments in the same business to build the same model from scratch. The paper also presents the possibility of developing a badge identifying AI research papers that show considerable effort in mitigating carbon impact when these papers are presented at conferences. Lastly, the authors highlight important lacunas in relation to driver support and implementation. Systems that would allow data on energy use to be collected are unavailable for certain hardware, or the data is difficult for users to obtain. Addressing these barriers would allow for more widespread collection of energy use data, and contribute to making carbon impact measurement more mainstream in the AI community.
Original paper by Henderson et al. (Henderson, P., Hu, J., Romoff, J., Brunskill, E., Jurafsky, D., & Pineau, J.): https://arxiv.org/pdf/2002.05651.pdf