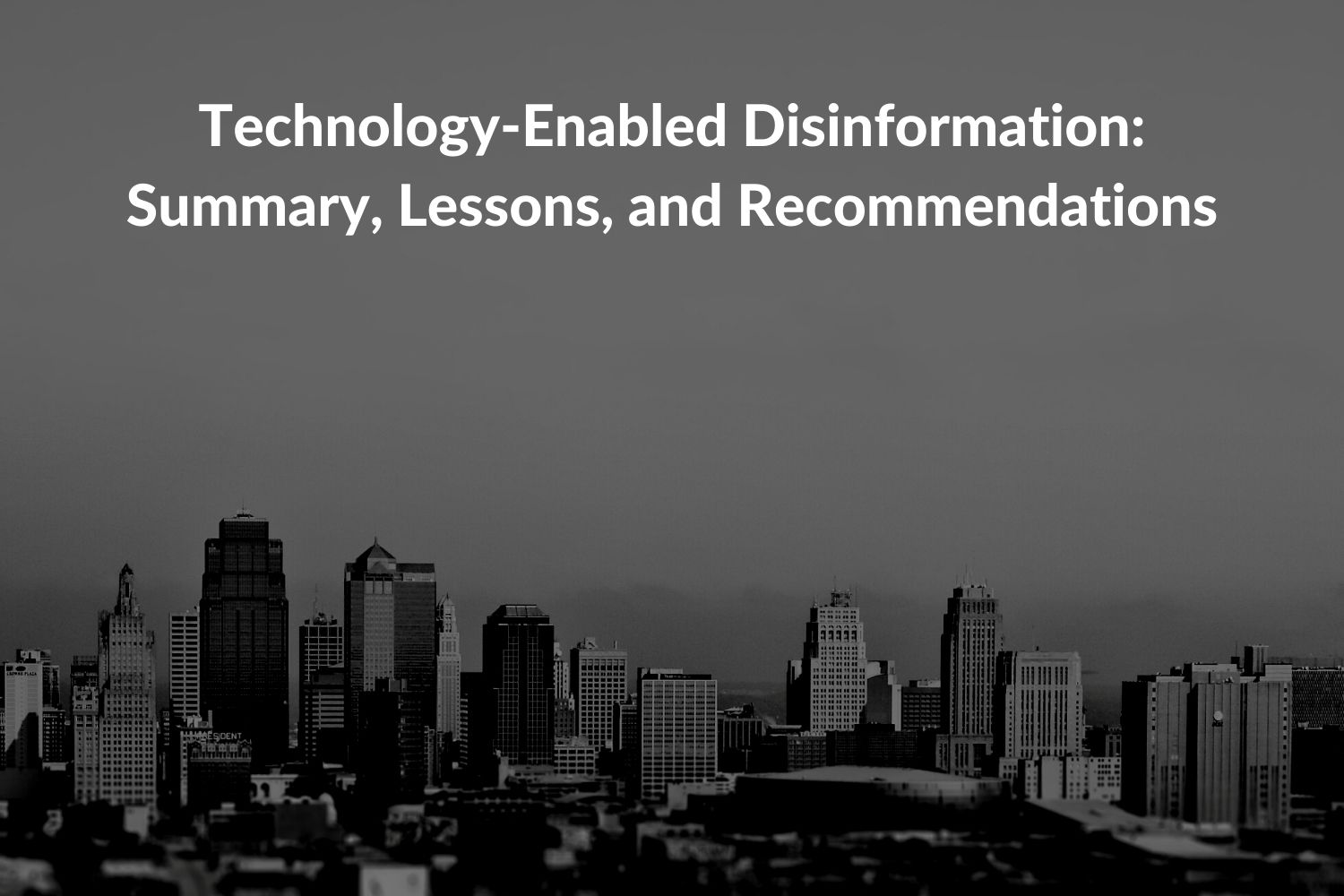
Mini summary (scroll down for full summary):
The upcoming election cycle and the current pandemic have made all of us into inveterate consumers of copious amounts of information from a variety of sources. For a lot of people, their primary source of information is social media where they rely not only on the official accounts of large media organizations but also on content that is shared by people they know and they don’t know. There is an increased importance of being able to successfully combat mis/disinformation, especially when it means that one can achieve better health outcomes and safeguard the state of our democracies.
This paper provides a comprehensive overview of the state of the mis/disinformation ecosystem providing guidance on specific technical and design interventions that help us build a better understanding of the disinformation ecosystem. It also brings forth the dire need for interdisciplinary collaboration highlighting the challenges that researchers face today when analyzing some of the platforms that operate on closed-network models. Most research today focuses on Twitter because of its public nature but there is a need to get deeper insights into the state of how information flows in closed groups and how it is perceived by users. There is also an explanation offered into how novel technology is accelerating the pace and impact of disinformation campaigns and how the variation in the motivations of the actors necessitates tailored defenses.
The ecosystem is inherently adversarial, just as in the world of cybersecurity, and hence being successful today in detecting and combating disinformation doesn’t mean that we can do so tomorrow. The constant evolution in understanding of the ecosystem coupled with redesigning some of the affordances on the platforms that better meet the needs of diverse users is crucial. We need to build defenses that don’t just cater to those who are technically-literate and easing the burden of detecting disinformation from the shoulders of users is important because the onus needs to be shared by the platforms and the regulators as well.
Recommendations are also made for how policymakers can meaningfully engage in this space and how educational initiatives for the users can help increase the quality of interactions on the platform. Ultimately, tailored approaches that rely on interdisciplinary expertise attacking the root causes of the spread of disinformation by analyzing the sources, plumbing of platforms that permits rapid dissemination, the motivations and incentives of the various actors in the ecosystem and the perception issues on the end of the users need to be addressed in a piecewise, tangible manner such that the problems are tractable and the solutions to those problems can then be combined into a pipeline to more comprehensively mitigate the harm from disinformation.
Full summary:
There is a distinction between misinformation and disinformation – misinformation is the sharing of false information unintentionally where no harm is intended whereas disinformation is false information that is spread intentionally with the aims of causing harm to the consumers. This is also referred to as information pollution and fake news. It has massive implications that have led to real harms for people in many countries with one of the biggest examples being the polarization of views in the 2016 US Presidential elections.
Meaningful solutions to this will only emerge when we have researchers from both technical and social sciences backgrounds working together to gain a deeper understanding of the root causes. This isn’t a new problem and has existed for a very long time, it’s just that with the advent of technology and more people being connected to each other we have a much more rapid dissemination of the false information and modern tools enable the creation of convincing fake images, text and videos, thus amplifying the negative effects.
Some of the features that help to delve deeper into the study of how mis/disinformation spreads are:
- Democratization of content creation: with practically anyone now having the ability to create and publish content, information flow has increased dramatically and there are few checks for the veracity of content and even fewer mechanisms to limit the flow rate of information.
- Rapid news cycle and economic incentives: with content being monetized, there is a strong incentive to distort information to evoke a response from the reader such that they click through and feed the money-generating apparatus.
- Wide and immediate reach and interactivity: by virtue of almost the entire globe being connected, content quickly reaches the furthest corners of the planet. More so, content creators are also able to, through quantitative experiments, determine what kind of content performs well and then tailor that to feed the needs of people.
- Organic and intentionally created filter bubbles: the selection of who to follow along with the underlying plumbing of the platforms permits for the creation of echo chambers that further strengthen polarization and do little to encourage people to step out and have a meaningful exchange of ideas.
- Algorithmic curation and lack of transparency: the inner workings of platforms are shrouded under the veil of IP protections and there is little that is well-known about the manipulative effects of the platforms on the habits of content consumers.
- Scale and anonymity of online accounts: given the weak checks for identity, people are able to mount “sybil” attacks that leverage this lack of strong identity management and are able to scale their impact through the creation of content and dispersion of content by automated means like bot accounts on the platform.
What hasn’t changed even with the introduction of technology are the cognitive biases which act as attack surfaces for malicious actors to inject mis/disinformation. This vulnerability is of particular importance in the examination and design of successful interventions to combat the spread of false information. For example, the confirmation bias shows that people are more likely to believe something that conforms with their world-view even if they are presented with overwhelming evidence to the contrary. In the same vein, the backfire effect demonstrates how people who are presented with such contrary evidence further harden their views and get even more polarized thus negating the intention of presenting them with balancing information.
In terms of techniques, the adversarial positioning is layered into three tiers with spam bots that push out low-quality content, quasi-bots that have mild human supervision to enhance the quality of content and pure human accounts that aim to build up a large following before embarking on spreading the mis/disinformation.
From a structural perspective, the alternate media sources often copy-paste content with source attribution and are tightly clustered together with a marked separation with other mainstream media outlets. On the consumer front, there is research that points to the impact that structural deficiencies in the platforms, say Whatsapp where source gets stripped out in sharing information, create not only challenges for researchers trying to study the ecosystem but also exacerbate the local impact effect whereby a consumer trusts things coming from friends much more so than other potentially more credible sources from an upstream perspective.
Existing efforts to study the ecosystem require a lot of manual effort but there is hope in the sense that there are some tools that help automate the analysis. As an example, we have the Hoaxy tool, a tool that collects online mis/disinformation and other articles that are fact-checking versions. Their creators find that the fact-checked articles are shared much less than the original article and that curbing bots on a platform has a significant impact.
There are some challenges with these tools in the sense that they work well on public platforms like Twitter but on closed platforms with limited ability to deploy bots, automation doesn’t work really well. Additionally, even the metrics that are surfaced need to be interpreted by researchers and it isn’t always clear how to do that.
The term deepfake originated in 2017 and since then a variety of tools have been released such as Face2Face that allow for the creation of reanimations of people to forge identity, something that was alluded to in this paper here on the evolution of fraud. While being able to create such forgeries isn’t new, what is new is that this can be done now with a fraction of the effort, democratizing information pollution and casting aspersions on legitimate content as one can always argue something was forged.
Online tracking of individuals, which is primarily used for serving personalized advertisements and monetizing the user behaviors on websites can also be used to target mis/disinformation in a fine-grained manner. There are a variety of ways this is done through third-party tracking like embedding of widgets to browser cookies and fingerprinting. This can be used to manipulate vulnerable users and leverage sensitive attributes gleaned from online behaviors that give malicious actors more ammunition to target individuals specifically. Even when platforms provide some degree of transparency on why users are seeing certain content, the information provided is often vague and doesn’t do much to improve the understanding for the user.
Earlier attempts at using bots used simplistic techniques such as tweeting at certain users and amplifying low-credibility information to give the impression that something has more support than it really does but recent attempts have become more sophisticated: social spambots. These slowly build up credibility within a community and then use that trust to sow disinformation either automatically or in conjunction with a human operator, akin to a cyborg.
Detection and measurement of this problem is a very real concern and researchers have tried using techniques like social network graph structure, account data and posting metrics, NLP on content and crowdsourcing analysis. From a platform perspective, they can choose to analyze the amount of time spent browsing posts vs. the time spent posting things.
There is an arms race between detection and evasion of bot accounts: sometimes even humans aren’t able to detect sophisticated social bots. Additionally, there are instances where there are positive and beneficial bots such as those that aggregate news or help coordinate disaster response which further complicates the detection challenge. There is also a potential misalignment in incentives since the platforms have an interest in having higher numbers of accounts and activity since it helps boost their valuations while they are the ones that have the maximum amount of information to be able to combat the problem.
This problem of curbing the spread of mis/disinformation can be broken down into two parts: enabling detection on the platform level and empowering readers to select the right sources. We need a good definition of what fake news is, one of the most widely accepted definitions is that it is something that is factually false and intentionally misleading. Framing a machine learning approach here as an end-to-end task is problematic because it requires large amounts of labelled data and with neural network based approaches, there is little explanation offered which makes downstream tasks harder.
So we can approach this by breaking it down into subtasks, one of which is verifying the veracity of information. Most current approaches use human fact-checkers but this isn’t a scalable approach and automated means using NLP aren’t quite proficient at this task yet. There are attempts to break down the problem even further such as using stance detection to see if information presented agrees, disagrees or is unrelated to what is mentioned in the source. Other approaches include misleading style detection whereby we try to determine if the style of the article can offer clues to the intent of the author but that is riddled with problems of not having necessarily a strong correlation with a misleading intent because the style may be pandering to hyperpartisanship or even if it is neutral that doesn’t mean that it is not misleading.
Metadata analysis looking at the social graph structure, attributes of the sharer and propagation path of the information can lend some clues as well. While all these attempts have their own challenges and in the arms race framing, there is a constant battle between attack and defense, even if the problem is solved, we still have human cognitive biases which muddle the impacts of these techniques. UX and UI interventions might serve to provide some more information as to combating those.
As a counter to the problems encoun tered in marking content as being “disputed” which leads to the implied truth effect leading to larger negative externalities, an approach is to show “related” articles when something is disputed and then use that as an intervention to link to fact-checking websites like Snopes. Other in-platform interventions include the change from Whatsapp to show “forwarded” next to messages so that people had a bit more insight into the provenance of the message because there was a lot of misinformation that was being spread in private messaging. There are also third-party tools like SurfSafe that are able to check images as people are browsing against other websites where they might have appeared and if they haven’t appeared in many places, including verified sources, then the user can infer that the image might be doctored.
Education initiatives by the platform companies for users to spot misinformation are a method to get people to become more savvy. There have also been attempts to assign nutrition labels to sources to list their slant, tone of the article, timeliness of the article and the experience of the author which would allow a user to make a better decision on whether or not to trust an article. Platforms have also attempted to limit the spread of mis/disinformation by flagging posts that encourage gaming of the sharing mechanisms on the platform, for example, downweighting posts that are “clickbait”.
The biggest challenges in the interventions created by the platforms themselves are that they don’t provide sufficient information as to make the results scientifically reproducible. Given the variety of actors and motivations, the interventions need to be tailored to be able to combat them such as erecting barriers to the rate of transmission of mis/disinformation and demonetization for actors with financial incentives but for state actors, detection and attribution might be more important. Along with challenges in defining the problem, one must look at socio-technical solutions because the problem has more than just the technical component, including the problem with human cognitive biases.
Being an inherently adversarial setting, it is important to see that not all techniques being used by the attackers are sophisticated, some simple techniques when scaled are just as problematic and require attention. But, given that this is constantly evolving, detecting disinformation today doesn’t mean that we can do so successfully tomorrow. Additionally, disinformation is becoming more personalized, more realistic and more widespread.
There is a misalignment in incentives as explored earlier in terms of what the platforms want and what’s best for users but also that empowering users to the point of them being just skeptical of everything isn’t good either, we need to be able to trigger legitimate and informed trust in the authentic content and dissuade them away from the fake content.
Among the recommendations proposed by the authors are: being specific about what a particular technological or design intervention means to achieve, breaking down the technological problems into smaller, concrete subproblems that have tractable solutions and then recombining them into the larger pipeline. We must also continue to analyze the state of the ecosystem and tailor defenses such that they can combat the actors at play. Additionally, rethinking of the monetary incentives on the platform can help to dissuade some of the financially-motivated actors.
Educational interventions that focus on building up knowledge so that there is healthy skepticism and learning how to detect markers for bots, the capabilities of technology to create fakes today and discussions in “public squares” on this subject are crucial yet we mustn’t place too much of a burden on the end-user that distracts them from their primary task which is interaction with others on the social network. If that happens, people will just abandon the effort. Additionally, designing for everyone is crucial, if the interventions, such as installing a browser extension, are complicated, then one can only reach the technically-literate people and everyone else gets left out.
On the platform end, apart from the suggestions made above, they should look at the use of design affordances that aid the user in judging the veracity, provenance and other measures to discern legitimate information vs. mis/disinformation. Teaming up with external organizations that specialize in UX/UI research will aid in understanding the impacts of the various features within the platform. Results from such research efforts need to be made public and accessible to non-technical audiences. Proposed solutions also need to be interdisciplinary to have a fuller understanding of the root causes of the problem. Also, just as we need tailoring for the different kinds of adversaries, it is important to tailor the interventions to the various user groups who might have different needs and abilities.
The paper also makes recommendations for policymakers, most importantly that the work in regulations and legislations be grounded in technical realities that are facing the ecosystem so that they don’t undershoot or overshoot the needs for successfully combating mis/disinformation. For users, there are a variety of recommendations provided in the references but notably being aware of our own cognitive biases and having a healthy degree of skepticism and checking information against multiple sources before accepting it as legitimate are the most important ones.
Original piece by Akers et al.: https://arxiv.org/abs/1812.09383