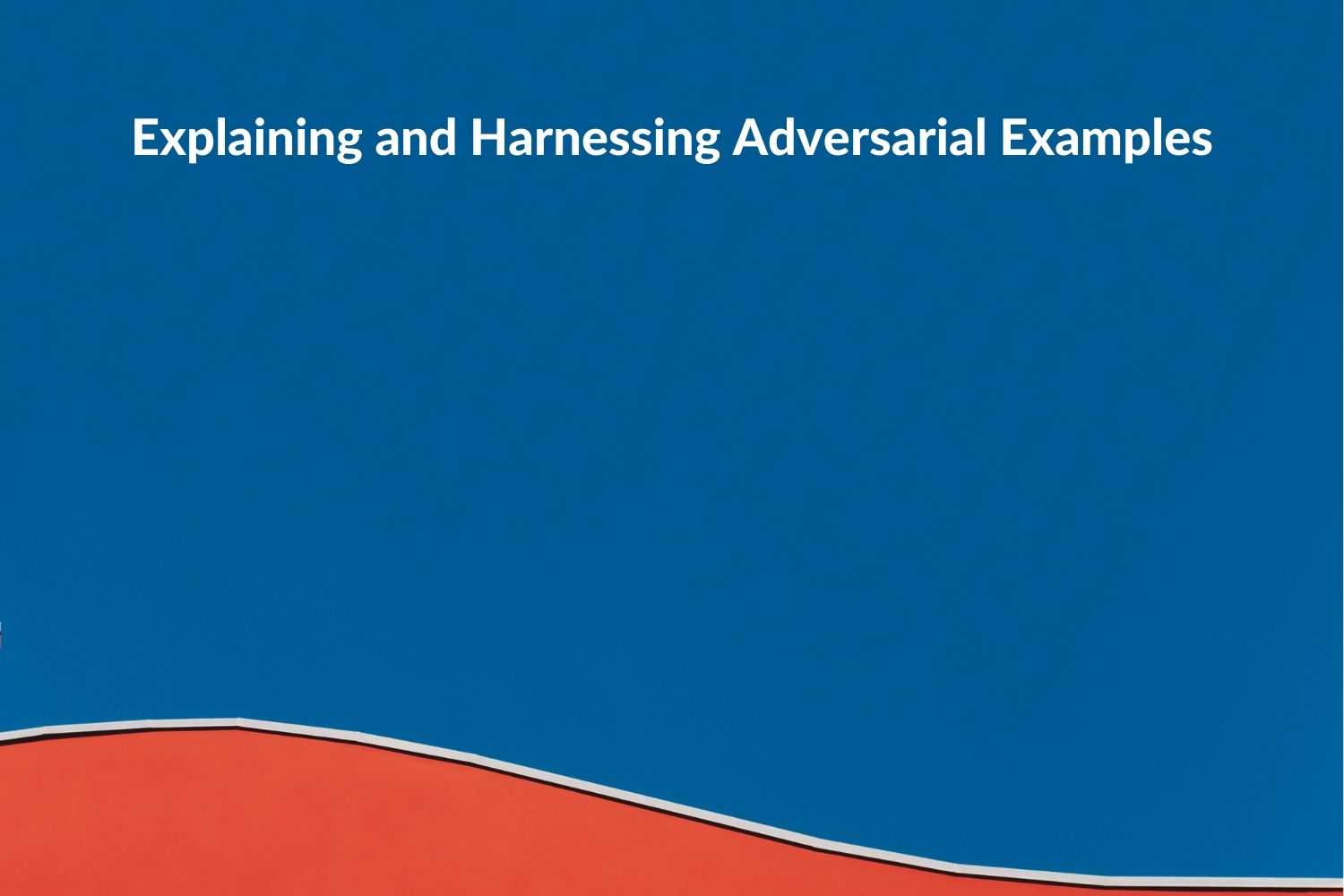
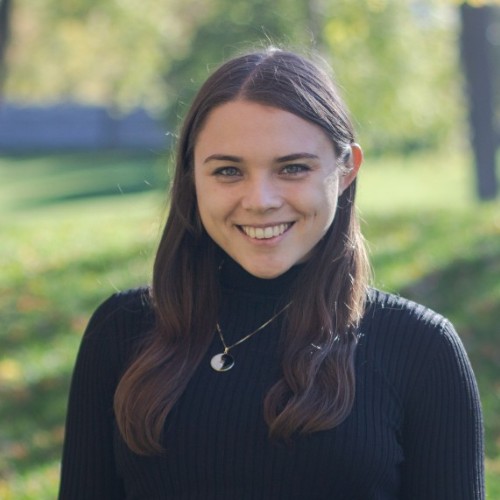
Summary contributed by Shannon Egan, Research Fellow at Building 21 and pursuing a master’s in physics at UBC.
*Author & link to original paper at the bottom.
Click here for the FULL summary in PDF form
(Short-form summary below)
A bemusing weakness of many supervised machine learning (ML) models, including neural networks (NNs), are adversarial examples (AEs). AEs are inputs generated by adding a small perturbation to a correctly-classified input, causing the model to misclassify the resulting AE with high confidence. Goodfellow et al. propose a linear explanation of AEs, in which the vulnerability of ML models to AEs is considered a by-product of their linear behaviour and high-dimensional feature space. In other words, small perturbations on an input can alter its classification because the change in NN activation (as result of the perturbation) scales with the size of the input vector.
Identifying ways to effectively handle AEs is of interest for problems like image classification, where the input consists of intensity data for many thousands of pixels. A method of generating AEs called “fast gradient sign method” badly fools a maxout network, leading to a 89.4% error rate on a perturbed MNIST test set. The authors propose an “adversarial training” scheme for NNs, in which an adversarial term is added to the loss function during training.Â
This dramatically improves the error rate of the same maxout network to 17.4% on AEs generated by the fast gradient sign method. The linear interpretation of adversarial examples suggests an approach to adversarial training which improves a model’s ability to classify AEs, and helps interpret properties of AE classification which the previously proposed nonlinearity and overfitting hypotheses do not explain.Â
Click here for the full summary in PDF form.
Original paper by Ian J. Goodfellow, Jonathan Shlens and Christian Szegedy: https://arxiv.org/abs/1412.6572