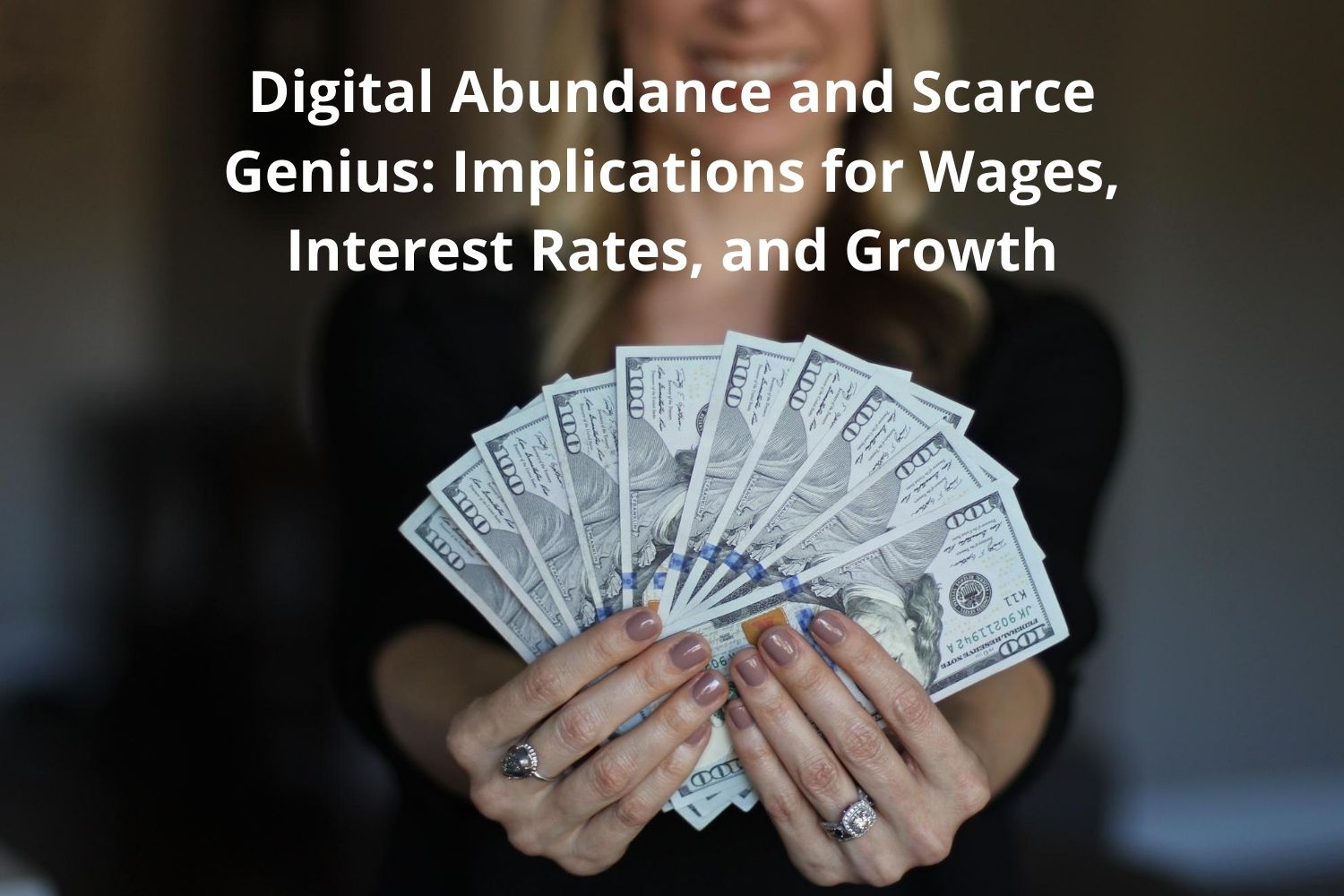
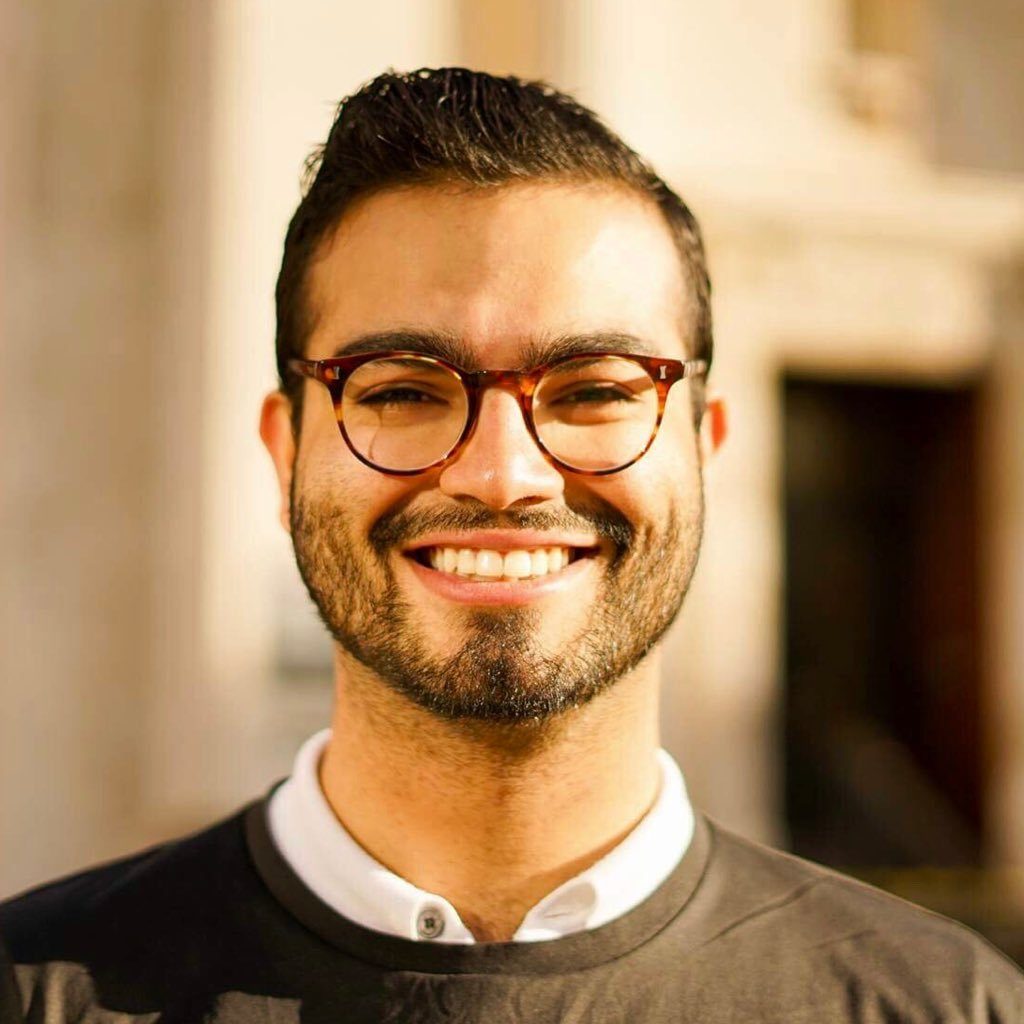
Summary contributed by Ryan Khurana, a graduate of UofT’s Rotman School of Management in the Master’s of Management Analytics program.
*Authors of original paper & link at the bottom
Mini-summary: A paradox that has been confounding economists in recent years is that both labor and capital are experiencing declines in their share of total income. Traditionally these two factors should account for nearly all of the income produced in an economy, with only a small share being taken by profits. The profit share of income has certainly been increasing, but the reasons for this trend and the differences in profits between different industries has been a contentious topic of debate.
Economists Seth Benzell and Erik Brynjolfsson propose that there is a missing component in these discussions, which they argue has been amassing a growing share of income that would have traditionally gone to labor or capital. They call this the “Genius” share of income, the amount of income that goes to superstar individuals or intangibles, such as a firm’s management practices and ability to leverage new technologies. The scarcity of “Genius” has allowed some firms to skyrocket ahead of their competition with the income distribution of their top employees far outpacing those in laggard industries.
The authors argue that increasing the supply of “Genius” through greater access to elite universities and more high-skilled immigration would not only kick start productivity in the wider economy but increase wages for all. The moral implications of this work are quite severe and the model raises lots of critical questions for researchers grappling with the social impacts of AI.
Full summary:
Traditionally the dynamics of capitalist economies are thought to be a clash between labor and capital. In competitive markets with low barriers to access to capital and a skilled labor force that is able to access opportunities, we would expect to find a good equilibrium where total income is growing and labor and capital each have reasonably large shares. When one is growing at the expense of the other, social unrest would be expected. It is surprising then that both labor and capital have had declining shares of income in recent years. Real interest rates are low, investment is low, and real incomes have barely increased in decades. The causes of this paradox are an active debate among economists, and the stakes for a good understanding couldn’t be higher given the current political climate.
Economists Seth Benzell and Erik Brynjolfsson in Digital Abundance and Scarce Genius aim to resolve this paradox by pointing towards the changing nature of the economy due to digitization. The digital world has removed barriers to work and increased access to capital in a way that was not possible in previous eras. The speed at which software scales and the gargantuan revenues digital giants command does not take advantage of traditional labor or traditional capital in the way economists expect. The returns to these superstar firms in the digital economy, the authors argue, result from the scarcity of “Genius”, a factor to which they show a sizeable chunk of total income has appreciated. “Genius” is not some innate superiority among a certain class of people, but rather the returns to a certain type of talent and intangible asset that the digital economy makes more valuable. One can be agnostic to the origins of genius, but the “10x programmers” that are mythical among the tech community do indicate an acknowledgment of the excess returns to a certain type of talent. The authors argue that genius is not just the raw talent, but the configuration of this in certain companies that can scale that talent. Organizational capital, such as being “ML-first” or knowing how to manage technical teams, is an aspect of genius that is rare outside of the tech giants. Virtual real estate, such as intellectual property or platform dominance due to network effects, is another aspect of genius that constricts its supply to a few firms.
The surprising findings of the paper are that in an economy where fewer firms are capturing scarce genius, which in turn commands a greater share of income, traditional means of boosting productivity would prove counterproductive. Upskilling low and medium-wage employees, should the models turn out to be correct, would decrease wages as more workers would be qualified to fill roles that contribute to a declining share of total productivity. Counter-intuitively, targeting productivity improvements at the top would increase wages at the bottom more rapidly. The logic is as follows: if the bottleneck on genius is lifted by increased top-end productivity, then the market for genius is more competitive and the cost of genius decreases, but given the necessity of genius to digital success, productivity would increase much more, returning a greater share to lower and medium-income workers. Efforts such as increasing high-skilled immigration, increasing access to top schools, loosening IP laws, and improved education that provides interdisciplinary management skills and creative thinking would all serve to boost genius.
This is where the authors leave their discussion and the point where AI ethicists need to pick up the mantle. The returns to genius would likely accelerate in an AI-driven world where only a few companies have yet to realize value from AI investments. While traditional companies seek out unicorn data scientists to do everything, and rarely realize value, “ML-first” companies are able to capitalize on specialized AI talent managed correctly. Democratizing AI needs to reckon with the scarcity of genius.
The solutions to this problem, however, require grappling with the institutional environment that allowed genius to become scarce. The current class of top earners whose salaries result from membership in the genius class have strong incentives to restrict access. Ivy league schools are selective not due to physical constraints, but in order to restrict the supply of people with their credentials, allowing them to command more income. Upskilling the middle class is far more politically popular than creating more elites because it presents less of a challenge to the current elites. Navigating this political minefield was briefly touched upon by the authors but presents an interesting area of exploration for AI ethicists. The question “who is benefitting from new technologies?” is at the heart of AI ethics research, and one that observing the scarcity of genius may help to answer.
Original paper by Seth Benzell and Erik Brynjolfsson: https://papers.ssrn.com/sol3/papers.cfm?abstract_id=3341245